Dimensionality reduction of RKHS model parameters.
ISA Transactions(2015)
摘要
This paper proposes a new method to reduce the parameter number of models developed in the Reproducing Kernel Hilbert Space (RKHS). In fact, this number is equal to the number of observations used in the learning phase which is assumed to be high. The proposed method entitled Reduced Kernel Partial Least Square (RKPLS) consists on approximating the retained latent components determined using the Kernel Partial Least Square (KPLS) method by their closest observation vectors. The paper proposes the design and the comparative study of the proposed RKPLS method and the Support Vector Machines on Regression (SVR) technique. The proposed method is applied to identify a nonlinear Process Trainer PT326 which is a physical process available in our laboratory. Moreover as a thermal process with large time response may help record easily effective observations which contribute to model identification. Compared to the SVR technique, the results from the proposed RKPLS method are satisfactory.
更多查看译文
关键词
RKHS,System identification,Kernel method,KPLS,RKPLS,Process Trainer
AI 理解论文
溯源树
样例
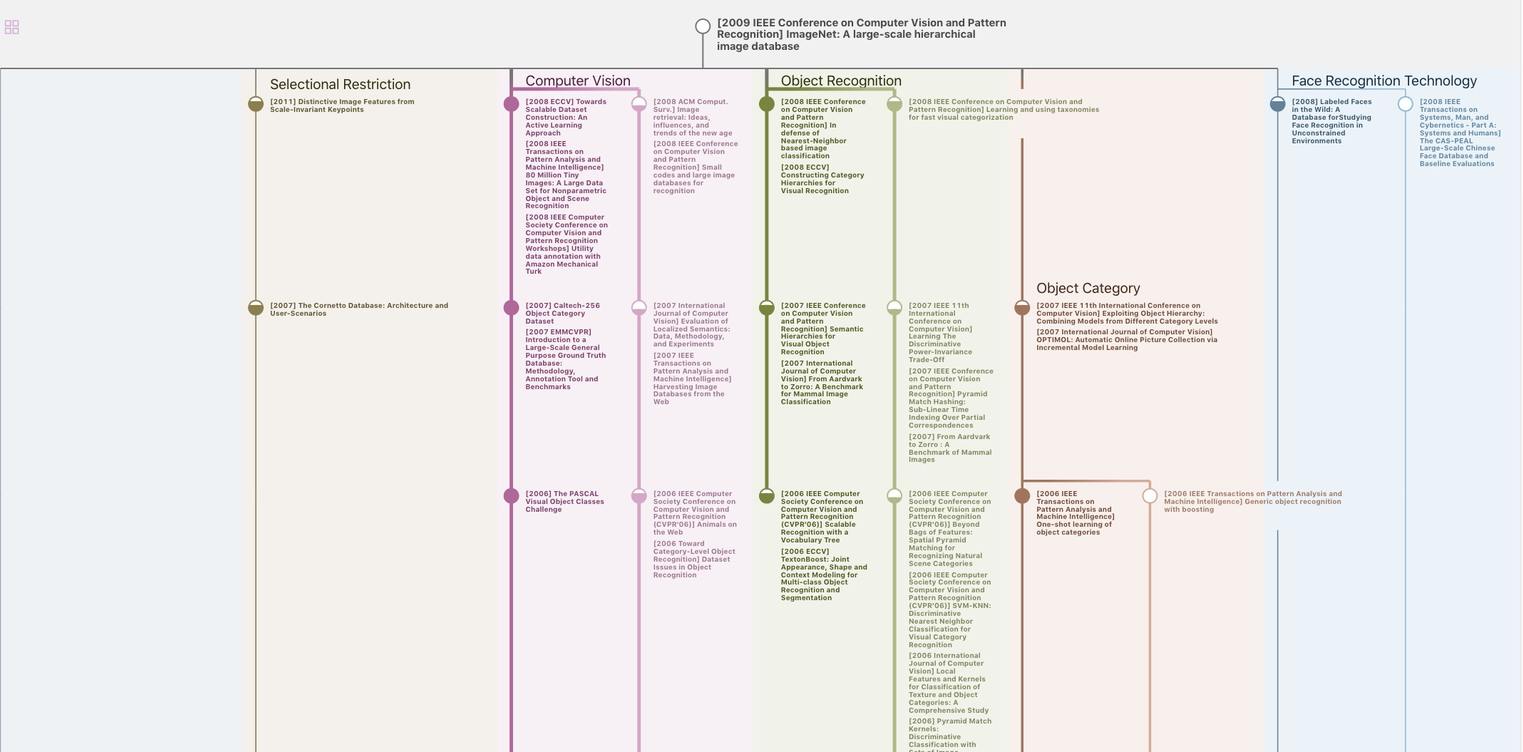
生成溯源树,研究论文发展脉络
Chat Paper
正在生成论文摘要