Manhattan rule training for memristive crossbar circuit pattern classifiers
Intelligent Signal Processing(2015)
摘要
We investigated batch and stochastic Manhattan Rule algorithms for training multilayer perceptron classifiers implemented with memristive crossbar circuits. In Manhattan Rule training, the weights are updated only using sign information of classical backpropagation algorithm. The main advantage of Manhattan Rule is its simplicity, which leads to more compact hardware implementation and faster training time. Additionally, in case of stochastic training, Manhattan Rule allows performing all weight updates in parallel, which further speeds up the training procedure. The tradeoff for simplicity is slightly worse classification performance. For example, simulation results showed that classification fidelity on Proben1 benchmark for memristor-based implementation trained with batch Manhattan Rule were comparable to that of classical backpropagation algorithm, and about 2.8 percent worse than the best reported results.
更多查看译文
关键词
backpropagation,pattern classification,Proben1 benchmark,backpropagation algorithm,manhattan rule training,memristive crossbar circuit pattern classifier,multilayer perceptron classifier,stochastic manhattan rule algorithm,Artificial neural network,Crossbar memory,Memristor,Pattern classification,Training algorithm
AI 理解论文
溯源树
样例
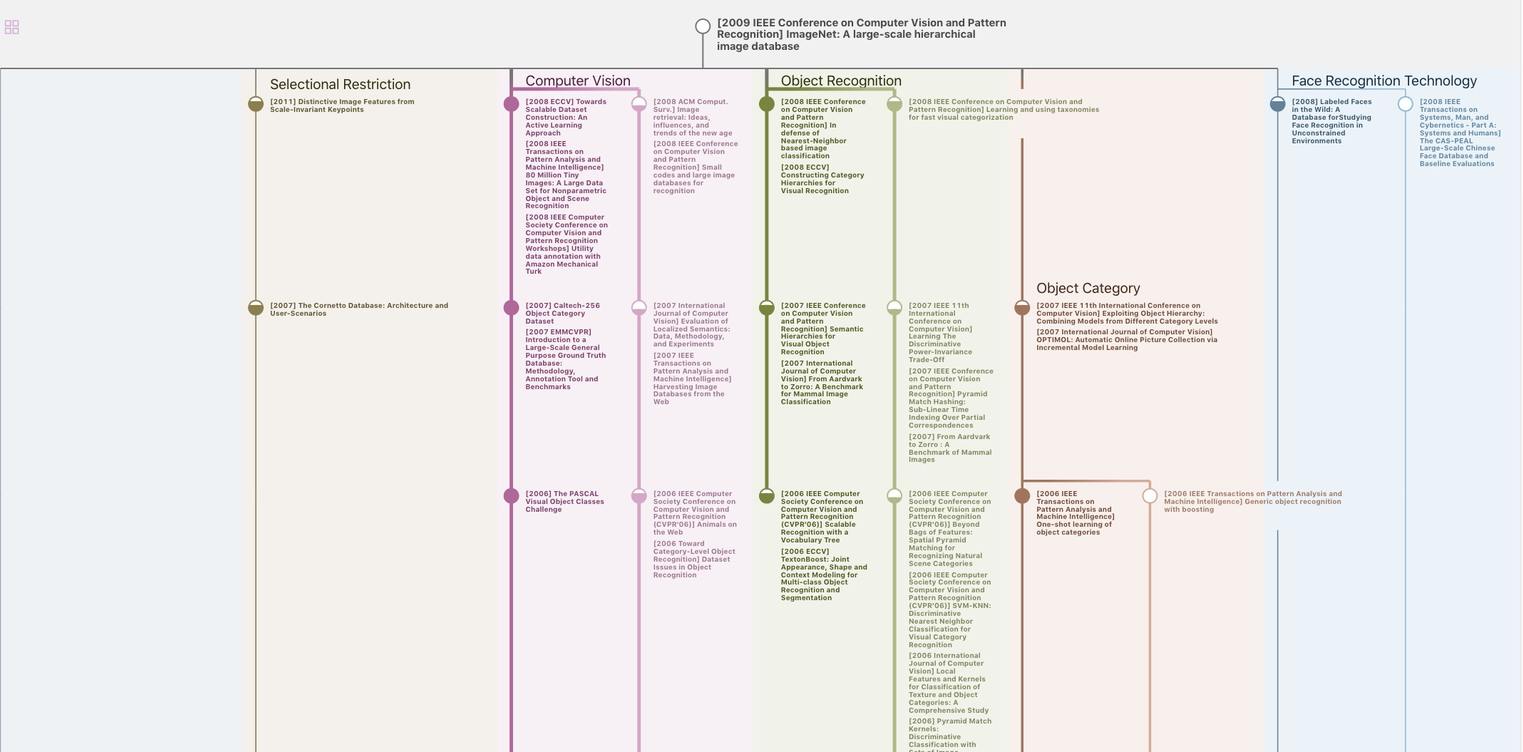
生成溯源树,研究论文发展脉络
Chat Paper
正在生成论文摘要