AUTOMATED CHARACTERIZATION AND DETECTION OF DIABETIC RETINOPATHY USING TEXTURE MEASURES
JOURNAL OF MECHANICS IN MEDICINE AND BIOLOGY(2015)
摘要
The chronic and uncontrolled diabetes mellitus (DM) damages the retinal blood vessels leading to diabetic retinopathy (DR). The advanced stage of DR leads to loss of vision and subsequently blindness. The morphological changes during the progression of DR can be diagnosed using digital fundus images. The pathological changes in the retina influence the variations in pixel patterns which can be quantified using texture measures. In this paper, we have explored different texture measures namely statistical moments, gray level co-occurrence matrix (GLCM), gray level run length matrix (GLRLM), local binary pattern (LBP), laws mask energy (LME), fractal dimension (FD), fourier spectrum (FS) and Gabor wavelet to characterize and classify the normal and DR classes. We have tabulated 109 texture parameters for the normal and DR classes. Further, these features were subjected to empirical receiver operating characteristic (ROC) based ranking to select optimal feature set. The ranked nested features were fed to the support vector machine (SVM) classifier with different kernel functions to evaluate the highest performance measure using the least number of features to discriminate normal and DR classes. Our proposed system was evaluated using two different databases Kasturba Medical College Hospital (KMCH) and Tan Tock Seng Hospital (TTSH), each with 340 images (170 normal and 170 DR). We have also formulated an integrated index called as diabetic retinopathy risk index (DRRI) using selected texture features to discriminate normal and DR classes using single number. The proposed frame work can be used to help the clinicians and also for mass DR screening programs.
更多查看译文
关键词
Retina,diabetic retinopathy,texture,local binary pattern,laws mask energy,gabor wavelet,Empirical ROC,support vector machine
AI 理解论文
溯源树
样例
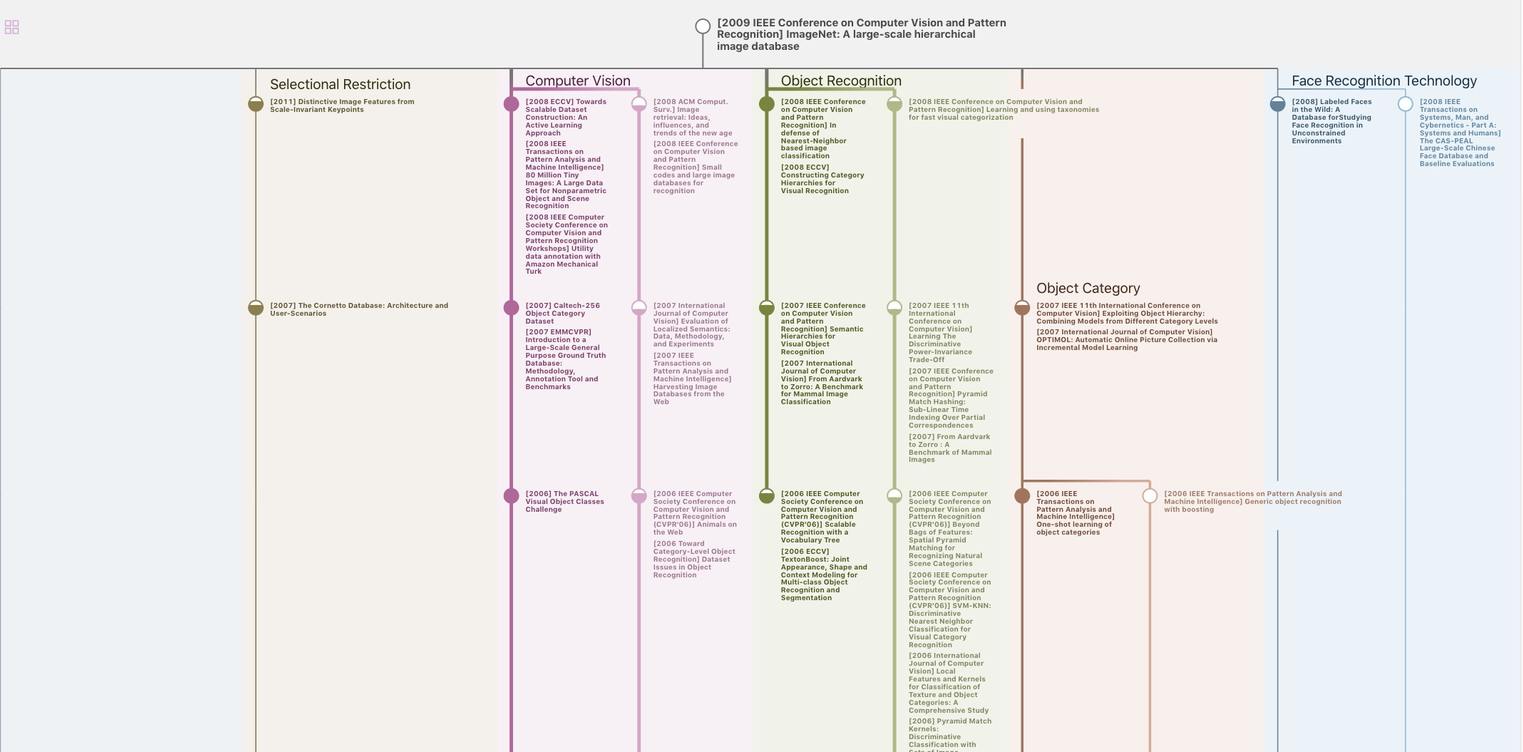
生成溯源树,研究论文发展脉络
Chat Paper
正在生成论文摘要