Joint adaptive loss and l2 /l0 -norm minimization for unsupervised feature selection
2015 International Joint Conference on Neural Networks (IJCNN)(2015)
摘要
Unsupervised feature selection is a useful tool for reducing the complexity and improving the generalization performance of data mining tasks. In this paper, we propose an Adaptive Unsupervised Feature Selection (AUFS) algorithm with explicit l
2
/l
0
-norm minimization. We use a joint adaptive loss for data fitting and a l
2
/l
0
minimization for feature selection. We solve the optimization problem with an efficient iterative algorithm and prove that all the expected properties of unsupervised feature selection can be preserved. We also show that the computational complexity and memory use is only linear to the number of instances and square to the number of clusters. Experiments show that our algorithm outperforms the state-of-the-arts on seven different benchmark data sets.
更多查看译文
关键词
joint adaptive loss,l2/l0-norm minimization,data mining,adaptive unsupervised feature selection,AUFS algorithm,data fitting,optimization problem,iterative algorithm,computational complexity,memory use,clusters
AI 理解论文
溯源树
样例
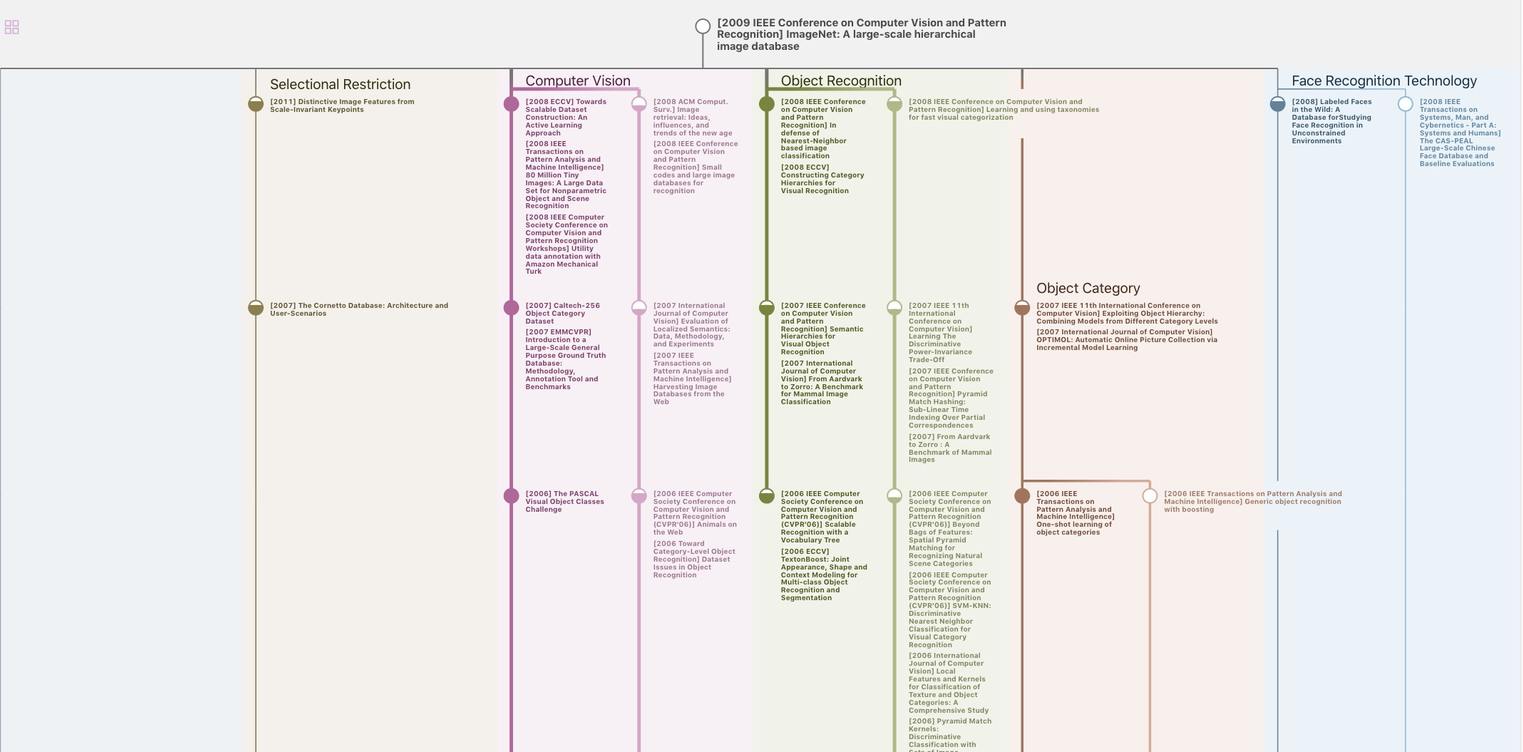
生成溯源树,研究论文发展脉络
Chat Paper
正在生成论文摘要