A Transfer Learning Approach for Applying Matrix Factorization to Small ITS Datasets.
EDM(2015)
摘要
Machine Learning methods for Performance Prediction in Intelligent Tutoring Systems (ITS) have proven their efficacy; specific methods, e.g. Matrix Factorization (MF), however suffer from the lack of available information about new tasks or new students. In this paper we show how this problem could be solved by applying Transfer Learning (TL), i.e. combining similar but not equal datasets to train Machine Learning models. In our case we obtain promising results by combining data collected of German fractions’ tasks (517 interactions, 88 students, 20 tasks) with their nonexact translation of a previously American US version (140 interactions, 14 students, 16 tasks). In order to do so we also analyze the performance of MF based predictors on smaller ITS’ samples evaluating their usefulness.
更多查看译文
关键词
matrices,statistics,data collection,performance,fractions,probability,task analysis,prediction,mathematics
AI 理解论文
溯源树
样例
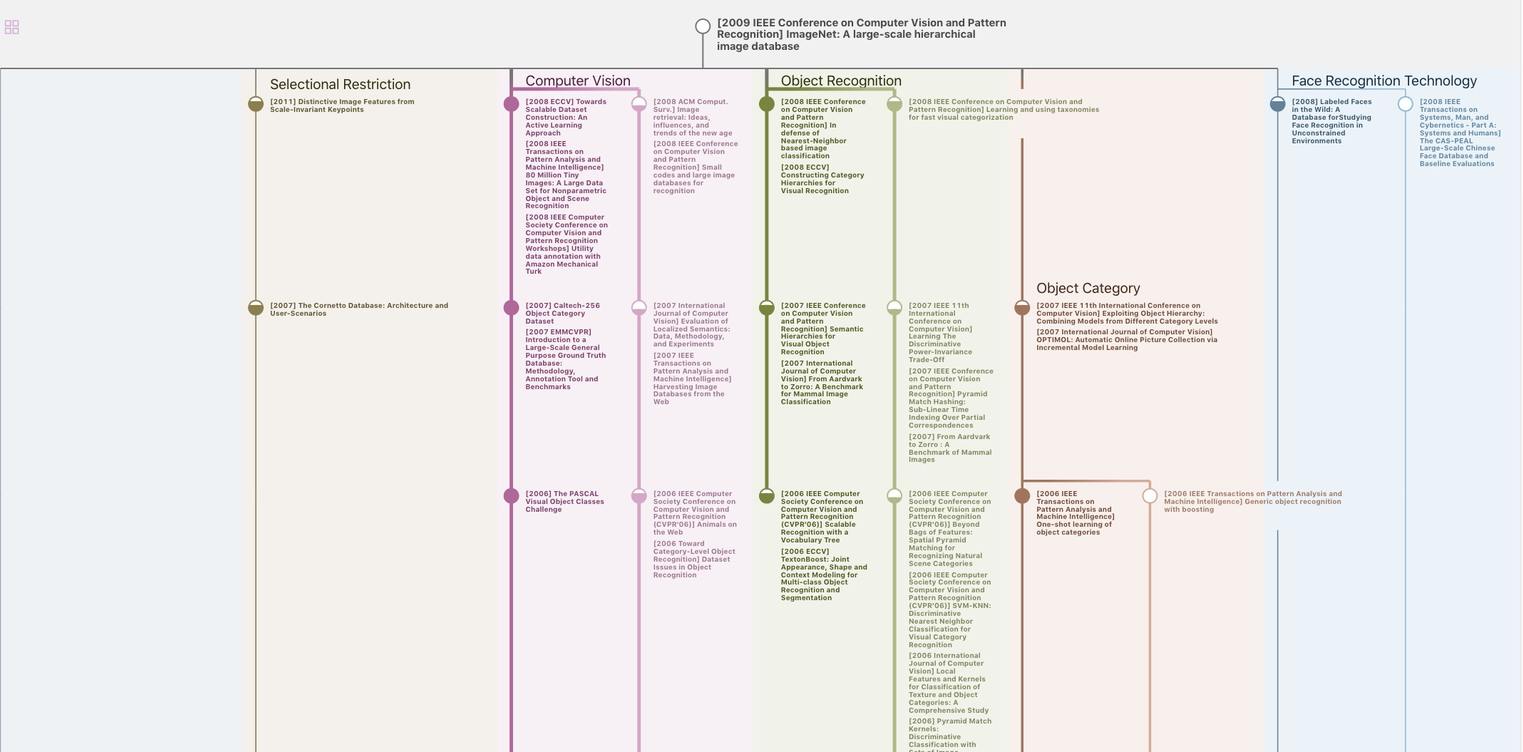
生成溯源树,研究论文发展脉络
Chat Paper
正在生成论文摘要