Learning Hierarchical Task Models from Input Traces
computational intelligence(2016)
摘要
AbstractWe describe HTN-MAKER, an algorithm for learning hierarchical planning knowledge in the form of task-reduction methods for hierarchical task networks HTNs. HTN-MAKERtakes as input a set of planning states from a classical planning domain and plans that are applicable to those states, as well as a set of semantically annotated tasks to be accomplished. The algorithm analyzes this semantic information to determine which portion of the input plans accomplishes a particular task and constructs task-reduction methods based on those analyses.
更多查看译文
关键词
HTN planning,automated planning,machine learning
AI 理解论文
溯源树
样例
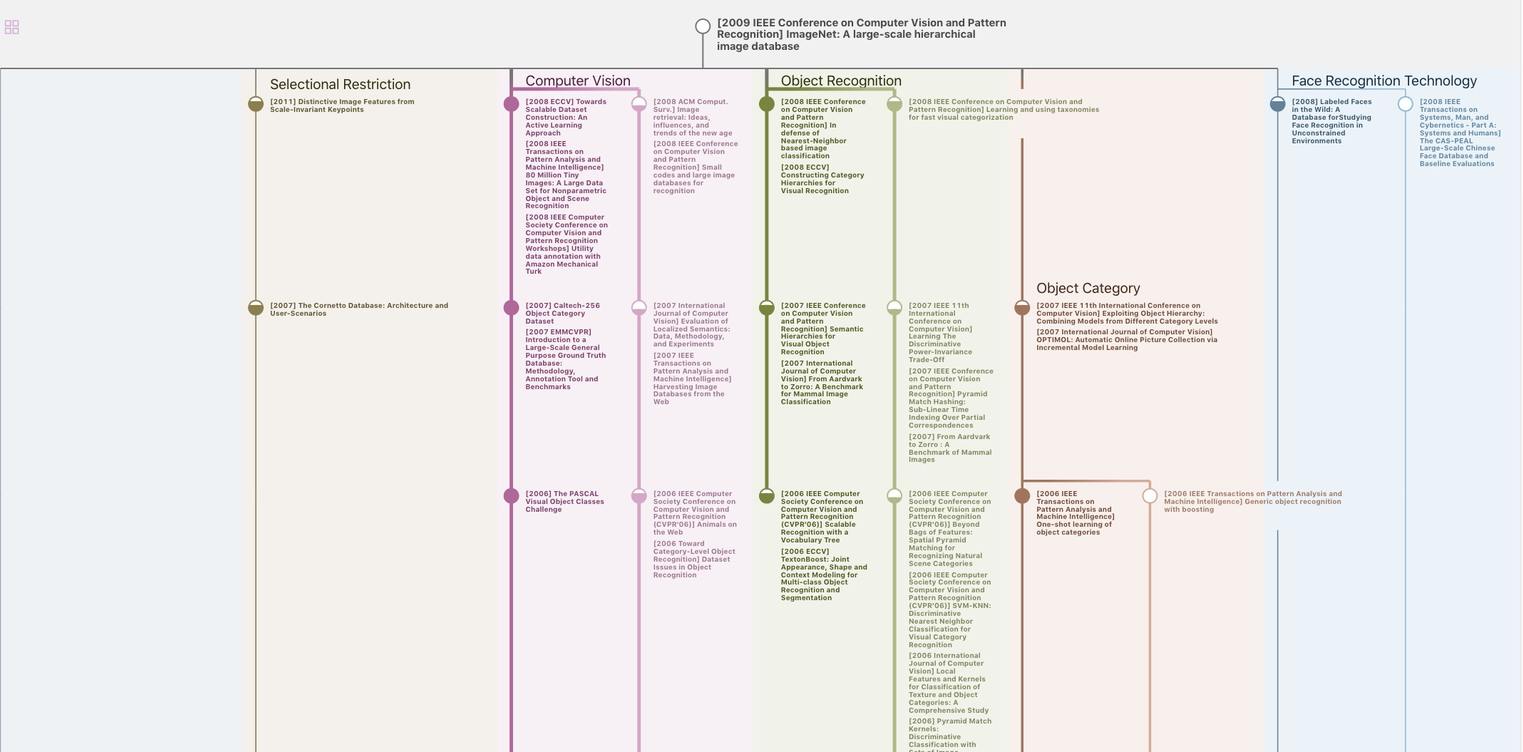
生成溯源树,研究论文发展脉络
Chat Paper
正在生成论文摘要