The Cancer Genome Atlas Pan-Cancer analysis project
Nature Genetics(2013)
摘要
The Cancer Genome Atlas (TCGA) Research Network has profiled and analyzed large numbers of human tumors to discover molecular aberrations at the DNA, RNA, protein and epigenetic levels. The resulting rich data provide a major opportunity to develop an integrated picture of commonalities, differences and emergent themes across tumor lineages. The Pan-Cancer initiative compares the first 12 tumor types profiled by TCGA. Analysis of the molecular aberrations and their functional roles across tumor types will teach us how to extend therapies effective in one cancer type to others with a similar genomic profile. Cancer can take hundreds of different forms depending on the location, cell of origin and spectrum of genomic alterations that promote oncogenesis and affect therapeutic response. Although many genomic events with direct phenotypic impact have been identified, much of the complex molecular landscape remains incompletely charted for most cancer lineages. That cancer is fundamentally a genomic disease is now well established. Early on, large numbers of oncogenes were identified using functional assays on genetic material from tumors in positive-selection systems1, 2, 3, and a subset of tumor suppressor genes was identified by analyzing loss of heterozygosity4. More recently, systematic cancer genomics projects, including TCGA (Box 1), have applied emerging technologies to the analysis of specific tumor types. This disease-specific focus has identified novel oncogenic drivers and the genes contributing to functional change5, 6, 7, has established definitions of molecular subtypes8, 9, 10, 11, 12 and has identified new biomarkers on the basis of genomic, transcriptomic, proteomic and epigenomic alterations. Some of these biomarkers have clinical implications13, 14. For example, we now view ductal breast cancer as a collection of distinct diseases whose major subtypes (for example, luminal A, luminal B, HER2 and basal-like) are managed differently in the clinic; the outcomes for metastatic melanoma have improved as a result of therapeutic targeting of BRAFV600 mutations15; and the fraction of lung cancers treated with targeted agents is increasing with the discovery of likely driver aberrations in most lung tumors16, 17. Large-scale processes that shape cancer genomes have similarly been identified. Analyses of chromothripsis18 and chromoplexy19, which involve the breakage and rearrangement of chromosomes at multiple loci, and kataegis20, which involves hypermutational processes associated with genomic rearrangements, are providing insights into tumor evolution (see Garraway and Lander21 for a review). Increased numbers of tumor sample data sets enhance the ability to detect and analyze molecular defects in cancers. For example, driver genes can be pinpointed more precisely by narrowing regions affected by amplification and deletion to smaller segments of the chromosome using data on recurrent events across tumor types. The use of large cohorts has enabled DNA sequencing to uncover a list of recurrent genomic aberrations (mutations, amplifications, deletions, translocations, fusions and other structural variants), both known and novel, as common events across tumor types22. However, 'long tails' in the distributions of aberrations among samples have also been uncovered23. Indeed, a majority of the TCGA samples have distinct alterations not shared with other samples in their cohort. Despite the apparent uniqueness of each individual tumor in this regard, the set of molecular aberrations often integrates into known biological pathways that are shared by sets of tumor samples. In other cases, rare somatic mutations can be implicated as drivers by aggregating events across tumor types to improve the detection of patterns, for example, hotspot mutations in DNA segments that encode particular protein domains, leading to the identification of potential new drug targets. Determining whether rare aberrations are drivers (oncogenic contributors) or just passengers (clonally propagated with neutral effect) and whether they are clinically actionable will require further functional evaluation as well as the analysis of additional tumors to increase power. The identification of more driver aberrations and acquired vulnerabilities for each individual tumor will undoubtedly boost personalized care. Developing treatments that target the ~140 drivers22 validated so far, however daunting, appears possible; devising one-off therapies for the thousands of aberrations in the long tails will be much more challenging. Although important general principles have emerged from decades of study24, 25, until recently, most research on the molecular, pathological and clinical natures of cancers has been 'siloed' by tumor type26. One has only to glance at the directory of oncology departments in any major cancer center to realize that medical and surgical cancer care are, for the most part, also divided by disease as defined by organ of origin. This framework has made sense for generations, but the results of molecular analysis are now calling this view into question; cancers of disparate organs have many shared features, whereas, conversely, cancers from the same organ are often quite distinct. Important similarities among tumor subtypes from different organs have already been identified. For example, TP53 mutations drive high-grade serous ovarian, serous