Reweighted Nuclear Norm Minimization With Application To System Identification
2010 AMERICAN CONTROL CONFERENCE(2010)
摘要
The matrix rank minimization problem consists of finding a matrix of minimum rank that satisfies given convex constraints. It is NP-hard in general and has applications in control, system identification, and machine learning. Reweighted trace minimization has been considered as an iterative heuristic for this problem. In this paper, we analyze the convergence of this iterative heuristic, showing that the difference between successive iterates tends to zero. Then, after reformulating the heuristic as reweighted nuclear norm minimization, we propose an efficient gradient-based implementation that takes advantage of the new formulation and opens the way to solving large-scale problems. We apply this algorithm to the problem of low-order system identification from input-output data. Numerical examples demonstrate that the reweighted nuclear norm minimization makes model order selection easier and results in lower order models compared to nuclear norm minimization without weights.
更多查看译文
关键词
minimization,nickel,sparse matrices,input output,machine learning,system identification,convergence,satisfiability,computational complexity,np hard problem,minimisation,identification,control system
AI 理解论文
溯源树
样例
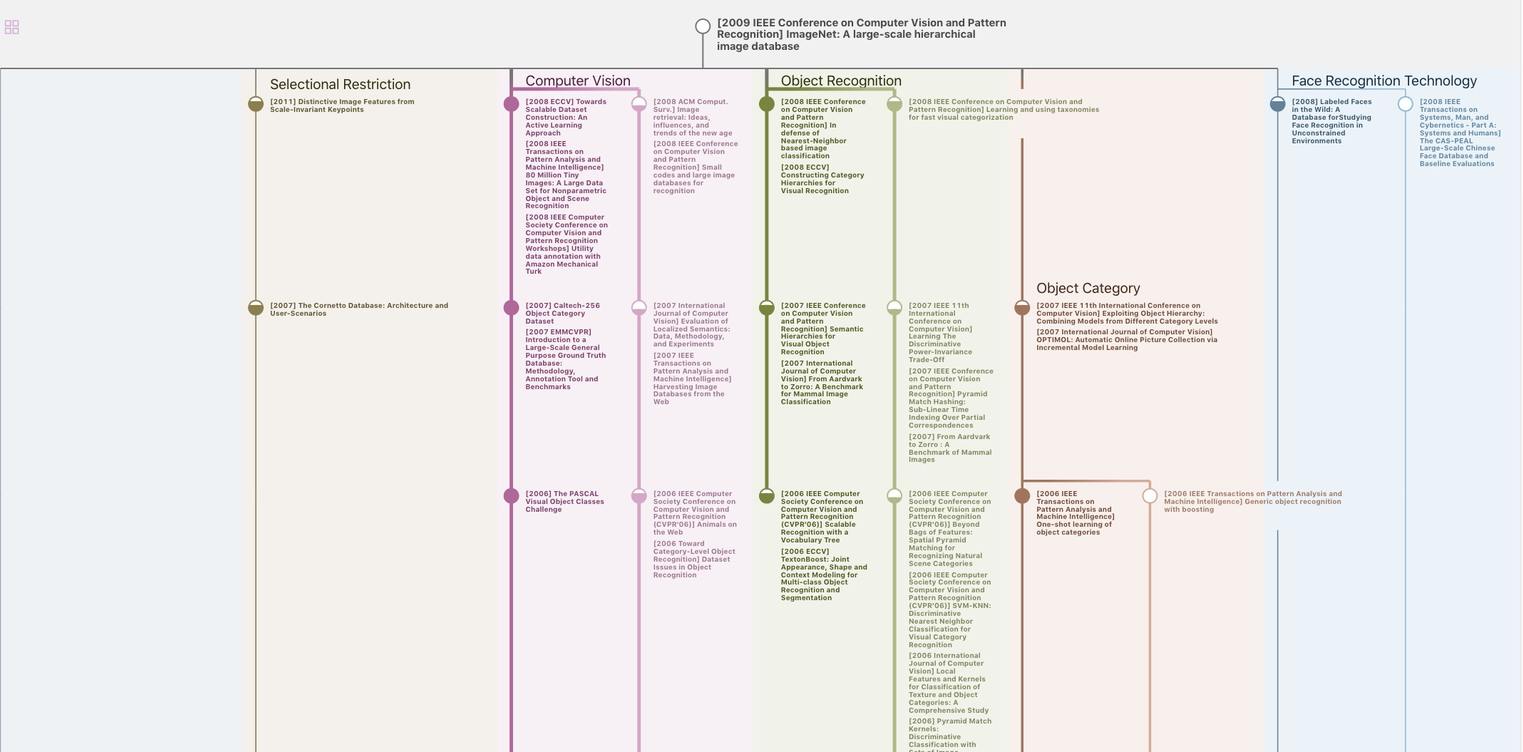
生成溯源树,研究论文发展脉络
Chat Paper
正在生成论文摘要