Iterative Deep Graph Learning and Embedding for Industrial Fault Diagnosis With Limited Labeled Data
IEEE TRANSACTIONS ON INSTRUMENTATION AND MEASUREMENT(2024)
摘要
The labeled monitoring data collected from industrial processes is extremely limited in real production, which imposes a big challenge on traditional supervised fault diagnosis methods. To alleviate this problem, we propose a novel iterative deep graph learning and embedding (IDGLE) framework for semisupervised fault diagnosis with limited labeled data. First, a contrastive-enhanced graph learning network (CGLN) is presented to explicitly model the relationships among samples into an affinity graph. The contrastive mechanism is innovatively applied to improve the graph quality by amplifying the intraclass similarity and interclass difference. Then, a structure-guided pseudo-label generator (SPLG) is developed for label inference and scoring. It annotates unlabeled nodes through a label-augmented graph neural network (GNN) and evaluates the predictions using a specially designed structure-guided confidence metric. The incorporation of structural information can provide additional views to improve the accuracy of classification and evaluation. Finally, a progressive iterative learning strategy is proposed to gradually augment the labeled data with more reliable pseudo labels according to the training pace. By using both the originally available labeled data and the generated pseudo-labeled data, IDGLE can iteratively update both the graph structure and node representations to continuously improve fault diagnosis performance. Extensive experimental results on the Tennessee Eastman (TE) process and a real-world air separation (AS) process show that the proposed method can achieve excellent fault diagnosis performance with limited labeled data. In the case where only 10% of the data is labeled, the classification accuracy of IDGLE in these two processes is 0.9391 and 0.9521, respectively, outperforming all comparison methods. Moreover, it takes IDGLE less than 2.4E-05 s to perform online diagnosis on one sample, which is computationally efficient for practice.
更多查看译文
关键词
Contrastive mechanism,graph neural networks (GNNs),industrial fault diagnosis,pseudo labeling,semisupervised learning (SSL)
AI 理解论文
溯源树
样例
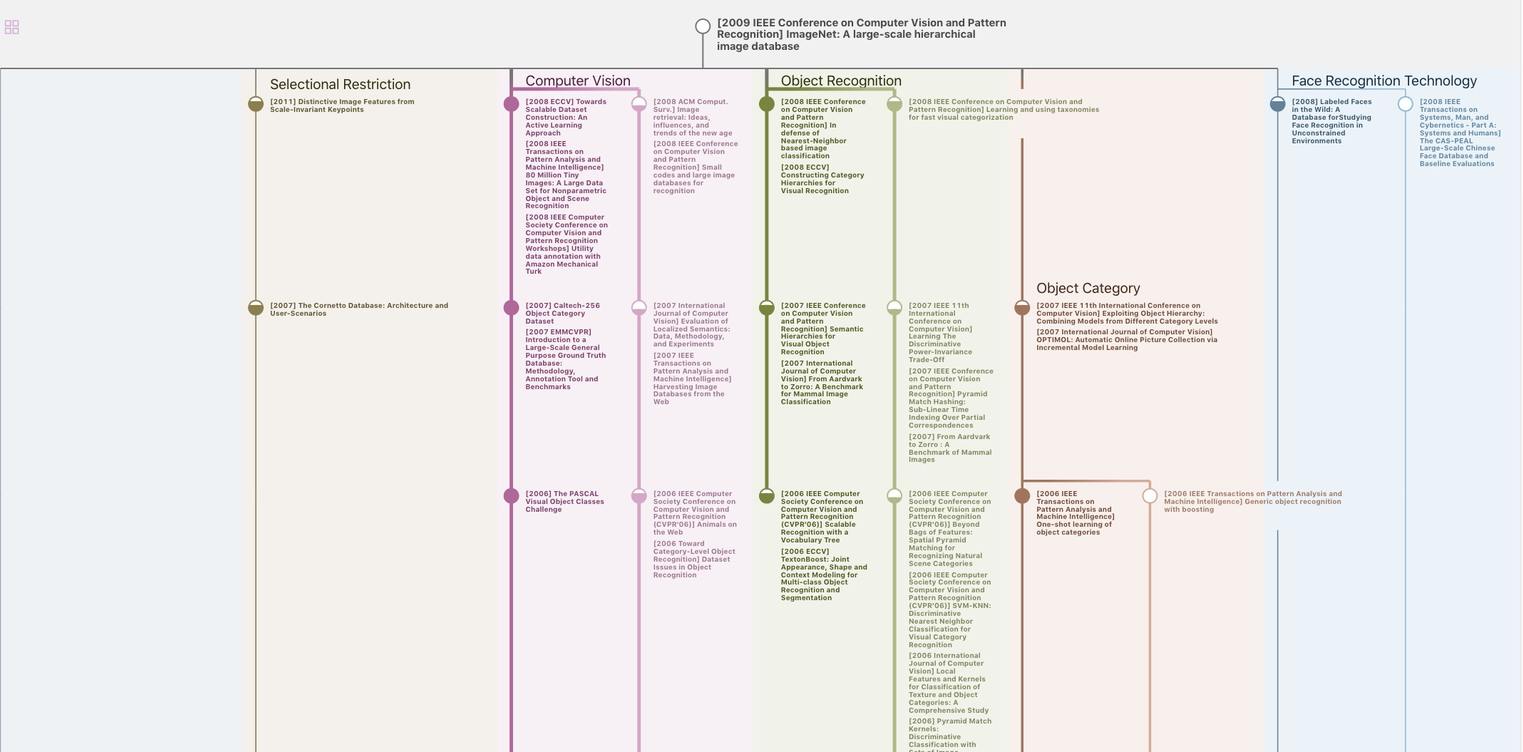
生成溯源树,研究论文发展脉络
Chat Paper
正在生成论文摘要