A hybrid feature selection approach for the early diagnosis of Alzheimer's disease.
JOURNAL OF NEURAL ENGINEERING(2015)
摘要
Objective. Recently, significant advances have been made in the early diagnosis of Alzheimer's disease (AD) from electroencephalography (EEG). However, choosing suitable measures is a challenging task. Among other measures, frequency relative power (RP) and loss of complexity have been used with promising results. In the present study we investigate the early diagnosis of AD using synchrony measures and frequency RP on EEG signals, examining the changes found in different frequency ranges. Approach. We first explore the use of a single feature for computing the classification rate (CR), looking for the best frequency range. Then, we present a multiple feature classification system that outperforms all previous results using a feature selection strategy. These two approaches are tested in two different databases, one containing mild cognitive impairment (MCI) and healthy subjects (patients age: 71.9 +/- 10.2, healthy subjects age: 71.7 +/- 8.3), and the other containing Mild AD and healthy subjects (patients age: 77.6 +/- 10.0; healthy subjects age: 69.4 +/- 11.5). Main results. Using a single feature to compute CRs we achieve a performance of 78.33% for the MCI data set and of 97.56% for Mild AD. Results are clearly improved using the multiple feature classification, where a CR of 95% is found for the MCI data set using 11 features, and 100% for the Mild AD data set using four features. Significance. The new features selection method described in this work may be a reliable tool that could help to design a realistic system that does not require prior knowledge of a patient's status. With that aim, we explore the standardization of features for MCI and Mild AD data sets with promising results.
更多查看译文
关键词
Alzheimer's disease,mild cognitive impairment,electroencephalography,synchrony,relative power,Granger causality,Gram-Schmidt orthogonal forward regression
AI 理解论文
溯源树
样例
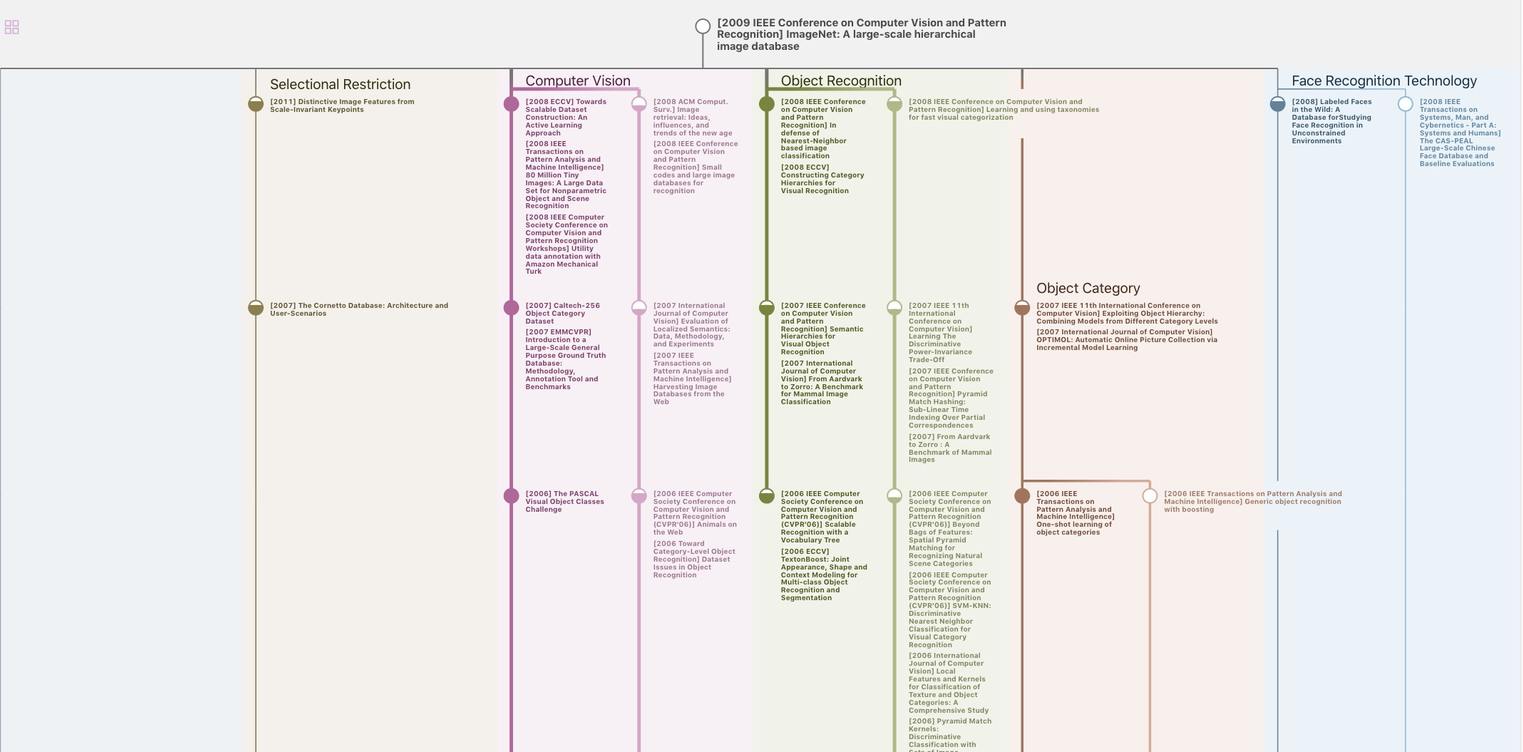
生成溯源树,研究论文发展脉络
Chat Paper
正在生成论文摘要