Learning Representations with a Dynamic Objective Sparse Autoencoder
neural information processing systems(2012)
摘要
The main objective of an auto-encoder is to reconstruct the input signals via a feature representation of latent variables. The number of latent variables defines the representational capacity limit of the model. For data sets where some or all signals contain noise there is an unnecessary amount of capacity spent on trying to reconstruct these signals. One solution is to increase the number of hidden units to increase the capacity so that there will be enough capacity to capture the valuable information. Another solution is to pre-process the signals or perform a manual signal selection. In this paper, we propose a method that will dynamically change the objective function depending on the current performance of the model. This is done by weighting the objective function individually for each input unit in order to guide the feature leaning and decrease the influence that problematic signals have on the learning of features. We evaluate our method on various multidimensional time-series data sets and handwritten digit recognition data sets and compare our results with a standard sparse auto-encoder.
更多查看译文
关键词
computer science
AI 理解论文
溯源树
样例
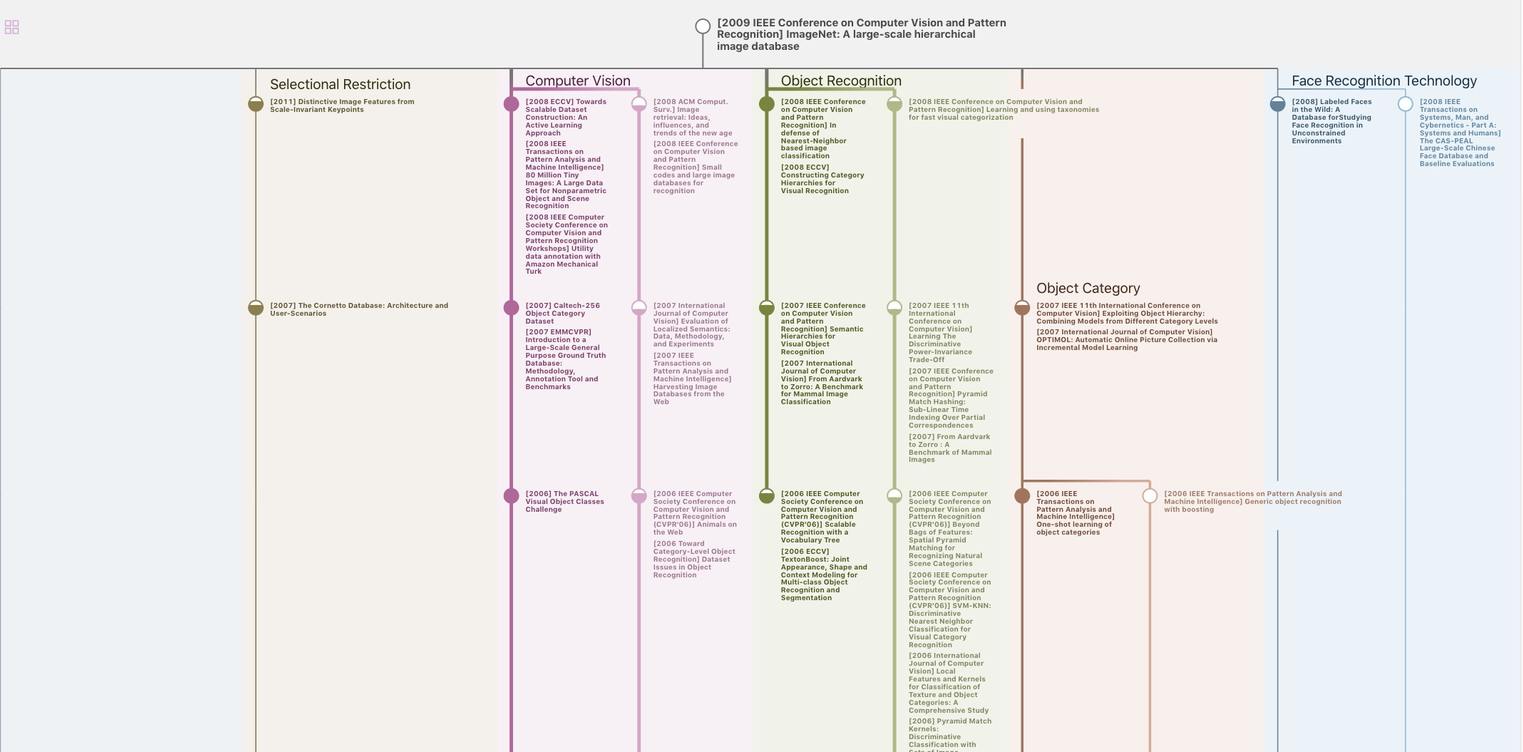
生成溯源树,研究论文发展脉络
Chat Paper
正在生成论文摘要