Multiple Linear Regression and Artificial Neural Network to Predict Blood Glucose in Overweight Patients.
EXPERIMENTAL AND CLINICAL ENDOCRINOLOGY & DIABETES(2016)
摘要
BACKGROUND:Overweight individuals are at higher risk for developing type II diabetes than the general population. We conducted this study to analyze the correlation between blood glucose and biochemical parameters, and developed a blood glucose prediction model tailored to overweight patients.
METHODS:A total of 346 overweight Chinese people patients ages 18-81 years were involved in this study. Their levels of fasting glucose (fs-GLU), blood lipids, and hepatic and renal functions were measured and analyzed by multiple linear regression (MLR). Based the MLR results, we developed a back propagation artificial neural network (BP-ANN) model by selecting tansig as the transfer function of the hidden layers nodes, and purelin for the output layer nodes, with training goal of 0.5×10(-5).
RESULTS:There was significant correlation between fs-GLU with age, BMI, and blood biochemical indexes (P<0.05). The results of MLR analysis indicated that age, fasting alanine transaminase (fs-ALT), blood urea nitrogen (fs-BUN), total protein (fs-TP), uric acid (fs-BUN), and BMI are 6 independent variables related to fs-GLU. Based on these parameters, the BP-ANN model was performed well and reached high prediction accuracy when training 1 000 epoch (R=0.9987).
CONCLUSIONS:The level of fs-GLU was predictable using the proposed BP-ANN model based on 6 related parameters (age, fs-ALT, fs-BUN, fs-TP, fs-UA and BMI) in overweight patients.
更多查看译文
关键词
glucose,overweight,regression,BP-ANN
AI 理解论文
溯源树
样例
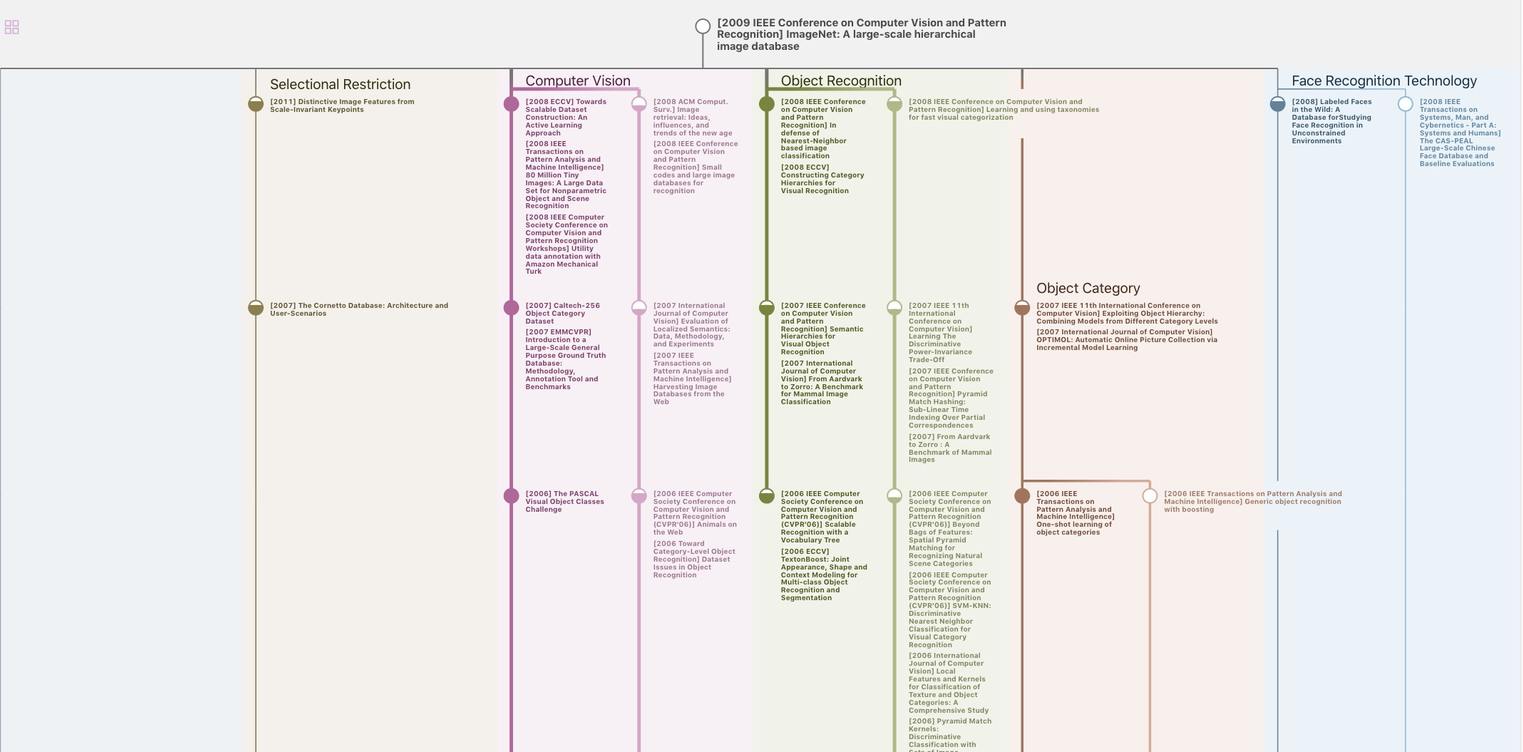
生成溯源树,研究论文发展脉络
Chat Paper
正在生成论文摘要