A Bayesian Approach For Classification Of Buried Objects Using Non-Parametric Prior Model
2006 IEEE INTERNATIONAL GEOSCIENCE AND REMOTE SENSING SYMPOSIUM, VOLS 1-8(2006)
摘要
We used kernel density estimation to build a-priori probability distributions on the vector of features used to characterize unexploded ordnance from electromagnetic induction sensor data. This priori information is then used in a Bayesian framework to develop a new suite of estimation and classification algorithms. Based on this prior information several classification algorithms are developed in feature and signal space. Results using real field data show more robust estimation and significant improvement in classification performance for signal space classifiers comparing to conventional Gaussian approximation to the density of the features.
更多查看译文
关键词
kernel,electromagnetic induction,kernel density estimate,frequency,electromagnetic modeling,classification algorithms,robust estimator,probability distribution,bayesian methods,bayesian approach,lifting equipment
AI 理解论文
溯源树
样例
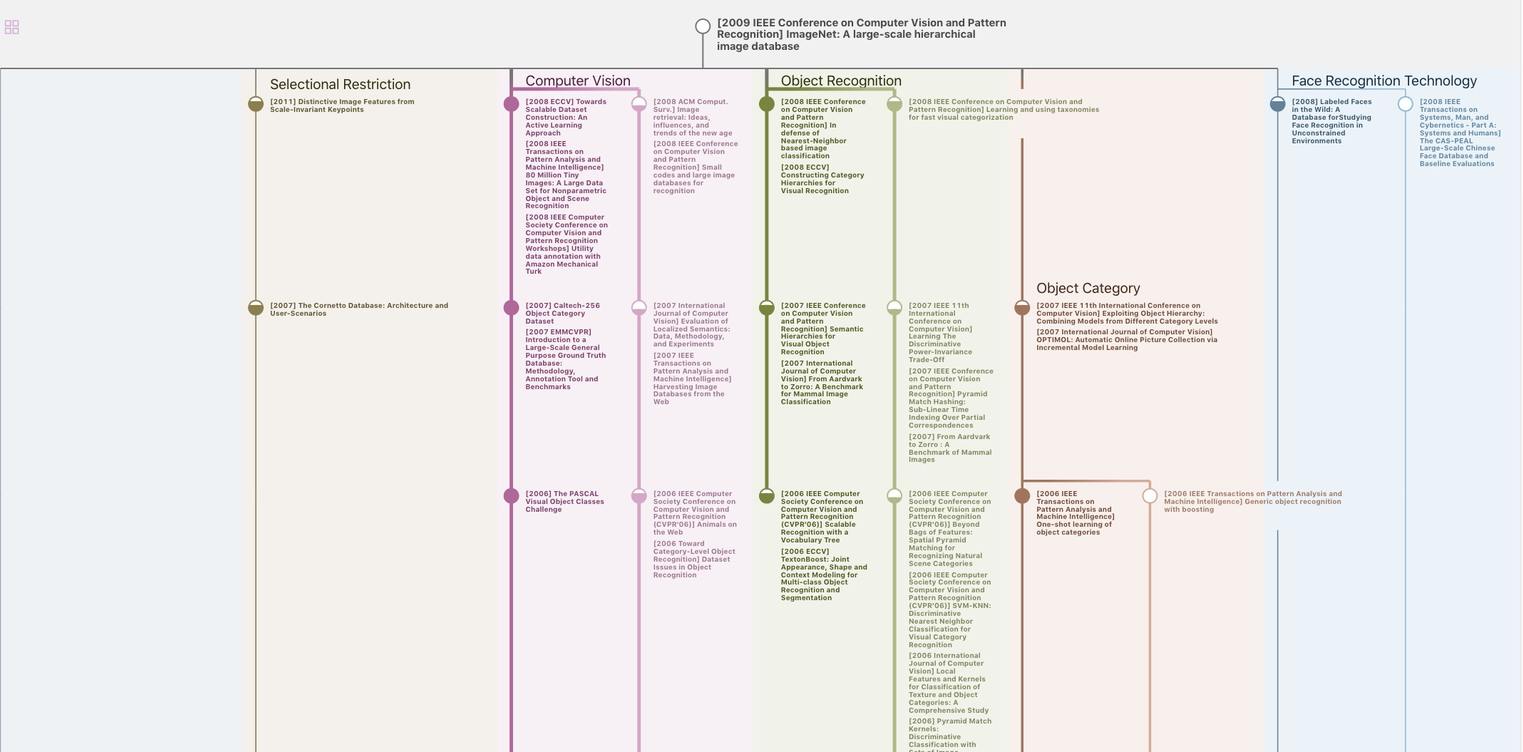
生成溯源树,研究论文发展脉络
Chat Paper
正在生成论文摘要