Empirical Study of Time Efficiency and Accuracy of Support Vector Machines Using an Improved Version of PSVM
parallel and distributed processing techniques and applications(2015)
摘要
We present a significantly improved implementation of a parallel SVM algorithm (PSVM) together with a comprehensive experimental study. Support Vector Machines (SVM) is one of the most well-known machine learning classification techniques. PSVM employs the Interior Point Method, which is a solver used for SVM problems that has a high potential of parallelism. We improve PSVM regarding its structure and memory management for contemporary processor architectures. We perform a number of experiments and study the impact of the reduced column size p and other important parameters as C and gamma on the class-prediction accuracy and training time. The experimental results show that there exists a threshold between the number of computational cores and the training time, and that choosing an appropriate value of p effects the choice of the C and gamma parameters as well as the accuracy.
更多查看译文
关键词
technology,computer science
AI 理解论文
溯源树
样例
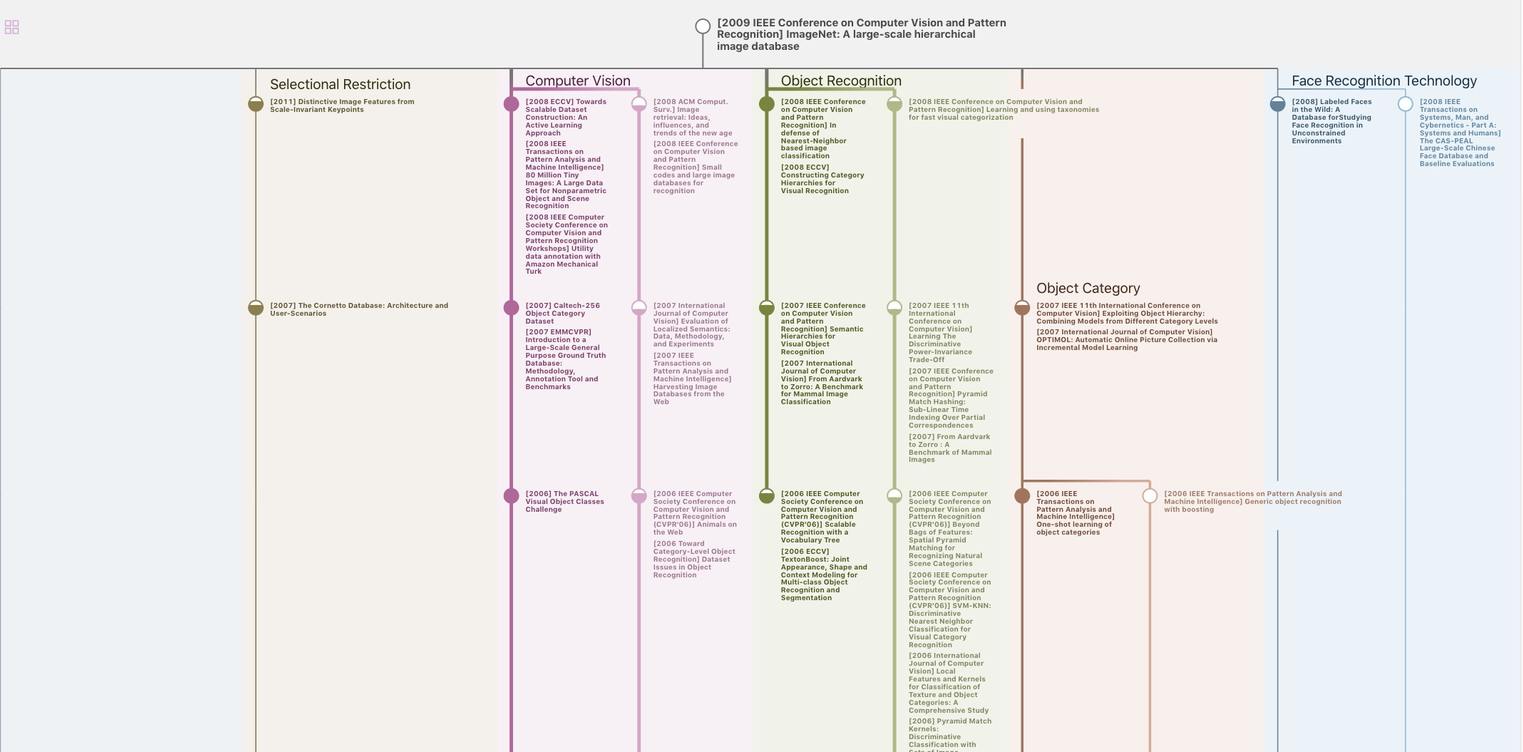
生成溯源树,研究论文发展脉络
Chat Paper
正在生成论文摘要