Remedying the Neyman–Scott phenomenon in model discrimination
JOURNAL OF STATISTICAL COMPUTATION AND SIMULATION(2011)
摘要
The objective of this paper is to investigate through simulation the possible presence of the incidental parameters problem when performing frequentist model discrimination with stratified data. In this context, model discrimination amounts to considering a structural parameter taking values in a finite space, with k points, k epsilon 2. This setting seems to have not yet been considered in the literature about the Neyman-Scott phenomenon. Here we provide Monte Carlo evidence of the severity of the incidental parameters problem also in the model discrimination setting and propose a remedy for a special class of models. In particular, we focus on models that are scale families in each stratum. We consider traditional model selection procedures, such as the Akaike and Takeuchi information criteria, together with the best frequentist selection procedure based on maximization of the marginal likelihood induced by the maximal invariant, or of its Laplace approximation. Results of two Monte Carlo experiments indicate that when the sample size in each stratum is fixed and the number of strata increases, correct selection probabilities for traditional model selection criteria may approach zero, unlike what happens for model discrimination based on exact or approximate marginal likelihoods. Finally, two examples with real data sets are given.
更多查看译文
关键词
Akaike's information criterion,group invariance,incidental parameters problem,Laplace expansion,marginal likelihood,nuisance parameter,panel data,profile likelihood,stratified data,Takeuchi's information criterion
AI 理解论文
溯源树
样例
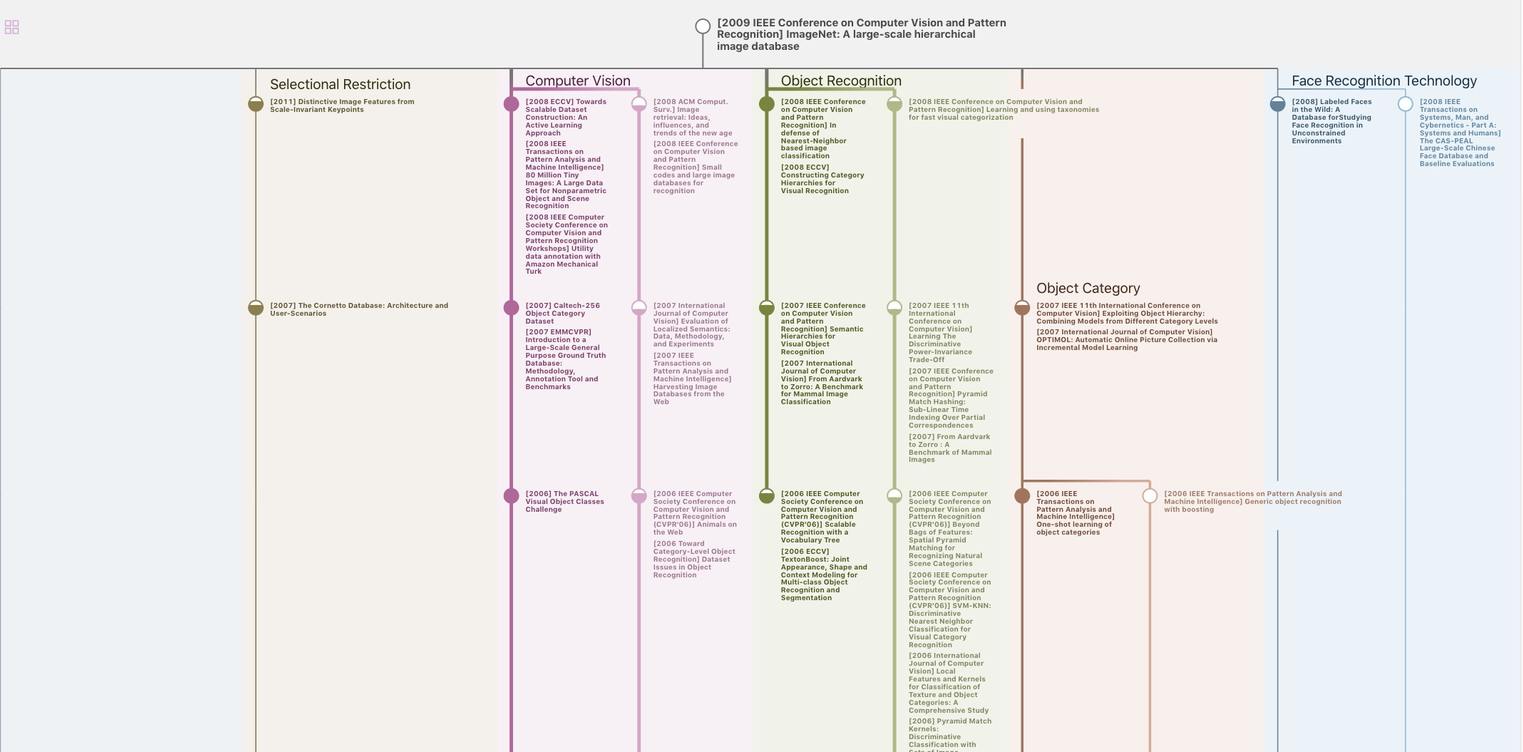
生成溯源树,研究论文发展脉络
Chat Paper
正在生成论文摘要