Fuzzy Local Mean Discriminant Analysis for Dimensionality Reduction
Neural Processing Letters(2015)
摘要
“Fuzzy set” theory can effectively manage the vagueness and ambiguity of the images being degraded by poor illumination component. In this study, we augment mechanism of “fuzzy set” into the algorithm design, and propose fuzzy local mean discriminant analysis (FLMDA) for dimensionality reduction. In FLMDA, the nearest neighborhoods are selected as the local patches. On each local patch, FLMDA redefines the fuzzy local class-means and then constructs the fuzzy local between-class and within-class scatters, respectively. By maximizing the difference of fuzzy local between-class scatter and fuzzy local within-class scatter, FLMDA finds the optimal transformed subspace, in which the local neighbor relationship is preserved while at the same time the compactness and separability are enhanced. The experimental results on the AR face database, Yale face database, UCI Wine dataset and PolyU palmprint database show that FLMDA outperforms the state-of-the-art algorithms.
更多查看译文
关键词
Fuzzy set,Locality,Dimensionality reduction
AI 理解论文
溯源树
样例
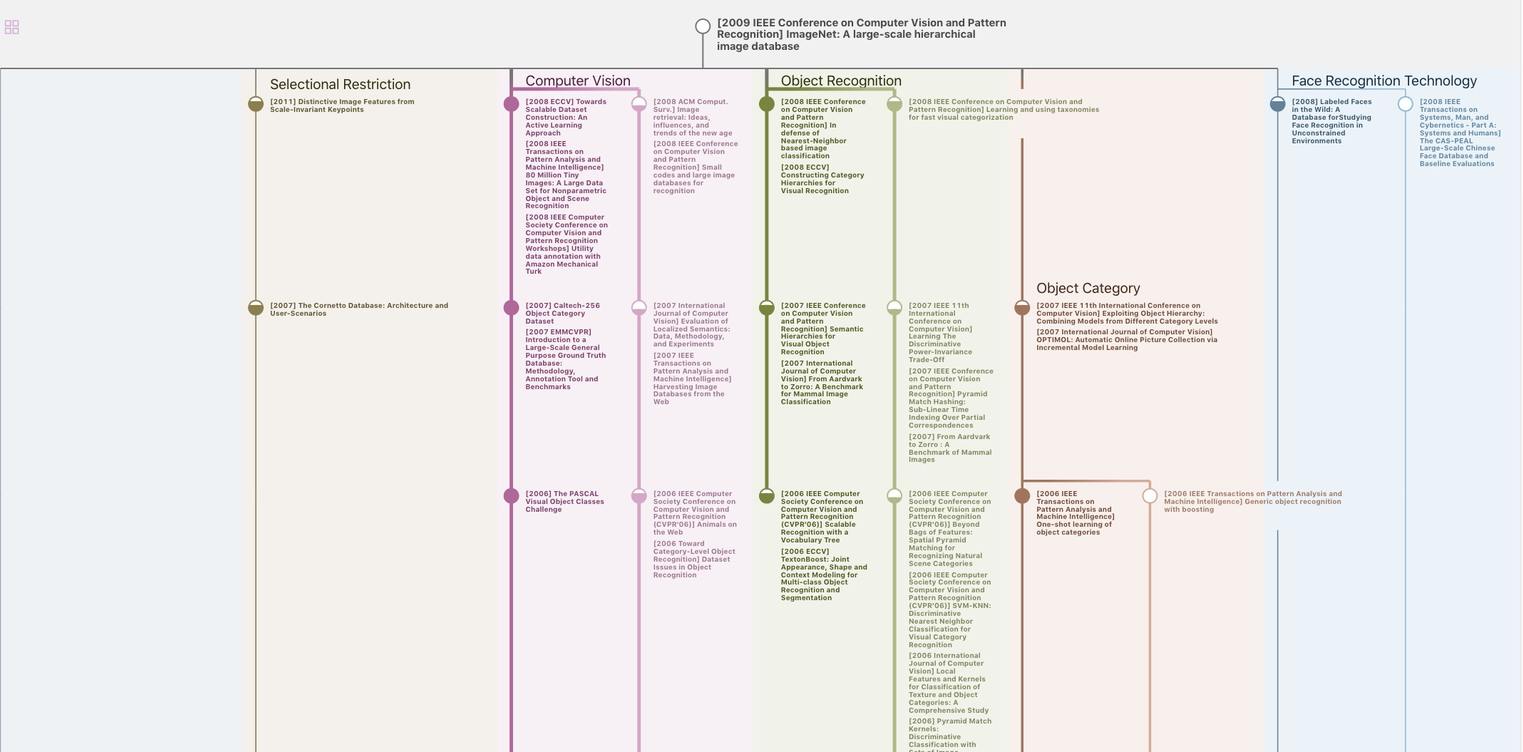
生成溯源树,研究论文发展脉络
Chat Paper
正在生成论文摘要