A deep bidirectional LSTM approach for video-realistic talking head
Multimedia Tools Appl.(2015)
摘要
This paper proposes a deep bidirectional long short-term memory approach in modeling the long contextual, nonlinear mapping between audio and visual streams for video-realistic talking head. In training stage, an audio-visual stereo database is firstly recorded as a subject talking to a camera. The audio streams are converted into acoustic feature, i.e. Mel-Frequency Cepstrum Coefficients (MFCCs), and their textual labels are also extracted. The visual streams, in particular, the lower face region, are compactly represented by active appearance model (AAM) parameters by which the shape and texture variations can be jointly modeled. Given pairs of the audio and visual parameter sequence, a DBLSTM model is trained to learn the sequence mapping from audio to visual space. For any unseen speech audio, whether it is original recorded or synthesized by text-to-speech (TTS), the trained DBLSTM model can predict a convincing AAM parameter trajectory for the lower face animation. To further improve the realism of the proposed talking head, the trajectory tiling method is adopted to use the DBLSTM predicted AAM trajectory as a guide to select a smooth real sample image sequence from the recorded database. We then stitch the selected lower face image sequence back to a background face video of the same subject, resulting in a video-realistic talking head. Experimental results show that the proposed DBLSTM approach outperforms the existing HMM-based approach in both objective and subjective evaluations.
更多查看译文
关键词
Talking head, Visual speech synthesis, Recurrent neural network, Long short-term memory, Active appearance model
AI 理解论文
溯源树
样例
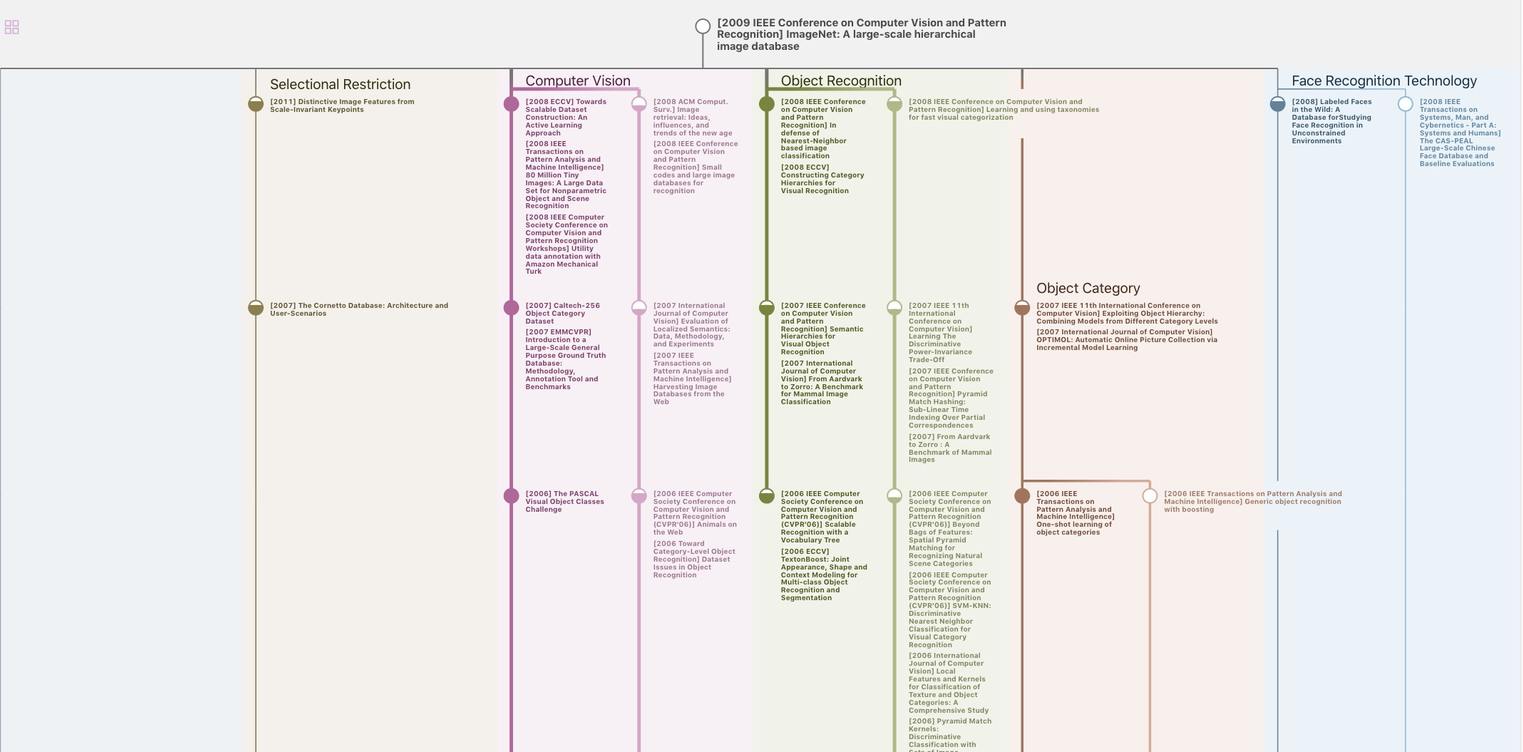
生成溯源树,研究论文发展脉络
Chat Paper
正在生成论文摘要