The importance of spatial models for estimating the strength of density dependence
ECOLOGY(2015)
摘要
Identifying the existence and magnitude of density dependence is one of the oldest concerns in ecology. Ecologists have aimed to estimate density dependence in population and community data by fitting a simple autoregressive (Gompertz) model for density dependence to time series of abundance for an entire population. However, it is increasingly recognized that spatial heterogeneity in population densities has implications for population and community dynamics. We therefore adapt the Gompertz model to approximate local densities over continuous space instead of population-wide abundance, and allow productivity to vary spatially using Gaussian random fields. We then show that the conventional (nonspatial) Gompertz model can result in biased estimates of density dependence (e.g., identifying oscillatory dynamics when not present) if densities vary spatially. By contrast, the spatial Gompertz model provides accurate and precise estimates of density dependence for a variety of simulation scenarios and data availabilities. These results are corroborated when comparing spatial and nonspatial models for data from 10 years and; 100 sampling stations for three long-lived rockfishes (Sebastes spp.) off the California, USA coast. In this case, the nonspatial model estimates implausible oscillatory dynamics on an annual time scale, while the spatial model estimates strong autocorrelation and is supported by model selection tools. We conclude by discussing the importance of improved data archiving techniques, so that spatial models can be used to reexamine classic questions regarding the existence and magnitude of density dependence in wild populations.
更多查看译文
关键词
autoregressive model,density dependence,Gaussian random field,Gompertz model,integrated nested Laplace approximation (INLA),Pacific rockfish (Sebastes spp.),spatial modeling,spatial variation,template model builder (TMB)
AI 理解论文
溯源树
样例
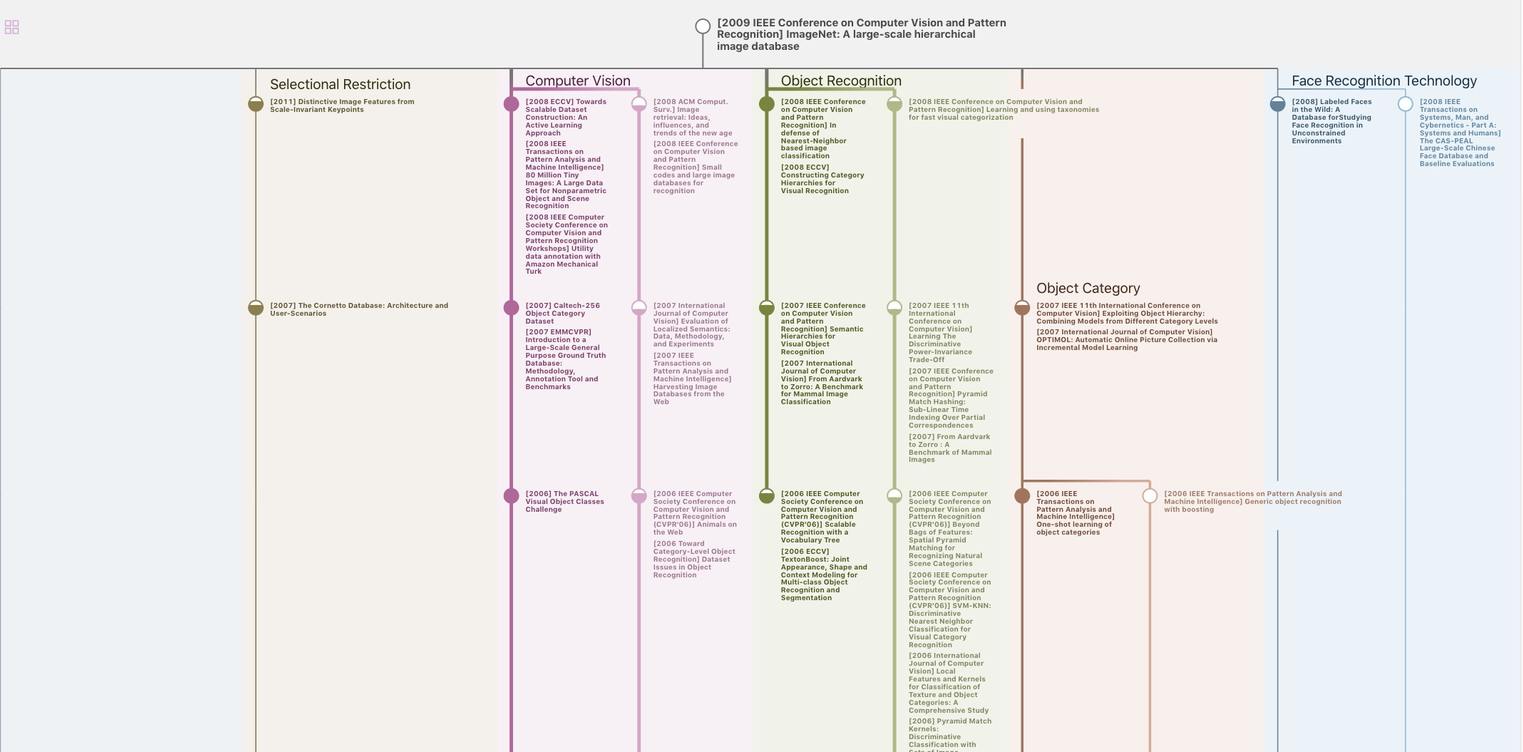
生成溯源树,研究论文发展脉络
Chat Paper
正在生成论文摘要