What is Semantic Distance? A Review and Proposed Method for Modeling Conceptual Transitions in Natural Language
crossref(2022)
摘要
Cognitive science has seen a rapid evolution of the tools available for studying conceptual knowledge. Historically, much of our understanding of semantic memory has been informed through studies using language. Natural language processing (NLP) has offered groundbreaking techniques for elucidating relationships between concepts and language at unprecedented scales. One of the most popular applications has involved mathematically representing concepts using high dimensional feature spaces. Here we describe the nature of such semantic spaces and review ways in which human- and machine-generated semantic distance metrics differ in capturing taxonomic (e.g., dog-wolf) versus thematic (e.g., dog-leash) semantic relationships. We propose a novel method and open-source algorithm for deriving semantic distances between adjacent content words in connected language samples. This R package transforms a user-specified language transcript into a vector of pairwise semantic distances spanning all adjacent bigrams (e.g., The cat drank the milk → cat-drink, drink-milk, etc.). These distances constitute a continuous time series reflecting word-by-word level changes in meaning across a language sample of any length. We derive semantic distance norms and apply the proposed technique to a classic work of short fiction, To Build a Fire (Jack London, 1908). We discuss extensions of this time series approach, including the potential for forecasting, causal modeling, topic cohesion, and as an implicit measure of semantic impairment in spoken and/or written narratives.
更多查看译文
AI 理解论文
溯源树
样例
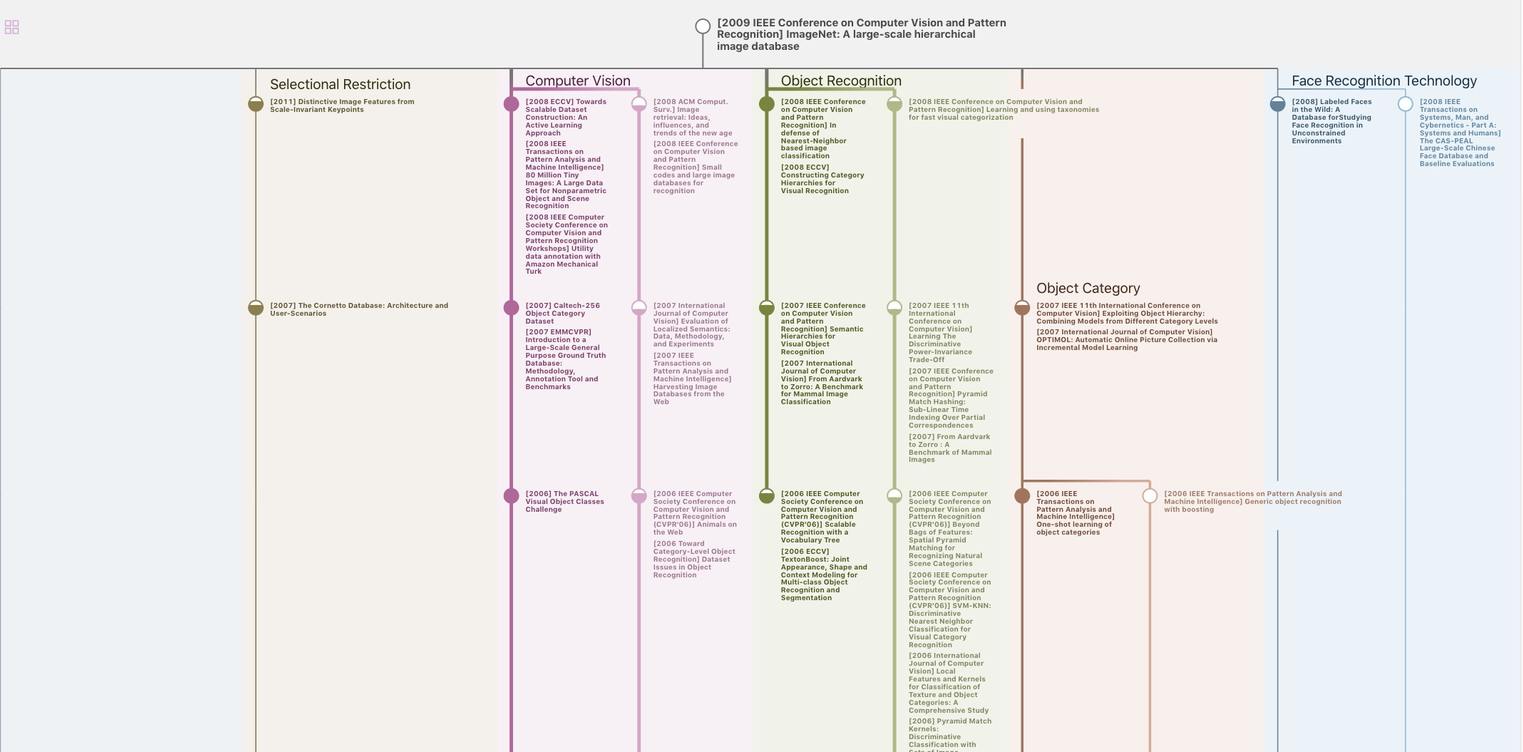
生成溯源树,研究论文发展脉络
Chat Paper
正在生成论文摘要