Bayesian Network Ensemble As A Multivariate Strategy To Predict Radiation Pneumonitis Risk
MEDICAL PHYSICS(2015)
摘要
Purpose: Prediction of radiation pneumonitis (RP) has been shown to be challenging due to the involvement of a variety of factors including dose-volume metrics and radiosensitivity biomarkers. Some of these factors are highly correlated and might affect prediction results when combined. Bayesian network (BN) provides a probabilistic framework to represent variable dependencies in a directed acyclic graph. The aim of this study is to integrate the BN framework and a systems' biology approach to detect possible interactions among RP risk factors and exploit these relationships to enhance both the understanding and prediction of RP.Methods: The authors studied 54 nonsmall-cell lung cancer patients who received curative 3D-conformal radiotherapy. Nineteen RP events were observed (common toxicity criteria for adverse events grade 2 or higher). Serum concentration of the following four candidate biomarkers were measured at baseline and midtreatment: alpha-2-macroglobulin, angiotensin converting enzyme (ACE), transforming growth factor, interleukin-6. Dose-volumetric and clinical parameters were also included as covariates. Feature selection was performed using a Markov blanket approach based on the Koller-Sahami filter. The Markov chain Monte Carlo technique estimated the posterior distribution of BN graphs built from the observed data of the selected variables and causality constraints. RP probability was estimated using a limited number of high posterior graphs (ensemble) and was averaged for the final RP estimate using Bayes' rule. A resampling method based on bootstrapping was applied to model training and validation in order to control under-and overfit pitfalls.Results: RP prediction power of the BN ensemble approach reached its optimum at a size of 200. The optimized performance of the BN model recorded an area under the receiver operating characteristic curve (AUC) of 0.83, which was significantly higher than multivariate logistic regression (0.77), mean heart dose (0.69), and a pre-to-midtreatment change in ACE (0.66). When RP prediction was made only with pretreatment information, the AUC ranged from 0.76 to 0.81 depending on the ensemble size. Bootstrap validation of graph features in the ensemble quantified confidence of association between variables in the graphs where ten interactions were statistically significant.Conclusions: The presented BN methodology provides the flexibility to model hierarchical interactions between RP covariates, which is applied to probabilistic inference on RP. The authors' preliminary results demonstrate that such framework combined with an ensemble method can possibly improve prediction of RP under real-life clinical circumstances such as missing data or treatment plan adaptation. (C) 2015 Author(s).
更多查看译文
关键词
Bayesian network, radiation pneumonitis, NTCP, biomarker, ensemble learning
AI 理解论文
溯源树
样例
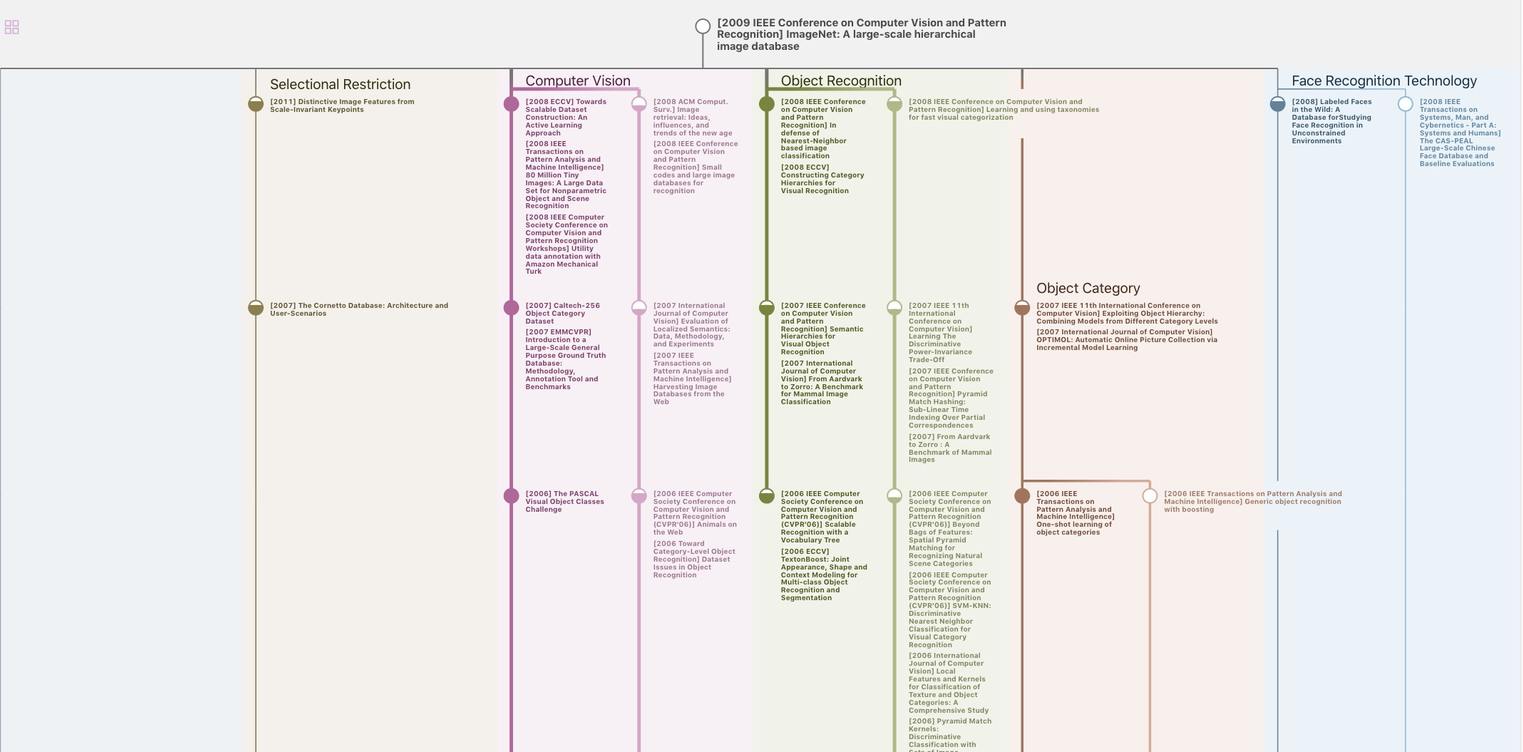
生成溯源树,研究论文发展脉络
Chat Paper
正在生成论文摘要