Rare disease registries classification and characterization: a data mining approach.
PUBLIC HEALTH GENOMICS(2015)
摘要
Background: The European Commission and Patients Organizations identify rare disease registries (RDRs) as strategic instruments to develop research and improve knowledge in the field of rare diseases. Interoperability between RDRs is needed for research activities, validation of therapeutic treatments, and public health actions. Sharing and comparing information requires a uniform and standardized way of data collection, so levels of interconnection between RDRs with similar aims and/or nature of data should be identified. The objective of this study is to define a classification and characterization of RDRs in order to identify different profiles and informative needs. Methods: Exploratory statistical analyses (cluster analysis and random forest) were applied to data derived from the EPIRARE project ('Building Consensus and Synergies for the EU Rare Disease Patient Registration') survey on the activities and needs of RDRs. Results: The cluster analysis identified 3 main typologies of RDRs: public health, clinical and genetic research, and treatment registries. The analysis of the most informative variables, identified by the random forest method, led to the characterization of 3 types of RDRs and the definition of different profiles and informative needs. Conclusions: These results represent a useful source of information to facilitate the harmonization and interconnection of RDRs in accordance with the different profiles identified. It could help sharing the information between RDRs with similar profiles and, whenever possible, interconnections between registries with different profiles. (C) 2015 S. Karger AG, Basel
更多查看译文
关键词
Cluster analysis,Data mining,Random forest,Rare disease registries
AI 理解论文
溯源树
样例
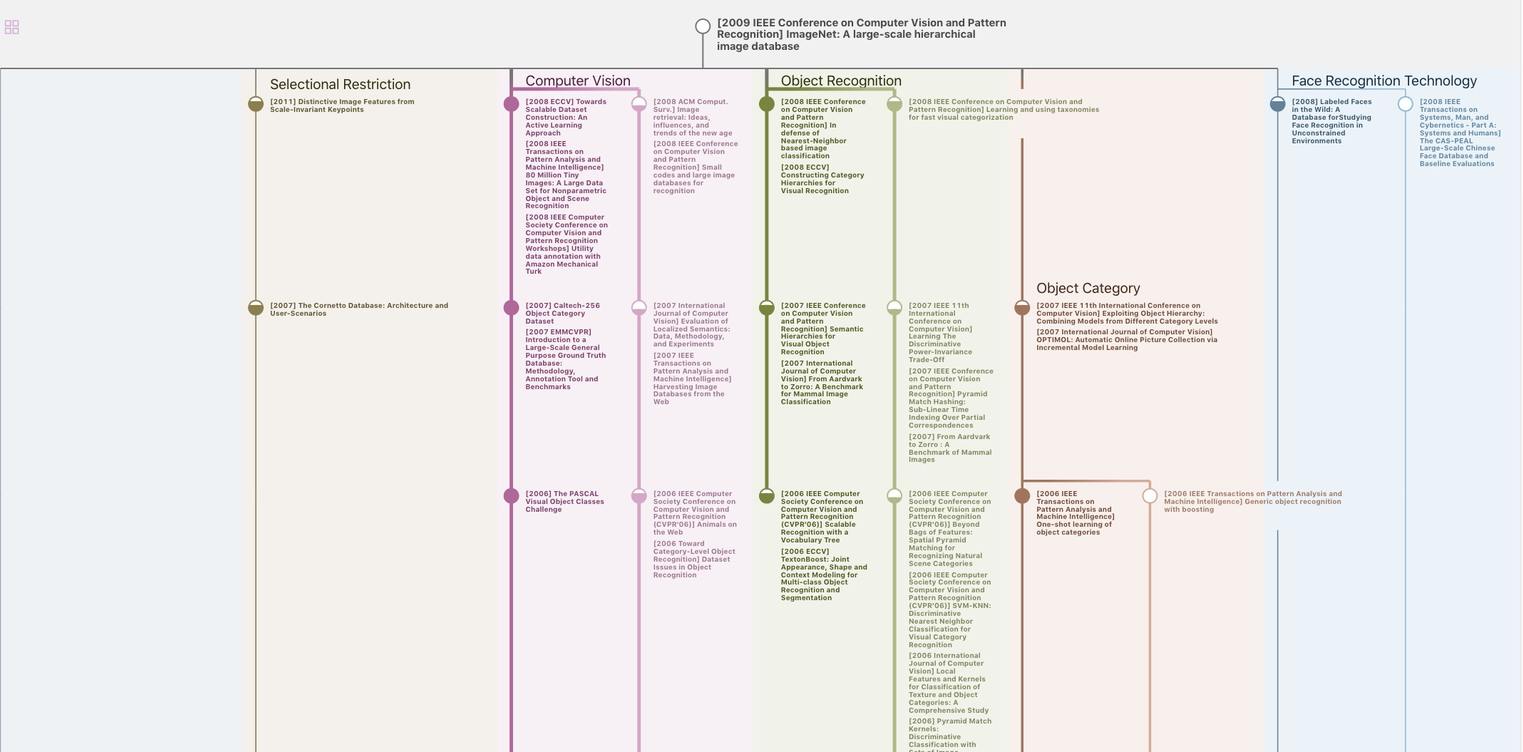
生成溯源树,研究论文发展脉络
Chat Paper
正在生成论文摘要