Tensor-based dictionary learning for dynamic tomographic reconstruction.
PHYSICS IN MEDICINE AND BIOLOGY(2015)
摘要
In dynamic computed tomography (CT) reconstruction, the data acquisition speed limits the spatio-temporal resolution. Recently, compressed sensing theory has been instrumental in improving CT reconstruction from far few-view projections. In this paper, we present an adaptive method to train a tensor-based spatio-temporal dictionary for sparse representation of an image sequence during the reconstruction process. The correlations among atoms and across phases are considered to capture the characteristics of an object. The reconstruction problem is solved by the alternating direction method of multipliers. To recover fine or sharp structures such as edges, the nonlocal total variation is incorporated into the algorithmic framework. Preclinical examples including a sheep lung perfusion study and a dynamic mouse cardiac imaging demonstrate that the proposed approach outperforms the vectorized dictionary-based CT reconstruction in the case of few-view reconstruction.
更多查看译文
关键词
dictionary learning,tensor decomposition,computed tomography,sparse representation
AI 理解论文
溯源树
样例
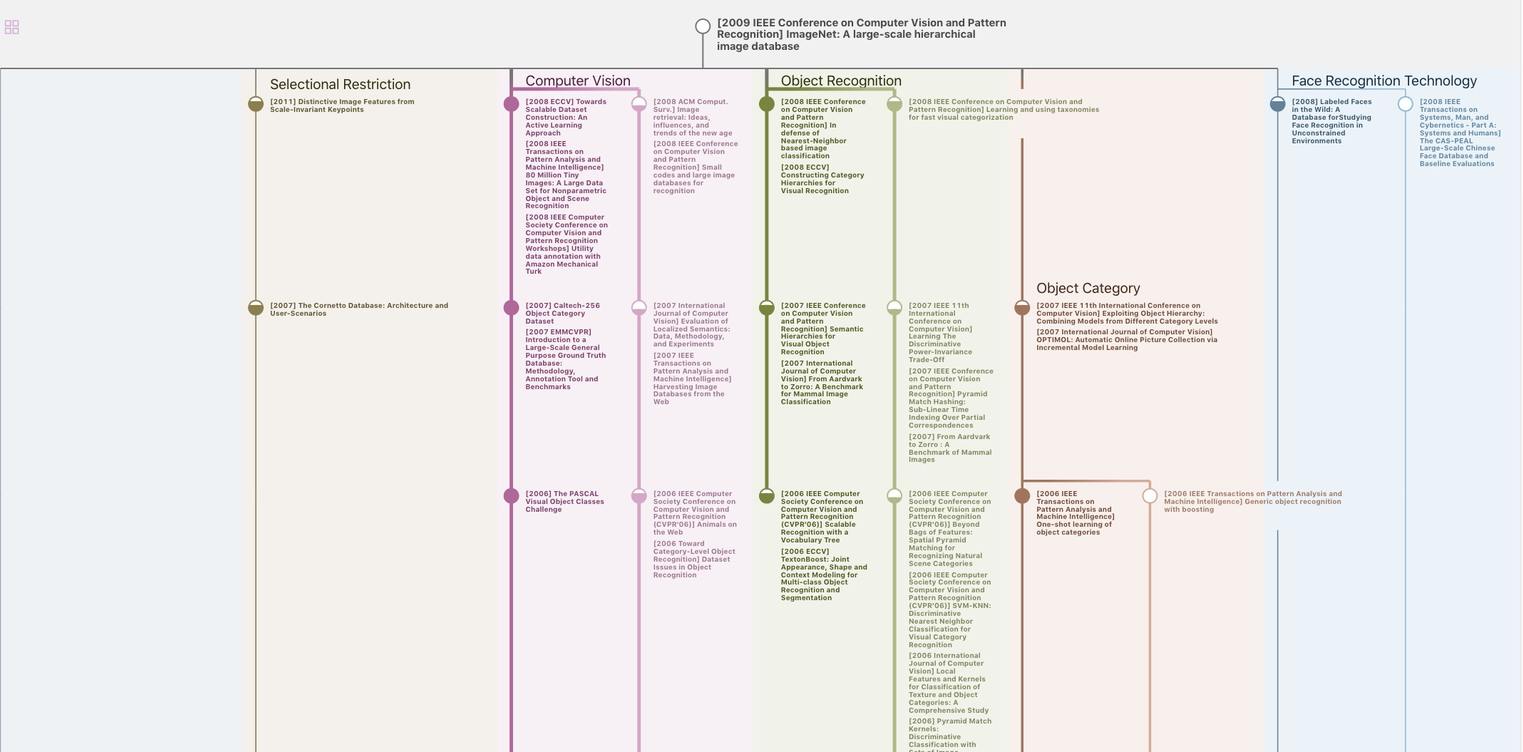
生成溯源树,研究论文发展脉络
Chat Paper
正在生成论文摘要