An adaptive accuracy-weighted ensemble for inter-subjects classification in brain-computer interfacing
Neural Engineering(2015)
摘要
Learning from other subjects and/or sessions led to considerable reduction of calibration time in EEG-based BCIs. However, such learning scheme is not straightforward because of the non-stationary nature of EEG signals. In this paper, we propose an adaptive accuracy-weighted ensemble (AAWE) approach that allows tracking non-stationarity in EEG signals and effectively learning from other subjects. It consists of an ensemble of classifiers, each of which is trained using data recorded from one BCI user. Classifiers' weights are initialized according to their accuracy in classifying calibration data of current BCI user. These weights are updated using ensemble decision during feedback phase, when there is no information about true class labels. The effectiveness of our approach is demonstrated through an empirical comparison with other state of the art classifiers combination strategies.
更多查看译文
关键词
brain-computer interfaces,calibration,electroencephalography,handicapped aids,medical control systems,medical signal processing,signal classification,AAWE,EEG-based BCI,adaptive accuracy-weighted ensemble,brain-computer interfacing,calibration time,classifiers combination strategies,ensemble decision,feedback phase,inter-subjects classification,nonstationarity tracking,Brain-computer interfaces (BCIs),Electroencephalography (EEG),ensemble methods,non-stationarity,transfer learning
AI 理解论文
溯源树
样例
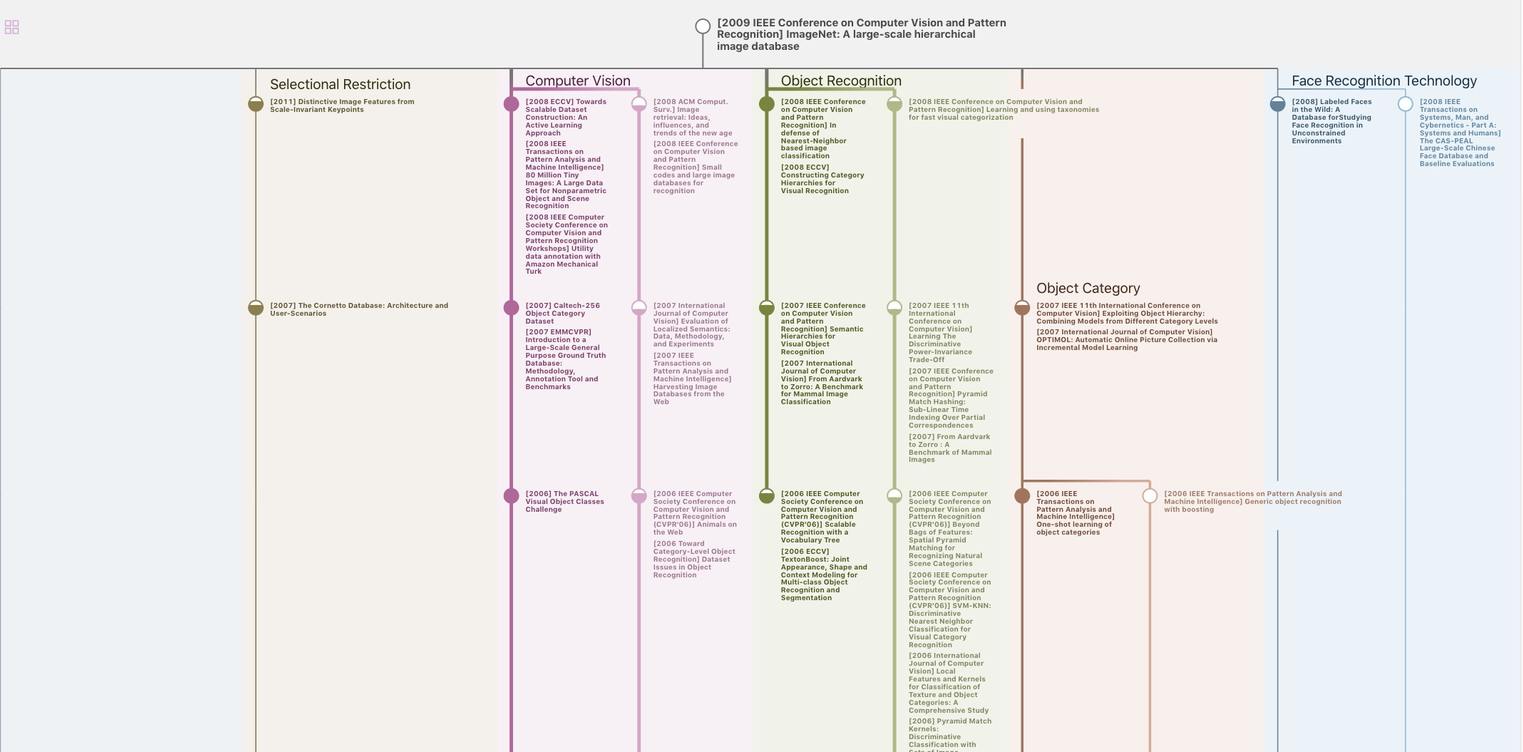
生成溯源树,研究论文发展脉络
Chat Paper
正在生成论文摘要