endometrial and basal-like breast carcinomas, all of which share a global transcriptional signature involving the activation of similar oncogenic pathways10, 27. Similarly, ERBB2-HER2 is mutated and/or amplified in subsets of glioblastoma, gastric, serous endometrial, bladder and lung cancers. The result, at least in some cases, is responsiveness to HER2-targeted therapy, analogous to that previously observed for HER2-amplified breast cancer. Other commonalities across tumor types include inherited and somatic inactivation of the BRCA1-BRCA2 pathway in both serous ovarian and basal-like breast cancers, microsatellite instability in colorectal and endometrial tumors, and the recently identified POLE-mediated ultramutator phenotype characterized by extremely high mutation rates, common to both colon and endometrial cancers12, 27, 28. Conversely, there are important cases in which the same genetic aberrations have very different effects depending on the organ within which they arise. A prime example is provided by the NOTCH gene family, which is inactivated in some squamous cell cancers of the lung, head and neck29, skin30 and cervix31 but activated by mutation in leukemias32. Such examples illustrate the importance of developing a comprehensive perspective across tumors, independent of histopathologic diagnosis; shared molecular patterns will enable etiologic and therapeutic discoveries in one disease that can be applied to another. Importantly, integrative interpretation of the data will help identify how the consequences of mutations vary across tissues, with important therapeutic implications. Relatively rare cancers, such as childhood malignancies, in particular stand to benefit from such an approach. We know much more about the molecular details of major cancers than we did just a few years ago, but once a cancer is metastatic it remains incurable with few exceptions. Only time will tell whether the integration of molecular characteristics with data on histology, organ site and metastatic location will contribute to an improvement in patient outcomes. But the balance is shifting in this direction. Hence, the goal of the Pan-Cancer project is to identify and analyze aberrations in the tumor genome and phenotype that define cancer lineages as well as to identify aberrations that transcend particular lineages. This report outlines the scope of the project and introduces the first coordinated set of manuscripts to be published from the enterprise. To gain analytical breadth—defining commonalities, differences and emergent themes across cancer types and organs of origin—TCGA launched the Pan-Cancer analysis project at a meeting held on 26–27 October 2012 in Santa Cruz, California. The Pan-Cancer project is a coordinated initiative whose goals are to assemble coherent, consistent TCGA data sets across tumor types, as well as across platforms, and then to analyze and interpret these data (Box 2). Within 2 months of the project's launch, a 'data freeze' was declared on the first 12 TCGA tumor types, each profiled using 6 different genomic, epigenomic, transcriptional and proteomic platforms (Fig. 1 and Table 1). Since that time, the aggregated data sets have been quality controlled, analyzed statistically and interpreted by a consortium of researchers, principally members of the TCGA Research Network (Fig. 2). The Pan-Cancer project lays the framework for an analytic process that, in the future, will include the integration of new tumor types and data from TCGA and other such enterprises. There are currently major consortium efforts in pediatric cancers (TARGET; Therapeutically Applicable Research to Generate Effective Treatments) and adult cancers (ICGC; International Cancer Genomics Consortium), as well as smaller projects by research teams around the world. A critical component of such efforts will be the functional validation of aberrations in individual genes in team science efforts such as CTD2 (Cancer Target Discovery and Development) and the elucidation of pathway and network relationships in programs such as the US National Cancer Institute's Integrative Cancer Biology Program. A number of investigations that go beyond the single-tumor perspective are being addressed in the collection of Pan-Cancer manuscripts. Examples of the kinds of questions addressed by these investigations are given below. Can increases in statistical power help to distinguish new driver mutations from the background of passenger mutations as the sample size is increased by aggregating the 12 tumor types? Assembled Pan-Cancer data have, in fact, enabled the identification of new patterns of genomic drivers. New computational approaches that leverage cross-tumor principles of replication timing and gene expression correlated with background mutation rates now enable the identification of frequently mutated genes while eliminating many false-positive and false-negative calls made in several single-tumor-type projects33. Further, the power to identify multiple signals of positive selection has increased the ability to distinguish 'driver' from 'passenger' aberrations34. What tissue associations underlie the major genomic structural changes in cancer? Improved methods for the analysis of structural variation of large chromosome segments have refined the ability to identify genomic and epigenetic regulators in multiple peak regions seen only by collating data across different cancer types. Tissue-associated patterns have now been