Hyperspectral image classification based on spatial and spectral features and sparse representation
Applied Geophysics(2015)
摘要
To minimize the low classification accuracy and low utilization of spatial information in traditional hyperspectral image classification methods, we propose a new hyperspectral image classification method, which is based on the Gabor spatial texture features and nonparametric weighted spectral features, and the sparse representation classification method (Gabor-NWSF and SRC), abbreviated GNWSF-SRC. The proposed (GNWSF-SRC) method first combines the Gabor spatial features and nonparametric weighted spectral features to describe the hyperspectral image, and then applies the sparse representation method. Finally, the classification is obtained by analyzing the reconstruction error. We use the proposed method to process two typical hyperspectral data sets with different percentages of training samples. Theoretical analysis and simulation demonstrate that the proposed method improves the classification accuracy and Kappa coefficient compared with traditional classification methods and achieves better classification performance.
更多查看译文
关键词
Hyperspectral,classification,sparse representation,spatial features,spectral features
AI 理解论文
溯源树
样例
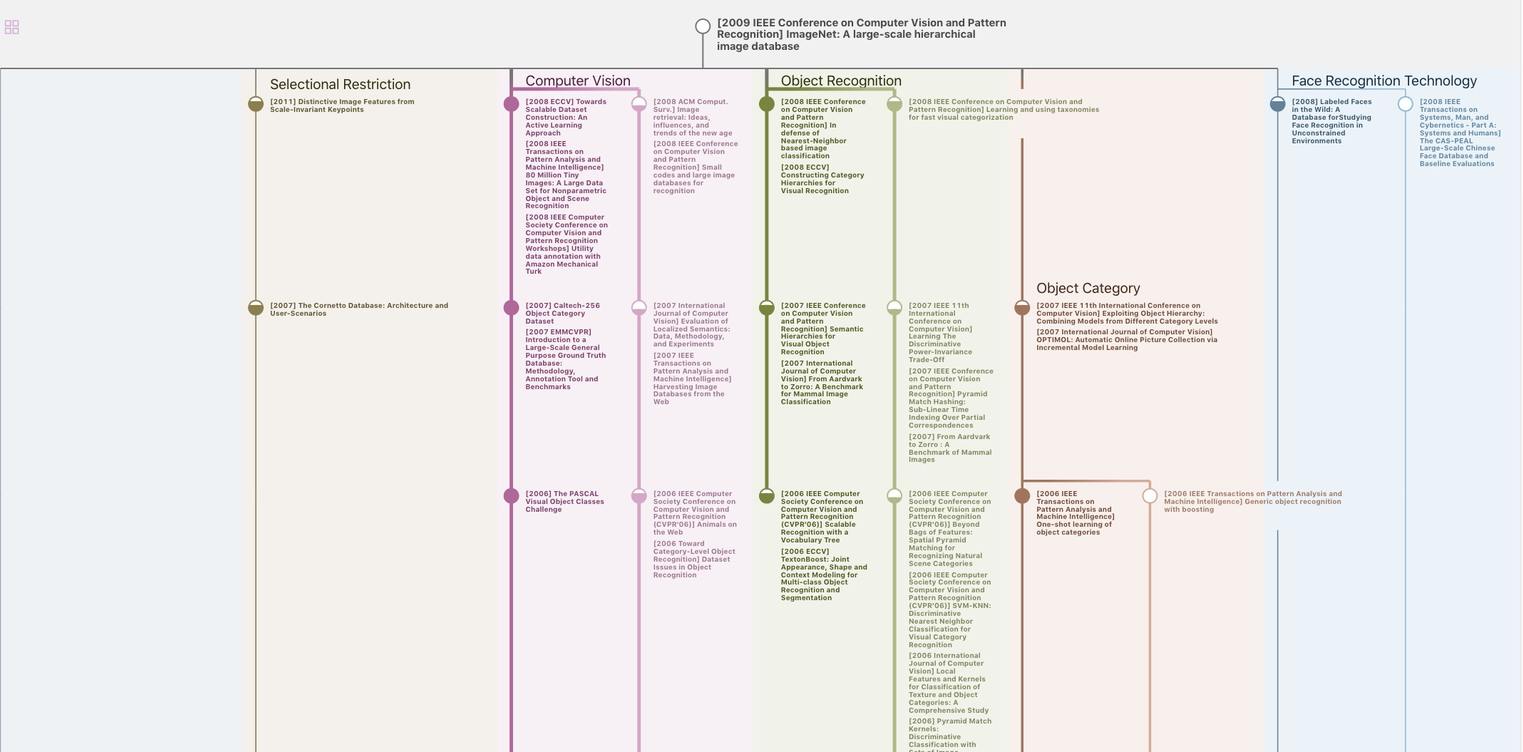
生成溯源树,研究论文发展脉络
Chat Paper
正在生成论文摘要