An Ultra-low power, “Always-On” Camera Front-End for Posture Detection in Body Worn Cameras using Restricted Boltzman Machines
Multi-Scale Computing Systems, IEEE Transactions(2015)
摘要
The Internet of Things (IoTs) has triggered rapid advances in sensors, surveillance devices, wearables and body area networks with advanced Human-Computer Interfaces (HCI). One such application area is the adoption of Body Worn Cameras (BWCs) by law enforcement officials. The need to be ‘always-on’ puts heavy constraints on battery usage in these camera front-ends, thus limiting their widespread adoption. Further, the increasing number of such cameras is expected to create a data deluge, which requires large processing, transmission and storage capabilities. Instead of continuously capturing and streaming or storing videos, it is prudent to provide “smartness” to the camera front-end. This requires hardware assisted image recognition and template matching in the front-end, capable of making judicious decisions on when to trigger video capture or streaming. Restricted Boltzmann Machines (RBMs) based neural networks have been shown to provide high accuracy for image recognition and are well suited for low power and re-configurable systems. In this paper we propose an RBM based “always-on’’ camera front-end capable of detecting human posture. Aggressive behavior of the human being in the field of view will be used as a wake-up signal for further data collection and classification. The proposed system has been implemented on a Xilinx Virtex 7 XC7VX485T platform. A minimum dynamic power of 19.18 mW for a target recognition accuracy while maintaining real time constraints has been measured. The hardware-software co-design illustrates the trade-offs in the design with respect to accuracy, resource utilization, processing time and power. The results demonstrate the possibility of a true “always-on” body-worn camera system in the IoT environment.
更多查看译文
关键词
Algorithms implemented in hardware,Object recognition,Reconfigurability
AI 理解论文
溯源树
样例
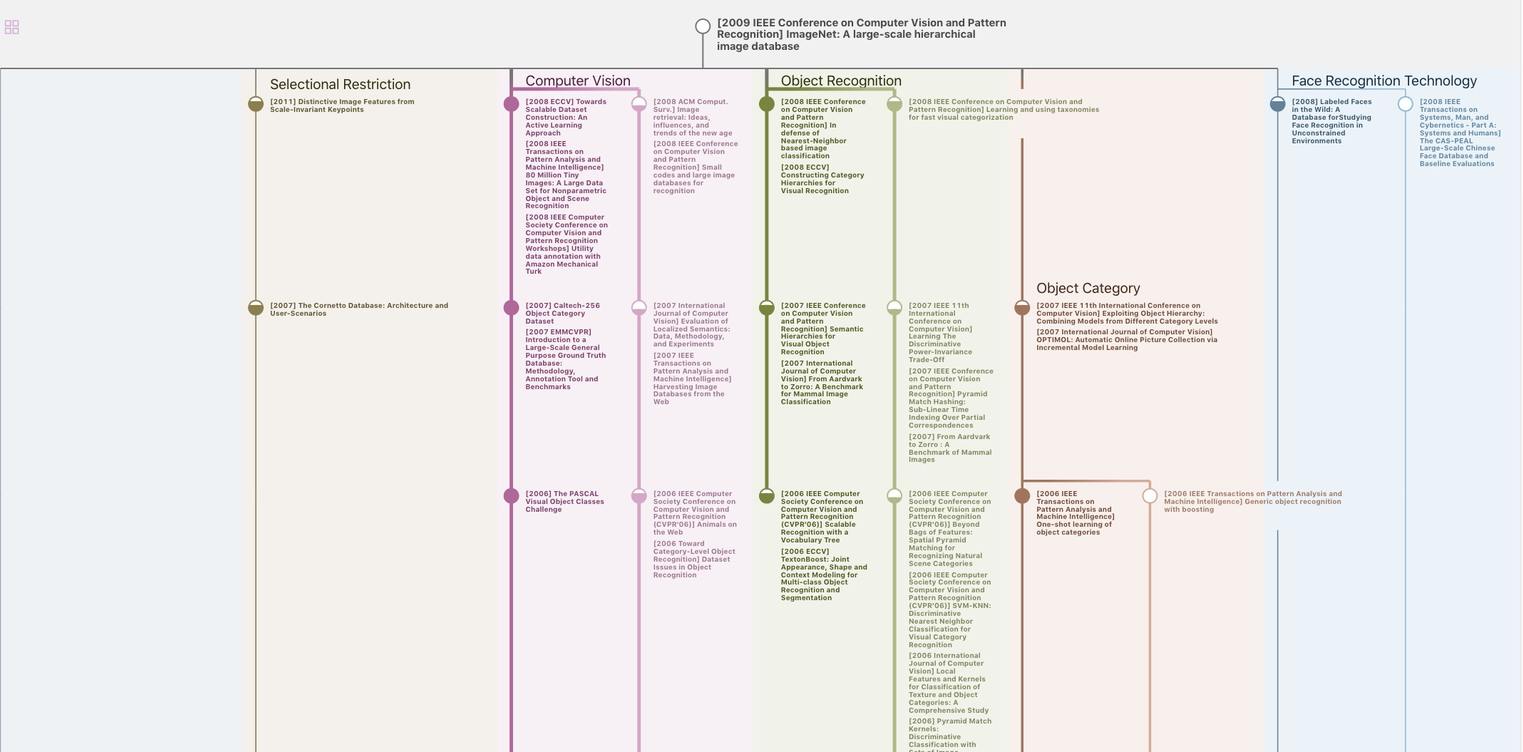
生成溯源树,研究论文发展脉络
Chat Paper
正在生成论文摘要