established for the rate and timing of whole-genome duplication events35. What pathways emerge as critical and potentially actionable when all mutational events across many tissues are considered together? New classes of mutations, such as those in chromatin-remodeling genes, are emerging as cancer drivers identified only by (i) collecting less frequent events across tumor types, (ii) integrating event types such as mutations, copy number changes and epigenetic silencing, (iii) combining multiple algorithms to identify predicted drivers34 and (iv) aggregating genes using gene networks and pathways36. Can an increase in the number of samples enhance analysis of the co-occurrence and mutual exclusivity of gene aberrations and improve the ability to distinguish driver aberrations from passengers? A bird's-eye view of genomic and epigenomic events yields a 'fate map' of the alternative routes to carcinogenesis in a decision tree that spans tissue boundaries37. Can molecular subtypes be delineated to disentangle tissue-specific from tissue-independent components of disease? Analyses of the epi-genome, transcriptome and proteome show a strong influence of tissue on the state of altered pathways in tumor cells. For instance, analysis of the gene expression landscape reinforces the dominant tissue dependence of altered pathways and complements simultaneous profiling of over a hundred proteins important in cancer38. Using all of the tumor types together allows for any tumor-specific signals to be subtracted from the data sets. Intriguingly, subtracting tissue-specific signal from DNA microarray gene expression data sets identifies signatures of immune stromal influence that transcend tumor type boundaries (R. Verhaak, personal communication). Further, events that are common across lineages become apparent in a cross-tumor analysis38. Examples are the hormonal dependencies of breast, ovarian and endometrial cancers and a common 'squamous cell' signature across head and neck, lung, cervical and bladder cancers. Which events actionable in one tumor lineage are also actionable in another tumor lineage, potentially increasing the range of indications for specific targeted therapeutics? A systematic evaluation of machine-learning approaches is needed to highlight methodological principles for predicting patient outcomes using integrated information across tissues (H. Liang, personal communication). Several data integration challenges place unavoidable limitations on cross-tumor analysis at the current time. A key challenge is the integration of data that have been generated on different platforms or updates of the same platform, as technologies improve. In the Pan-Cancer studies, for example, there have been transitions to much higher density DNA methylation arrays, use of different exome capture technologies, addition of RNA sequencing to microarray-based RNA characterization and increases in the quality and number of antibodies available for reverse-phase proteomic arrays (RPPAs). A series of analyses of batch effects has been carried out to assess systematic and platform-specific biases (R. Akbani, personal communication). However, more work is needed to establish best practices for minimizing unwanted batch effects while preserving biological signals. The nature and quality of available clinical data vary widely by cancer type. Differences in these data limit the ability to establish one-size-fits-all norms for the comparison of demographic information, histopathologic characterization, behavioral context and clinical outcomes. For example, the Pan-Cancer survival data are relatively robust for serous ovarian cancer because of its poor prognosis but are still immature for breast and endometrial cancers, as (thankfully) most patients with these cancers do better for longer periods of time. Certain data elements are routinely collected only when they are anticipated to be relevant (for example, the smoking history of patients with lung, bladder and head and neck cancers). Clear viral etiologies have been identified in several solid tumor types, including head and neck cancer, cervical cancer, Kaposi's sarcoma and hepatocellular carcinoma. However, a pan-cancer analysis of the infectious etiologies of other cancers could not be conducted at present because infection status was recorded for only some tumors and tumor types (as an optional data element). Finally, tumor stage and grade are not easily comparable across different tumor types because, for good reason, each tumor type has its own system. This set of challenges to cross-tumor analysis highlights the fact that current clinical practice is largely conducted according to classification by tissue or organ. Statistically speaking, care must be taken to ensure that the increased sample size achieved by cross-cancer comparison does not lead to increased false-negative rates for discovery (for example, by 'diluting out' an important mutation specific to one disease) or false-positive rates (for example, by compounding false positives known to result from current single-tumor investigations33). Tumor lineage has an important role in the observed patterns of co-aberrations and gene expression profiles that indicate different consequences of seemingly similar events, for example, involving the same gene(s) or amplicon(s). Likewise, new methods for accurately probing cross-tumor trends will need to account explicitly for differences across tissues in mutation rates, copy