A screening approach for non-parametric global sensitivity analysis
JOURNAL OF STATISTICAL COMPUTATION AND SIMULATION(2016)
摘要
Global sensitivity analysis (GSA) can help practitioners focusing on the inputs whose uncertainties have an impact on the model output, which allows reducing the complexity of the model. Screening, as the qualitative method of GSA, is to identify and exclude non- or less-influential input variables in high-dimensional models. However, for non-parametric problems, there remains the challenging problem of finding an efficient screening procedure, as one needs to properly handle the non-parametric high-order interactions among input variables and keep the size of the screening experiment economically feasible. In this study, we design a novel screening approach based on analysis of variance decomposition of the model. This approach combines the virtues of run-size economy and model independence. The core idea is to choose a low-level complete orthogonal array to derive the sensitivity estimates for all input factors and their interactions with low cost, and then develop a statistical process to screen out the non-influential ones without assuming the effect-sparsity of the model. Simulation studies show that the proposed approach performs well in various settings.
更多查看译文
关键词
screening,non-parametric,global sensitivity analysis,orthogonal array
AI 理解论文
溯源树
样例
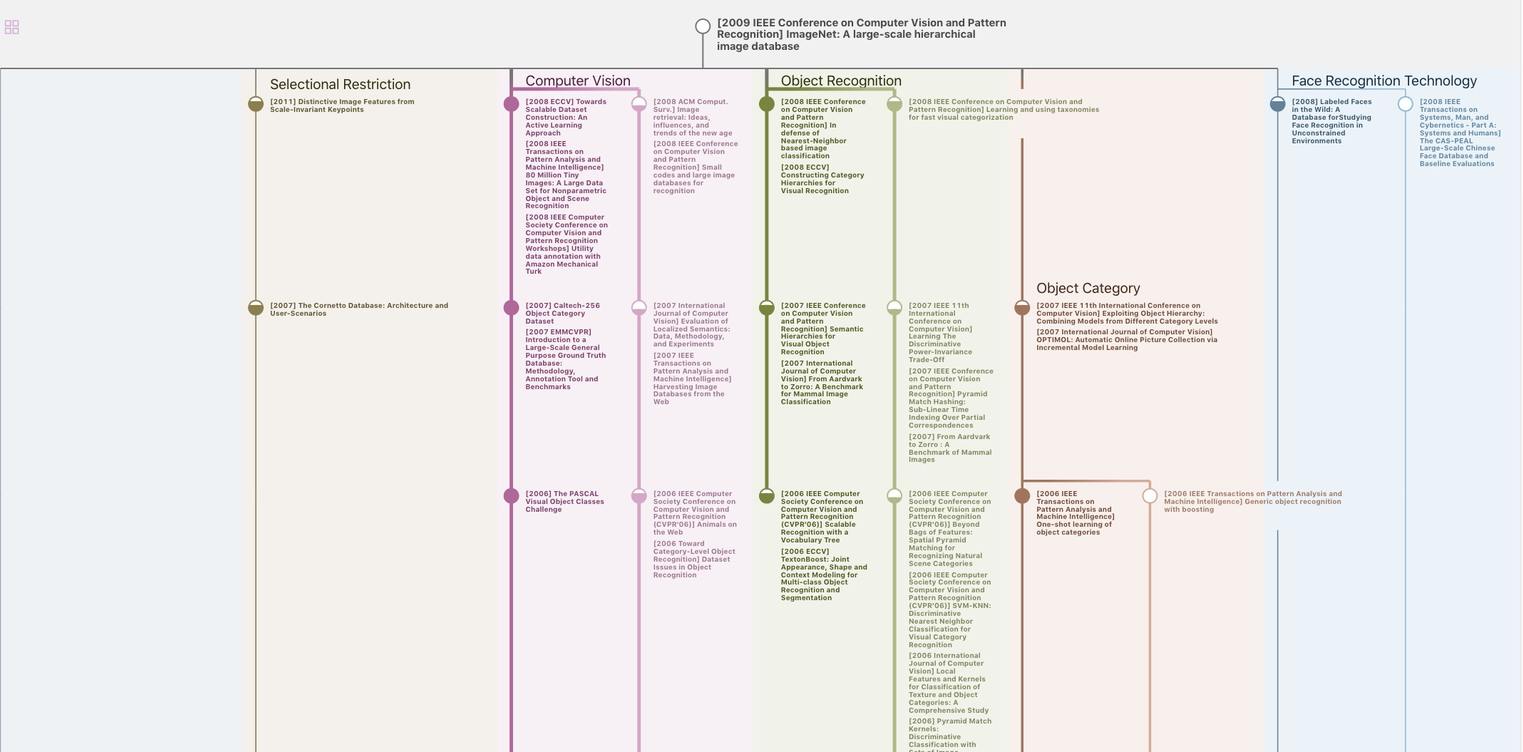
生成溯源树,研究论文发展脉络
Chat Paper
正在生成论文摘要