Memory Technologies For Neural Networks
Memory Workshop(2015)
摘要
Synapses, the most numerous elements of neural networks, are memory devices. Similarly to traditional memory applications, device density is one of the most essential metrics for large-scale artificial neural networks. This application, however, imposes a number of additional requirements, such as the continuous change of the memory state, so that novel engineering approaches are required. In this paper, we briefly review our recent efforts at addressing these needs. We start by reviewing the CrossNet concept, which was conceived to address major challenges of artificial neural networks. We then discuss the recent progress toward CrossNet implementation, in particular the experimental results for simple networks with crossbar-integrated resistive switching (memristive) metal oxide devices. Finally, we review preliminary results on redesigning commercial-grade embedded NOR flash memories to enable individual cell tuning. While NOR flash memories are less dense then memristor crossbars, their technology is much more mature and ready for the development of large-scale neural networks.
更多查看译文
关键词
memristors,flash memory,resistive switching,hybrid circuits,CrossNets,pattern classifiers,neural networks
AI 理解论文
溯源树
样例
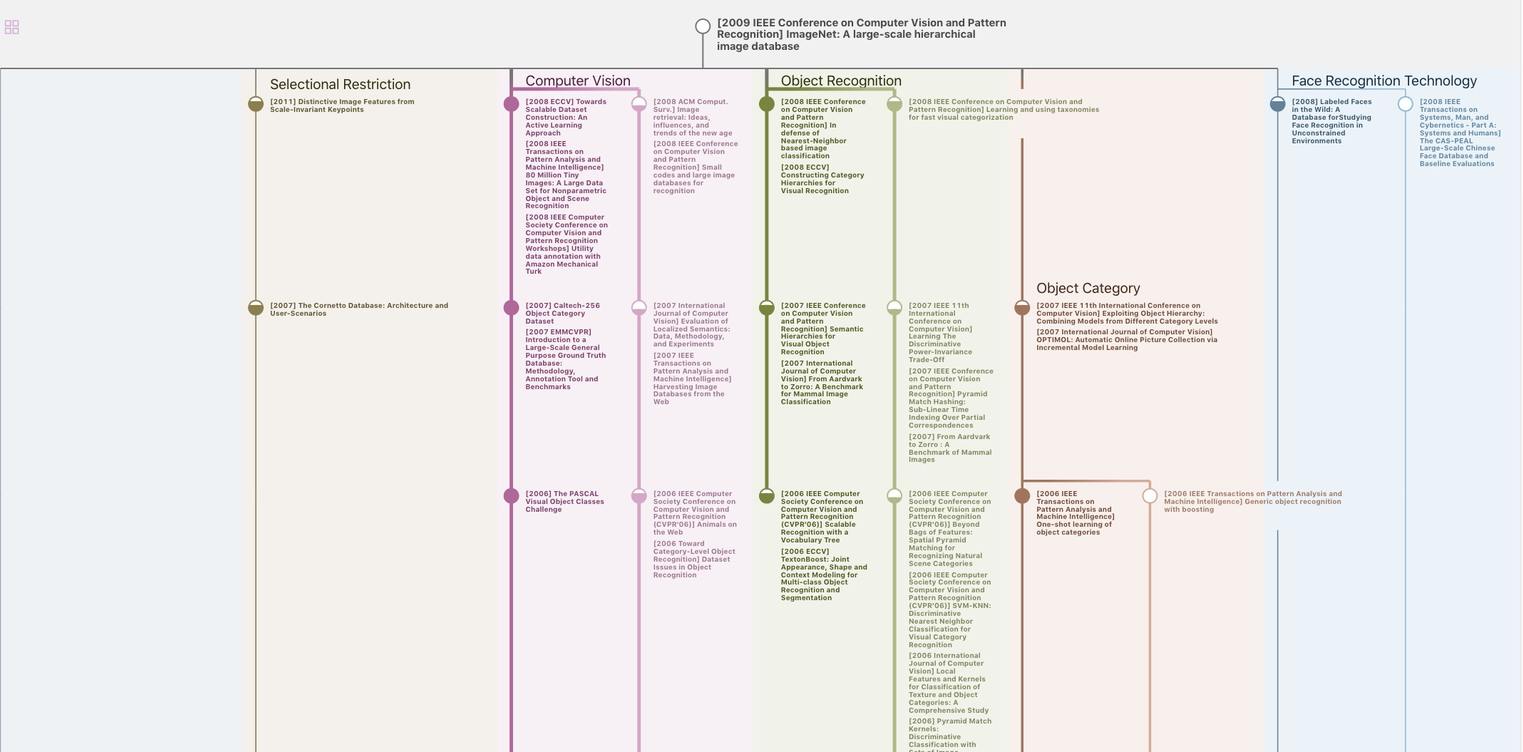
生成溯源树,研究论文发展脉络
Chat Paper
正在生成论文摘要