number changes on the focal and arm-level scales, and the prevalence of other co-occurring events in the genetic and epigenetic backgrounds. Despite these challenges, the collection of Pan-Cancer publications presented here represents a landmark in the continuing effort to understand the common and contrasting biologies of cancers from a molecular perspective. Still, major questions amenable to further cross-tumor investigations remain (Box 3), and the techniques used to compare different tumors will undoubtedly improve with use, time and further collaborative efforts. The Pan-Cancer project represents one of the first of what will surely be many efforts to coordinate analysis across the molecular landscape of cancer, especially as additional tumor types are investigated in large numbers. Further increasing the number of samples per tumor type and the variety of tumor types will improve our ability to detect rare driver events in heterogeneous tumor samples. But the true power will come from a detailed analysis across tumor types—with links to high-quality clinical outcomes and eventual experimental validation and clinical trials to test the hypotheses that emerge. Technologies such as laser capture microdissection and cell sorting will improve the ability to distinguish whether omic signals arise from malignant or stromal cells. Histone profiling, protein analysis based on mass spectrometry and deconvolution of tumor heterogeneity through single-cell sequencing are examples of technologies expected to add important new dimensions of information. Continued efforts to identify the progenitor cells of tumors will enable universal properties to be distinguished from parochial ones. Clone-level and other types of studies may identify even more connections among tumor types. Longitudinal genomic studies on primary resected tumors paired with their local recurrences and/or metastases will be undertaken by large consortium efforts, which have heretofore been restricted to primary disease and have lacked information about response to treatment. The characteristics of primary tumors may change markedly when they metastasize to distant sites, particularly bone and brain. Analysis of metastasis across tumor types will therefore be highly informative. The power of cross-tumor analysis will increase as technologies for monitoring individual tumor cells at high resolution come into play. Now that the price of genome sequencing has fallen, the next Pan-Cancer enterprise will be able to analyze large numbers of whole-genome sequences across tumor types. Whole-genome analysis will complement the current studies by shedding light on mutational processes in the noncoding parts of the genome, which have not been as well explored so far. This expanded analysis will bring focus to disruptions in promoter and enhancer sites and aberrations in noncoding RNAs, as well as the genomic integration processes at work in tumor evolution that result from mobile endogenous and exogenous DNA elements such as retrotransposons and viruses. Whole-genome sequencing will create a backdrop against which genome-wide association studies can relate inherited predispositions to particular forms of cancer. Systems-oriented approaches, based on relevant pathways and networks, will add to the therapeutic opportunities that arise from the wealth of data. Experimental follow-up will be critical to assess the functional consequences and therapeutic liabilities of these new findings. The hope is that investigations across tumor type such as the Pan-Cancer project will ultimately inform clinical decision-making. We hope such studies will enable the discovery of novel therapeutic agents that can be tested clinically—perhaps in novel adaptive, biomarker-based clinical trials that cross boundaries between tumor types. Toward this end, TCGA Pan-Cancer data sets have been made available publicly in one location. Although coordination remains a challenge, the data sets comprise an unequalled resource for the integrative analysis of cancer in its many forms. A key challenge is the development of clinical trial strategies for connecting subsets of tumors from different tissues in terms of molecular signatures. Recent analyses of pharmacological profiling experiments across a diverse panel of cancer cell lines has suggested that common genetic alterations can sometimes predict response to therapy across multiple cell lineages39, 40, 41, 42. Biomarker-based design of clinical trials can increase statistical power, greatly decreasing the size, expense and duration of clinical trials. The number and size of omic data sets on cancer available to the research community for mining and exploring continue to expand rapidly, and computational tools to derive insights into the fundamental causes of cancer are becoming more powerful. It is important to note that the full potential of the enterprise will be realized only over time and with broader efforts. Still, the collection of TCGA Pan-Cancer publications represents a significant contribution to a new period of discovery in cancer research. Download references We thank J. Zhang for the administrative coordination of TCGA Pan-Cancer Analysis Working Group activities, C. Perou and K. Hoadley for contributions to Figure 1, and A. Margolin, D. Wheeler, M. Meyerson and L. Ding for comments on early drafts of the manuscript. The study was funded by the National Cancer Institute and the National Human Genome Research Institute.
