Support Vector Machines For Ranking Learning: The Full And The Truncated Fixed Margin Strategies
PROCEEDINGS OF 2007 INTERNATIONAL CONFERENCE ON MACHINE LEARNING AND CYBERNETICS, VOLS 1-7(2007)
摘要
Two known SVM-based approaches to ranking learning (ordinal regression estimation, supervised pattern recognition with ordered classes) are scrutinized as different generalizations of the classical principle of finding the optimal discriminant hyperplane in a linear space. Easily verifiable natural conditions are found under which the training result obtained by the computationally much more attractive truncated technique is completely equivalent to the hypothetical strict solution. The numerical procedures are essentially simplified for both techniques.
更多查看译文
关键词
ordinal regression, ranking learning, large margin learning, support vector machine, computational complexity
AI 理解论文
溯源树
样例
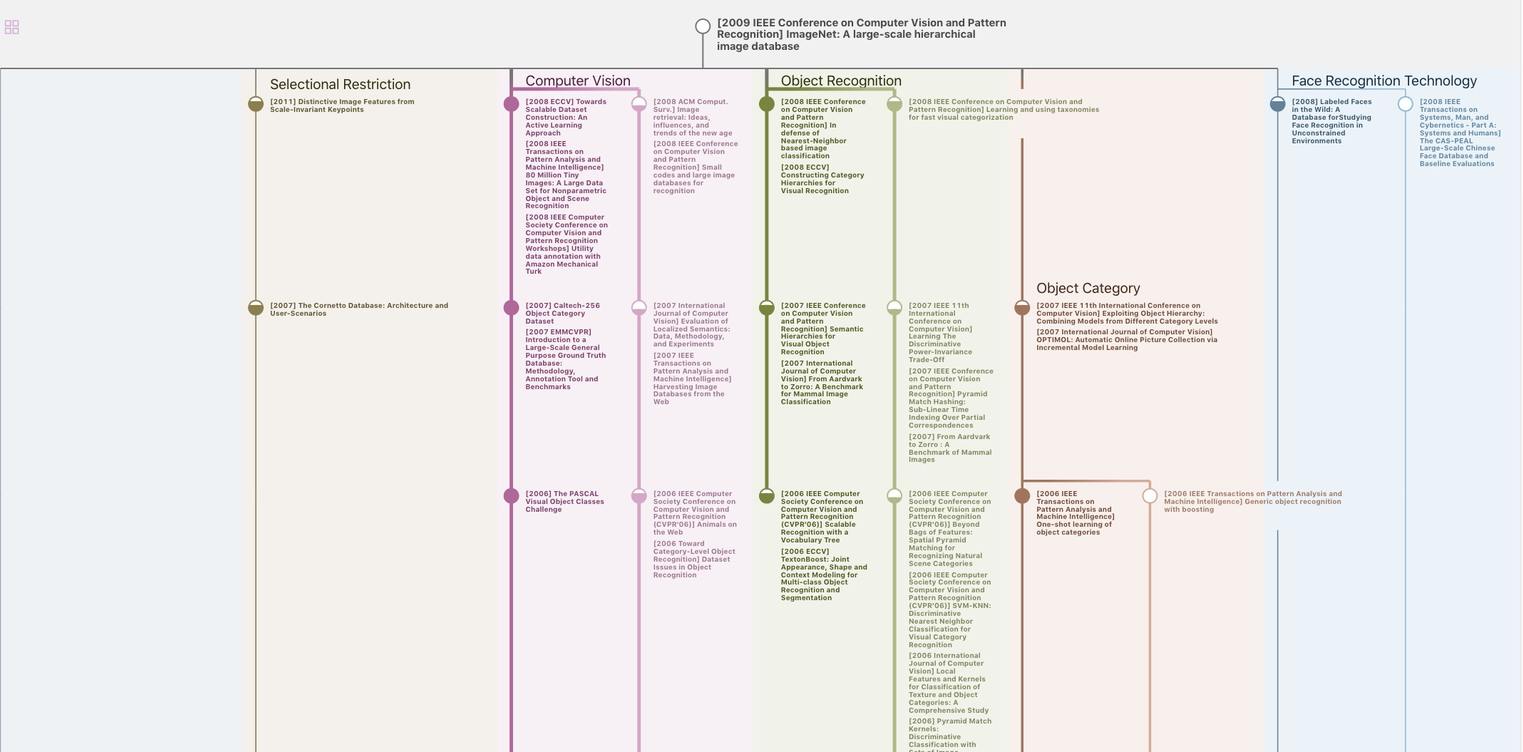
生成溯源树,研究论文发展脉络
Chat Paper
正在生成论文摘要