Speed versus accuracy in visual search: Optimal performance and neural architecture.
JOURNAL OF VISION(2015)
摘要
Searching for objects among clutter is a key ability of the visual system. Speed and accuracy are the crucial performance criteria. How can the brain trade off these competing quantities for optimal performance in different tasks? Can a network of spiking neurons carry out such computations, and what is its architecture? We propose a new model that takes input from V1-type orientation-selective spiking neurons and detects a target in the shortest time that is compatible with a given acceptable error rate. Subject to the assumption that the output of the primary visual cortex comprises Poisson neurons with known properties, our model is an ideal observer. The model has only five free parameters: the signal-to-noise ratio in a hypercolumn, the costs of false-alarm and false-reject errors versus the cost of time, and two parameters accounting for nonperceptual delays. Our model postulates two gain-control mechanisms-one local to hypercolumns and one global to the visual field-to handle variable scene complexity. Error rate and response time predictions match psychophysics data as we vary stimulus discriminability, scene complexity, and the uncertainty associated with each of these quantities. A five-layer spiking network closely approximates the optimal model, suggesting that known cortical mechanisms are sufficient for implementing visual search efficiently.
更多查看译文
关键词
visual search,ideal observer,speed-accuracy tradeoff,spiking neural networks,gain control
AI 理解论文
溯源树
样例
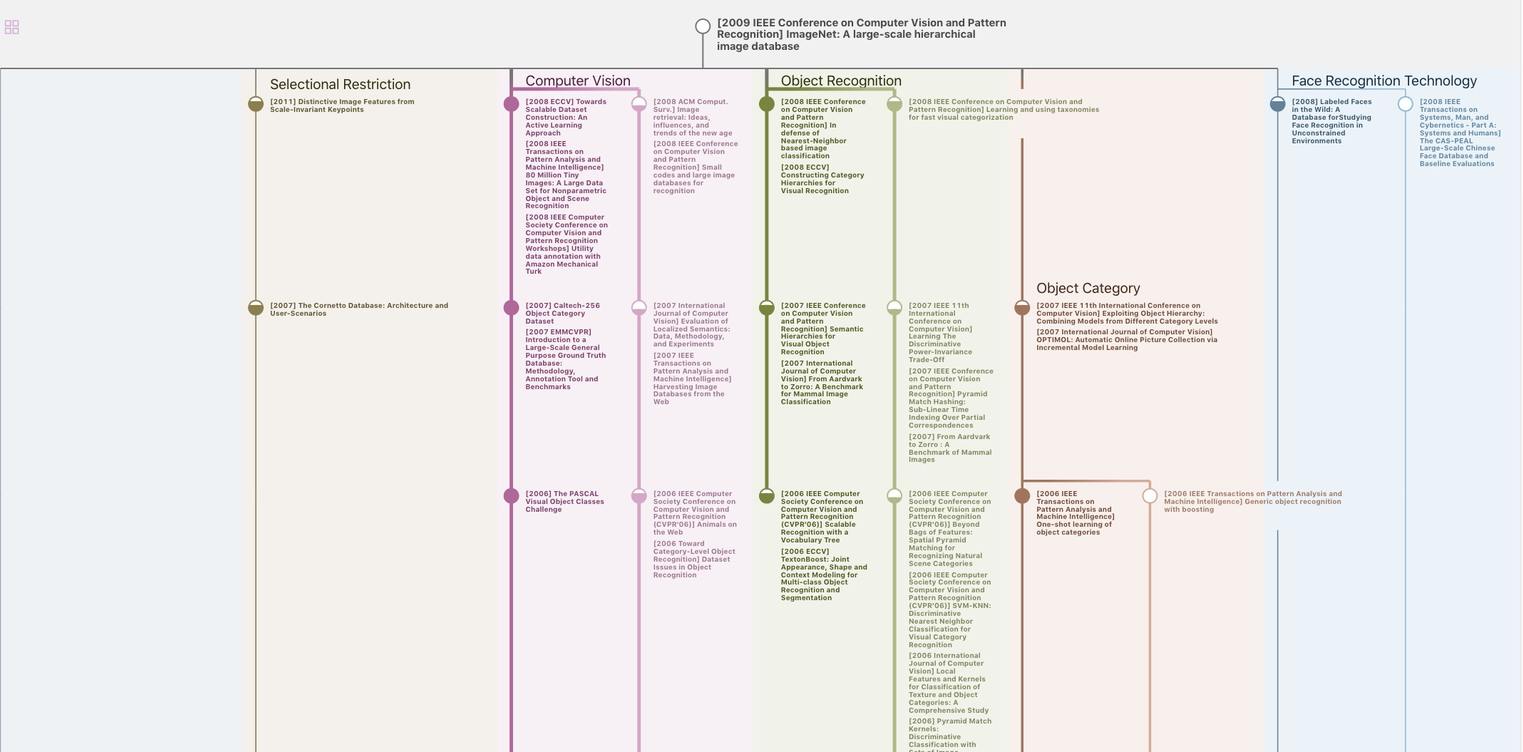
生成溯源树,研究论文发展脉络
Chat Paper
正在生成论文摘要