Bayesian Two-Stage Biomarker-Based Adaptive Design for Targeted Therapy Development
Statistics in Biosciences(2014)
摘要
We propose a Bayesian two-stage biomarker-based adaptive randomization (AR) design for the development of targeted agents. The design has three main goals: (1) to test the treatment efficacy, (2) to identify prognostic and predictive markers for the targeted agents, and (3) to provide better treatment for patients enrolled in the trial. To treat patients better, both stages are guided by the Bayesian AR based on the individual patient’s biomarker profiles. The AR in the first stage is based on a known marker. A Go/No-Go decision can be made in the first stage by testing the overall treatment effects. If a Go decision is made at the end of the first stage, a two-step Bayesian lasso strategy will be implemented to select additional prognostic or predictive biomarkers to refine the AR in the second stage. We use simulations to demonstrate the good operating characteristics of the design, including the control of per-comparison type I and type II errors, high probability in selecting important markers, and treating more patients with more effective treatments. Bayesian adaptive designs allow for continuous learning. The designs are particularly suitable for the development of multiple targeted agents in the quest of personalized medicine. By estimating treatment effects and identifying relevant biomarkers, the information acquired from the interim data can be used to guide the choice of treatment for each individual patient enrolled in the trial in real time to achieve a better outcome. The design is being implemented in the BATTLE-2 trial in lung cancer at the MD Anderson Cancer Center.
更多查看译文
关键词
Adaptive design,Outcome-adaptive randomization,Bayesian Lasso,Predictive and prognostic biomarkers,Personalized medicine,Targeted therapy,Variable selection
AI 理解论文
溯源树
样例
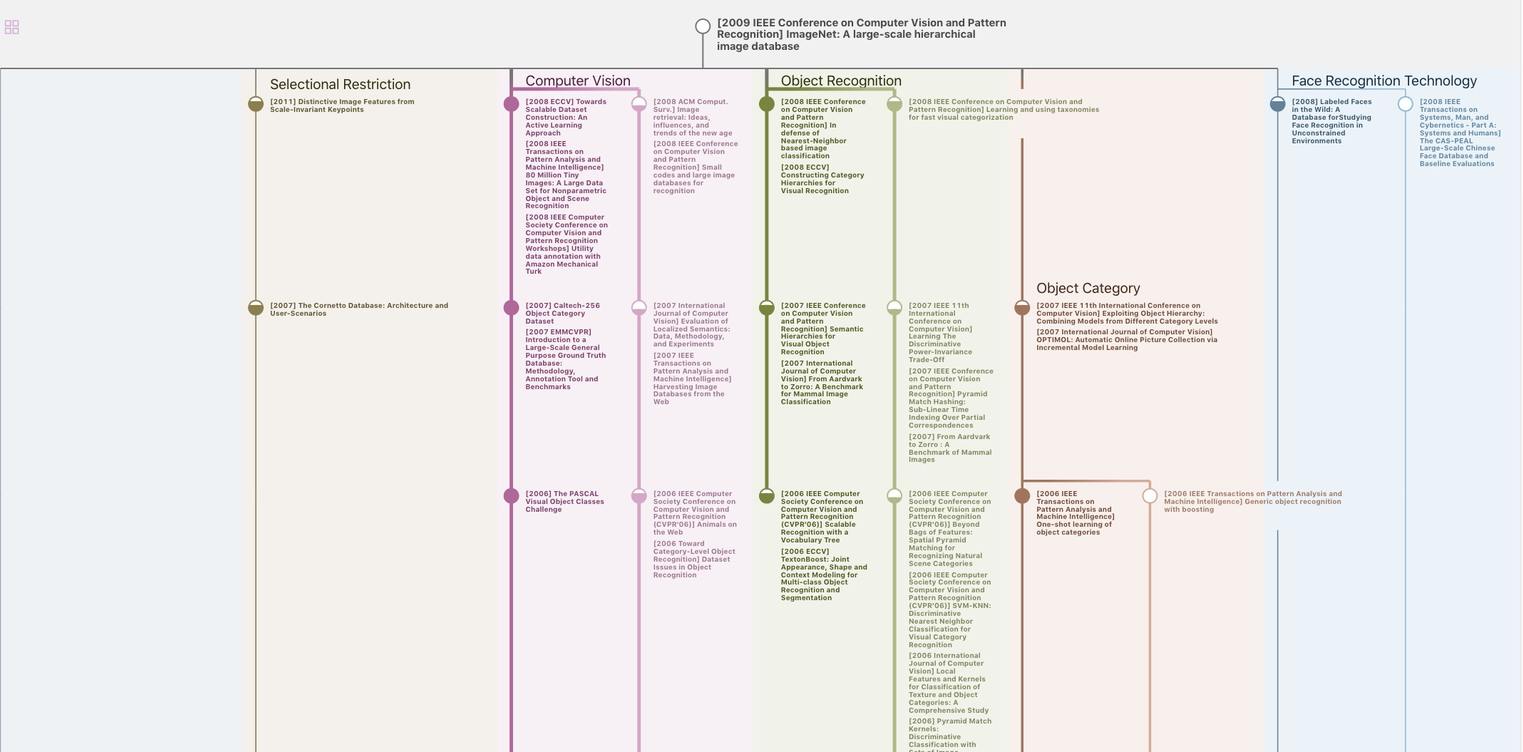
生成溯源树,研究论文发展脉络
Chat Paper
正在生成论文摘要