更多查看译文
关键词
Cancer,Genomics,Biomedicine,general,Human Genetics,Cancer Research,Agriculture,Gene Function,Animal Genetics and Genomics
AI 理解论文
溯源树
样例
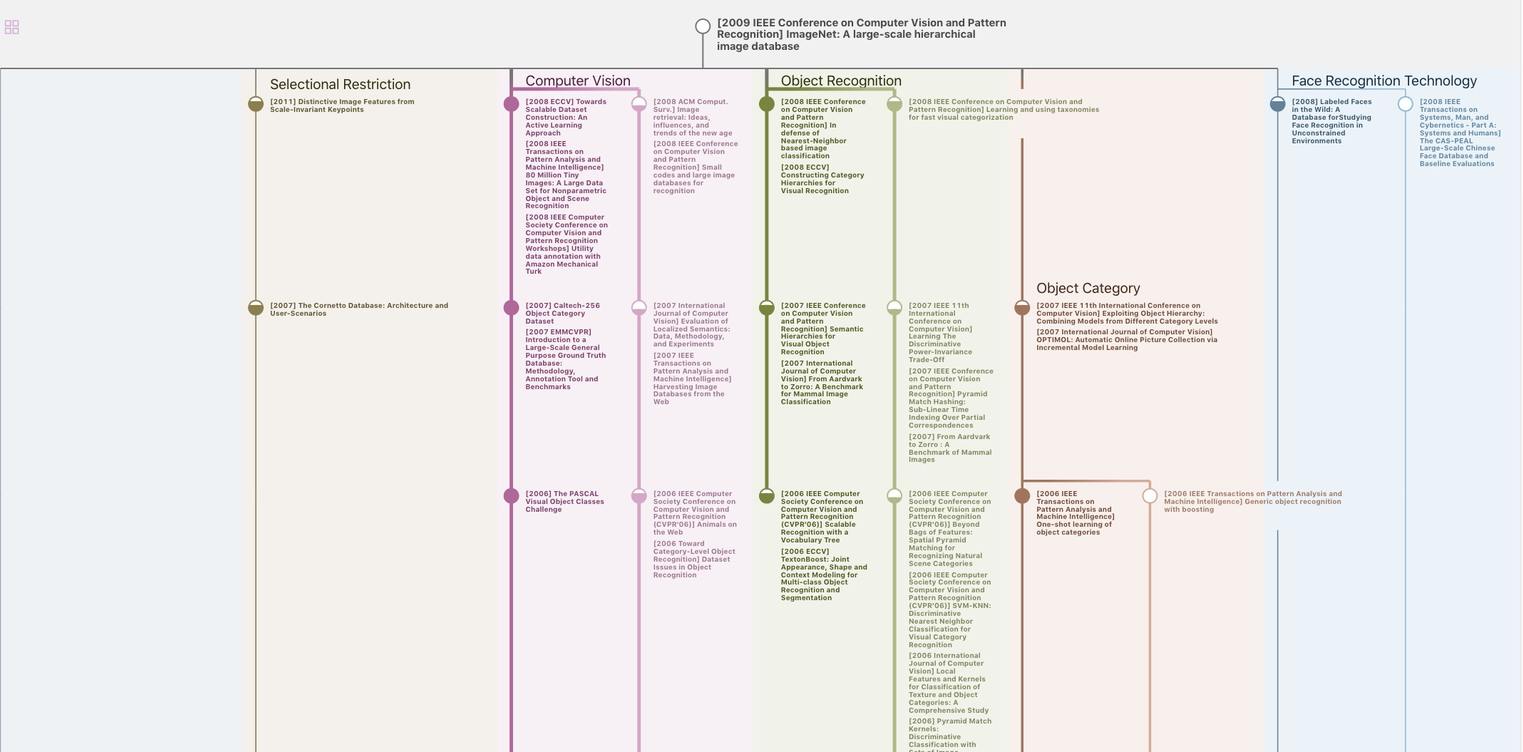
生成溯源树,研究论文发展脉络
Chat Paper
正在生成论文摘要