A Causal Model For Longitudinal Randomised Trials With Time-Dependent Non-Compliance
STATISTICS IN MEDICINE(2015)
摘要
In the presence of non-compliance, conventional analysis by intention-to-treat provides an unbiased comparison of treatment policies but typically under-estimates treatment efficacy. With all-or-nothing compliance, efficacy may be specified as the complier-average causal effect (CACE), where compliers are those who receive intervention if and only if randomised to it. We extend the CACE approach to model longitudinal data with time-dependent non-compliance, focusing on the situation in which those randomised to control may receive treatment and allowing treatment effects to vary arbitrarily over time. Defining compliance type to be the time of surgical intervention if randomised to control, so that compliers are patients who would not have received treatment at all if they had been randomised to control, we construct a causal model for the multivariate outcome conditional on compliance type and randomised arm. This model is applied to the trial of alternative regimens for glue ear treatment evaluating surgical interventions in childhood ear disease, where outcomes are measured over five time points, and receipt of surgical intervention in the control arm may occur at any time. We fit the models using Markov chain Monte Carlo methods to obtain estimates of the CACE at successive times after receiving the intervention. In this trial, over a half of those randomised to control eventually receive intervention. We find that surgery is more beneficial than control at 6months, with a small but non-significant beneficial effect at 12months. (c) 2015 The Authors. Statistics in Medicine Published by JohnWiley & Sons Ltd.
更多查看译文
关键词
non-compliance, intention to treat, complier average causal effect, longitudinal model
AI 理解论文
溯源树
样例
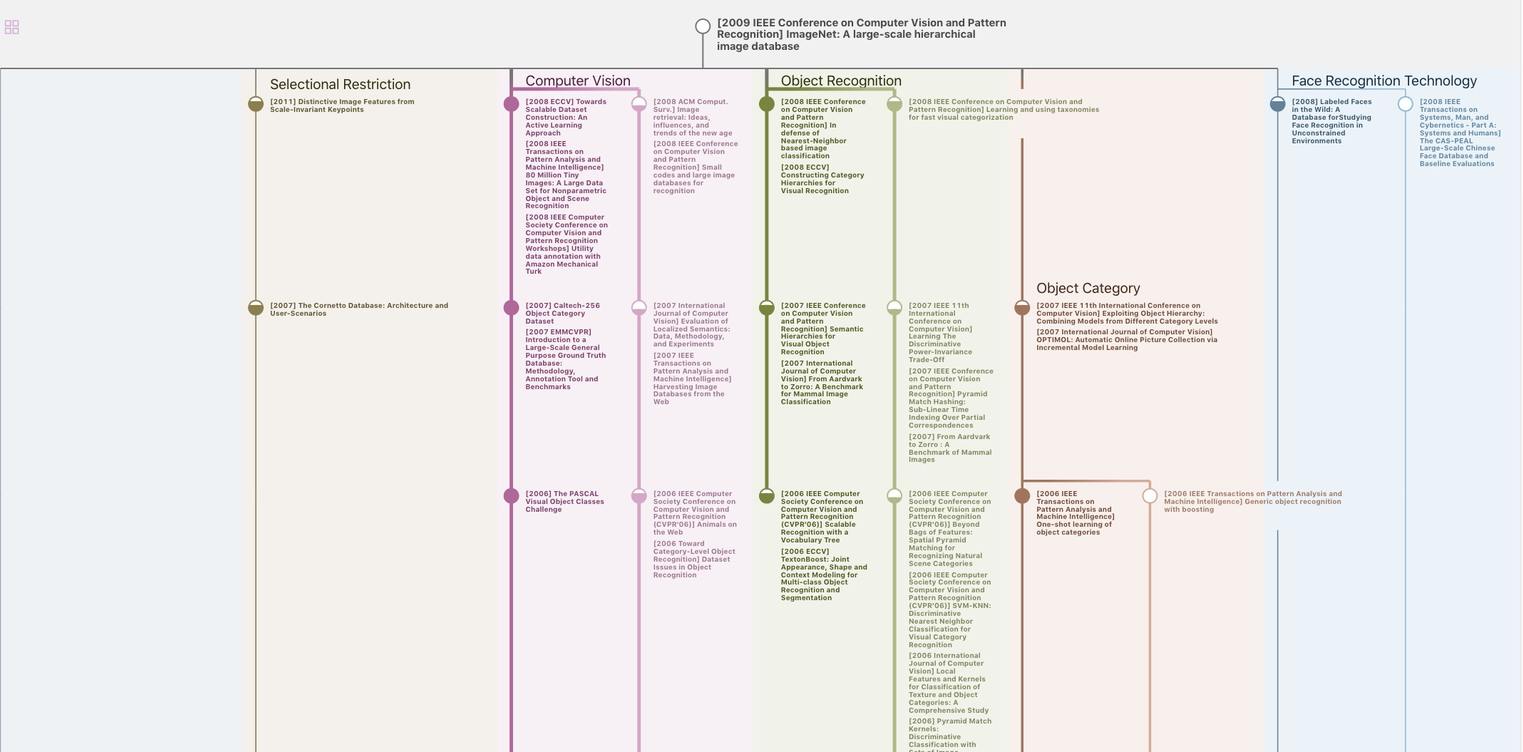
生成溯源树,研究论文发展脉络
Chat Paper
正在生成论文摘要