Social Network and Click-through Prediction with Factorization Machines
knowledge discovery and data mining(2012)
摘要
The two tasks of KDDCup 2012 are to predict the followers of a microblogger (track 1) and to predict the click-through rate of ads (track 2). On rst glance, both tasks look dierent however they share two important challenges. First, the main variables in both problem settings are of large categorical domain. Estimating variable interactions between this type of variables is dicult with standard machine learning models and factorization models have become popular for such data. Secondly, many additional predictor variables are available which requires exible models based on feature engineering to facilitate model denition. In this work, it is shown how Factorization Machines (FM) can be used as a generic approach to solve both tracks. FMs combine the exibility of feature engineering with the advantages of factorization models. This paper shortly introduces FMs and presents for both tasks in detail the learning objectives and how features can be generated. For track 1, Bayesian inference with Markov Chain Monte Carlo (MCMC) is used whereas for track 2, stochastic gradient descent (SGD) and MCMC based solutions are combined.
更多查看译文
AI 理解论文
溯源树
样例
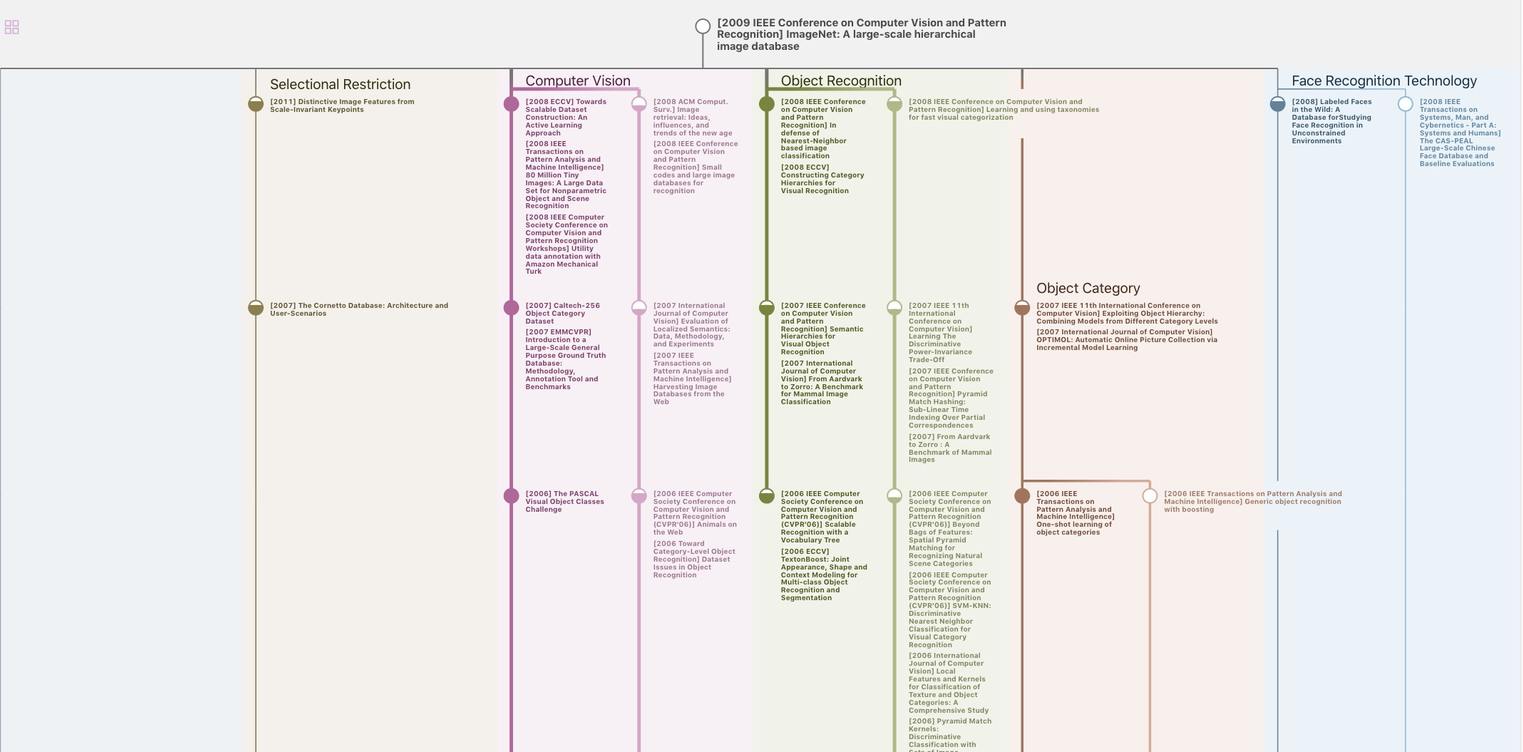
生成溯源树,研究论文发展脉络
Chat Paper
正在生成论文摘要