] Matching Images With Multiple Descriptors: An Unsupervised Approach For Locally Adaptive Descriptor Selection
IEEE TRANSACTIONS ON IMAGE PROCESSING(2015)
摘要
With the aim to improve the performance of feature matching, we present an unsupervised approach for adaptive description selection in the space of homographies. Inspired by the observation that the homographies of correct feature correspondences vary smoothly along the spatial domain, our approach stands on the unsupervised nature of feature matching, and can choose a good descriptor locally for matching each feature point, instead of using one global descriptor. To this end, the homography space serves as the domain for selecting various heterogeneous descriptors. Correspondences obtained by any descriptors are considered as points in the space, and their geometric coherence and spatial continuity are measured via computing the geodesic distances. In this way, mutual verification across different descriptors is allowed, and correct correspondences will be highlighted with a high degree of consistency short geodesic distances here. It follows that one-class SVM can be applied to identifying these correct correspondences, and achieves adaptive descriptor selection. The proposed approach is comprehensively compared with the state-of-the-art approaches, and evaluated on five benchmarks of image matching. The promising results manifest its effectiveness.
更多查看译文
关键词
Image feature matching,descriptor selection,geometric verification,homography space,geodesic distance,one-class SVM
AI 理解论文
溯源树
样例
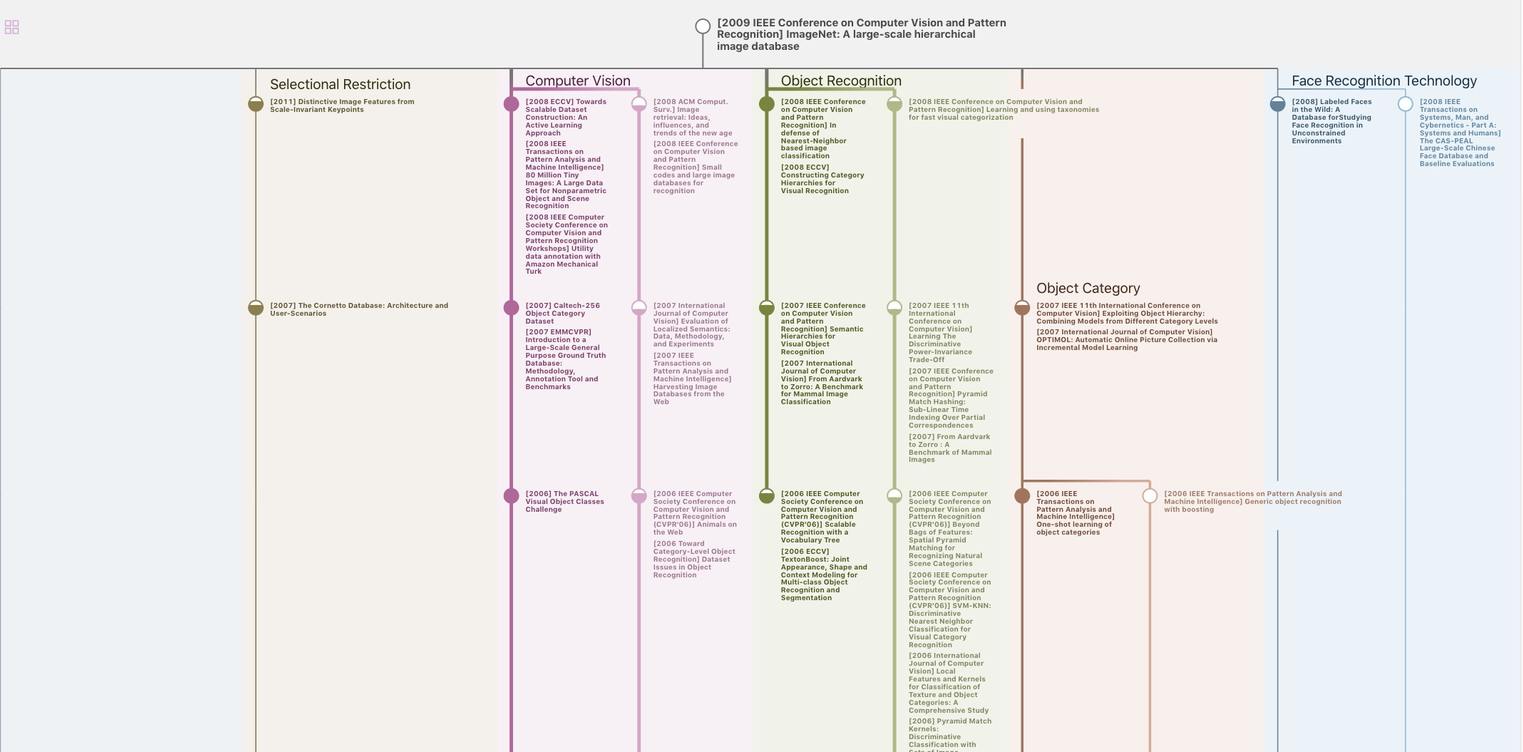
生成溯源树,研究论文发展脉络
Chat Paper
正在生成论文摘要