EPILEPTIC EEG CLASSIFICATION USING NONLINEAR PARAMETERS ON DIFFERENT FREQUENCY BANDS
Journal of Mechanics in Medicine and Biology(2015)
摘要
Epilepsy is a chronic neurological disorder with considerable incidence and affects the population everywhere in the world. It occurs due to recurrent unprovoked seizures which can be noninvasively diagnosed using electroencephalograms (EEGs) which are the neuronal electrical activity recorded on the scalp. The EEG signal is highly random, nonlinear, nonstationary and non-Gaussian in nature. The nonlinear features characterize the EEG more accurately than linear models. EEG comprsises of different activities like delta, theta, lower alpha, upper alpha, lower beta, upper beta and lower gamma which are correlated to the brain anatomy and its function. In the current study, the nonlinear features such as Hurst exponent (HE), Higuchi fractal dimension (HFD), largest Lyapunov exponent (LLE) and sample entropy (SE) are extracted on these individual activities to provide improved discrimination. The ranked features are classified using support vector machine (SVM) with different kernel functions, decision tree (DT) and k-nearest neighbor (KNN) to select the best classifier. It is observed that SVM with radial basis function (RBF) kernel provides highest accuracy of 98%, sensitivity and specificity of 99.5% and 100%, respectively using five features. The developed methodology is ready for epilepsy screening and can be deployed in many programmes.
更多查看译文
关键词
Epilepsy,electroencephalogram (EEG),hurst exponent,fractal dimension,largest Lyapunov exponent,sample entropy,classifier
AI 理解论文
溯源树
样例
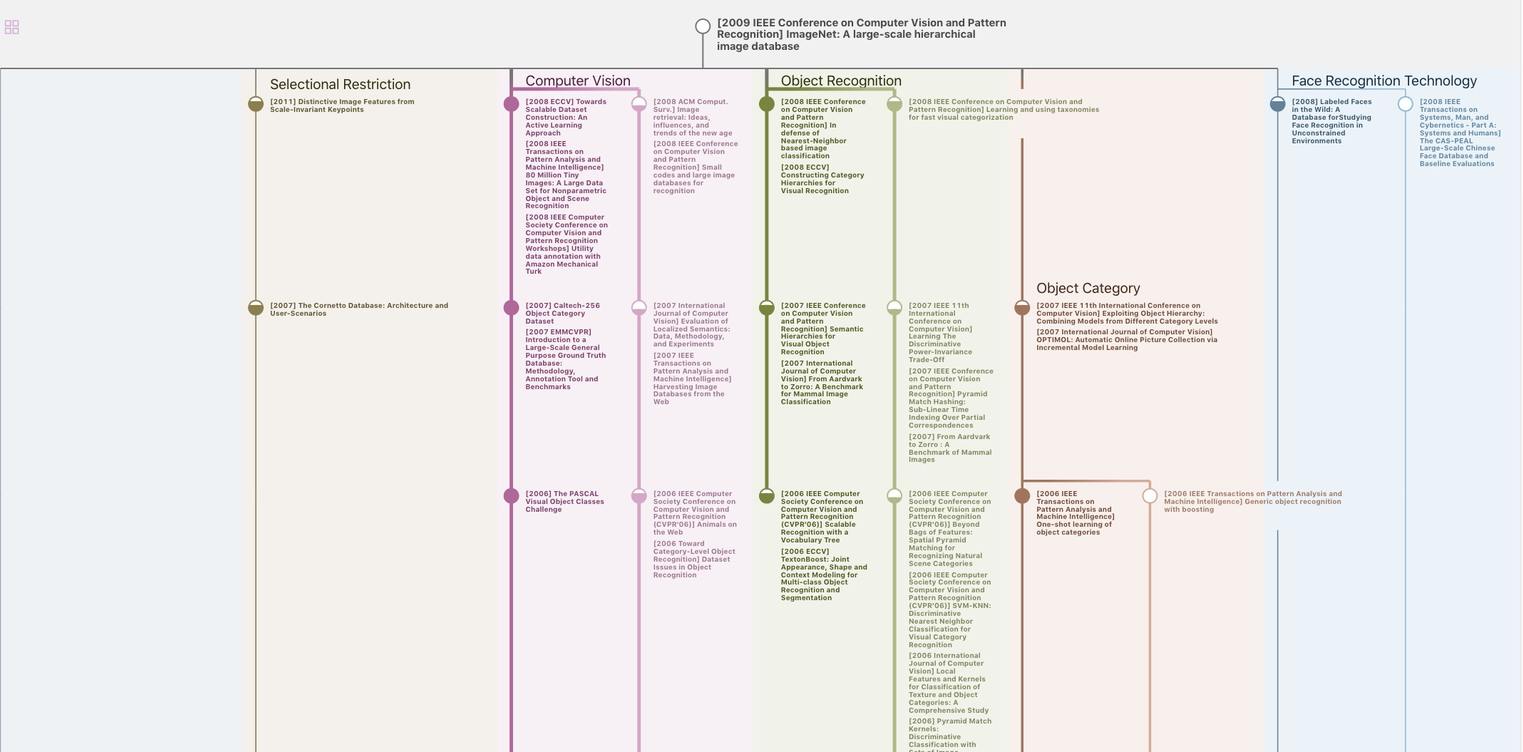
生成溯源树,研究论文发展脉络
Chat Paper
正在生成论文摘要