Active Semi-Supervised Community Detection Based On Must-Link And Cannot-Link Constraints
PLOS ONE(2014)
摘要
Community structure detection is of great importance because it can help in discovering the relationship between the function and the topology structure of a network. Many community detection algorithms have been proposed, but how to incorporate the prior knowledge in the detection process remains a challenging problem. In this paper, we propose a semi-supervised community detection algorithm, which makes full utilization of the must-link and cannot-link constraints to guide the process of community detection and thereby extracts high-quality community structures from networks. To acquire the high-quality must-link and cannot-link constraints, we also propose a semi-supervised component generation algorithm based on active learning, which actively selects nodes with maximum utility for the proposed semi-supervised community detection algorithm step by step, and then generates the must-link and cannot-link constraints by accessing a noiseless oracle. Extensive experiments were carried out, and the experimental results show that the introduction of active learning into the problem of community detection makes a success. Our proposed method can extract high-quality community structures from networks, and significantly outperforms other comparison methods.
更多查看译文
关键词
algorithms,internet
AI 理解论文
溯源树
样例
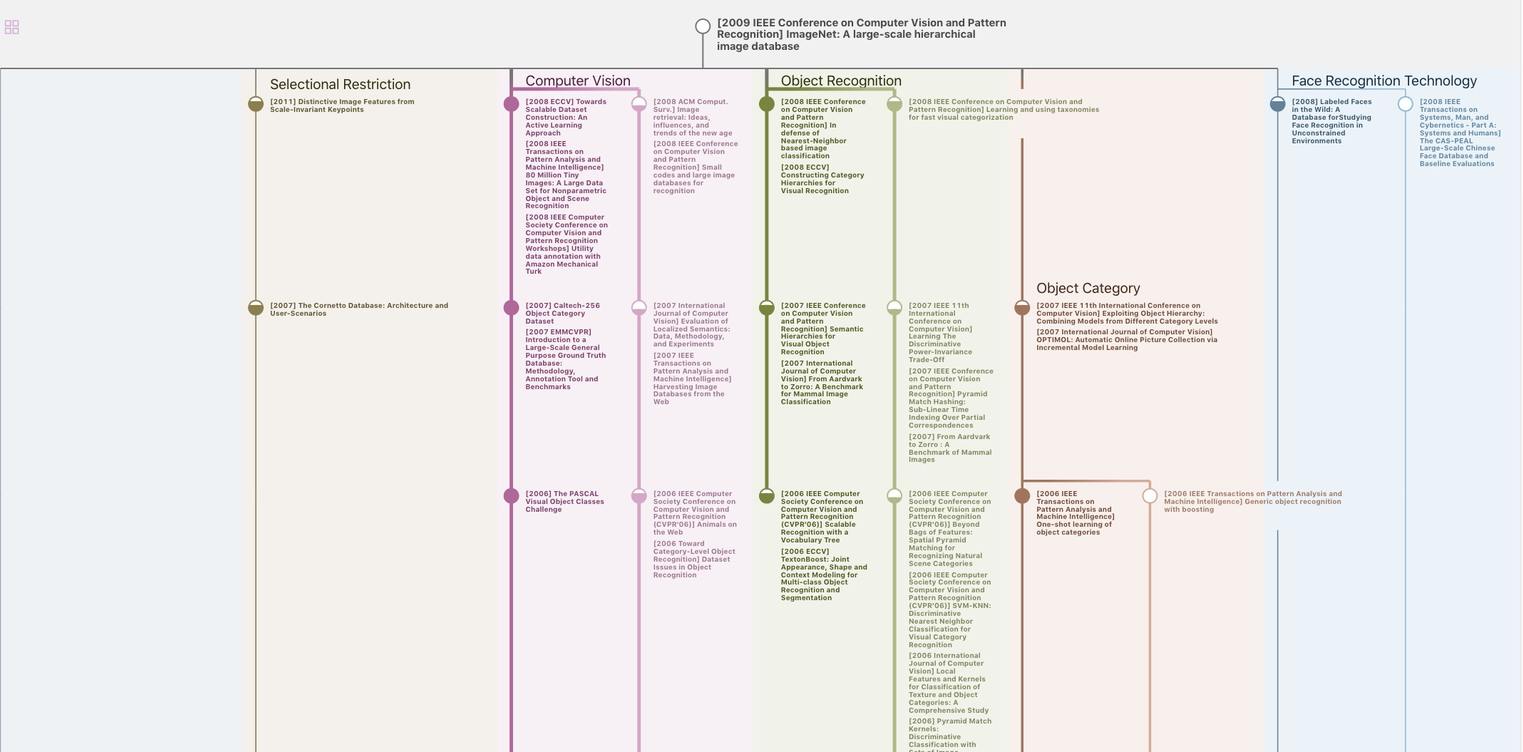
生成溯源树,研究论文发展脉络
Chat Paper
正在生成论文摘要