Alternative Approach To Chemical Accuracy: A Neural Networks-Based First-Principles Method For Heat Of Formation Of Molecules Made Of H, C, N, O F, S, And Cl
JOURNAL OF PHYSICAL CHEMISTRY A(2014)
摘要
The neural network correction approach that was previously proposed to achieve the chemical accuracy for first-principles methods is further developed by a combination of the Kennard-Stone sampling and Bootstrapping methods. As a result, the accuracy of the calculated heat of formation is improved further, and moreover, the error bar of each calculated result can be determined. An enlarged database (Chen/13), which contains a total of 539 molecules made of the common elements H, C, N, O, F, S, and Cl, is constructed and is divided into the training (449 molecules) and testing (90 molecules) data sets with the Kennard-Stone sampling method. Upon the neural network correction, the mean absolute deviation (MAD) of the B3LYP/6-311+G(3df,2p) calculated heat of formation is reduced from 10.92 to 1.47 kcal mol(-1) and 14.95 to 1.31 kcal mol(-1) for the training and testing data sets, respectively. Furthermore, the Bootstrapping method, a broadly used statistical method, is employed to assess the accuracy of each neural-network prediction by determining its error bar. The average error bar for the testing data set is 1.05 kcal mol(-1), therefore achieving the chemical accuracy. When a testing molecule falls into the regions of the "Chemical the Space" where the distribution density of the training molecules is high, its predicted error bar is comparatively small, and thus, among the existing experimental data.
更多查看译文
关键词
Computational Chemistry,Molecular Docking,Materials Discovery,Standard Molar Enthalpies,Calorimetry
AI 理解论文
溯源树
样例
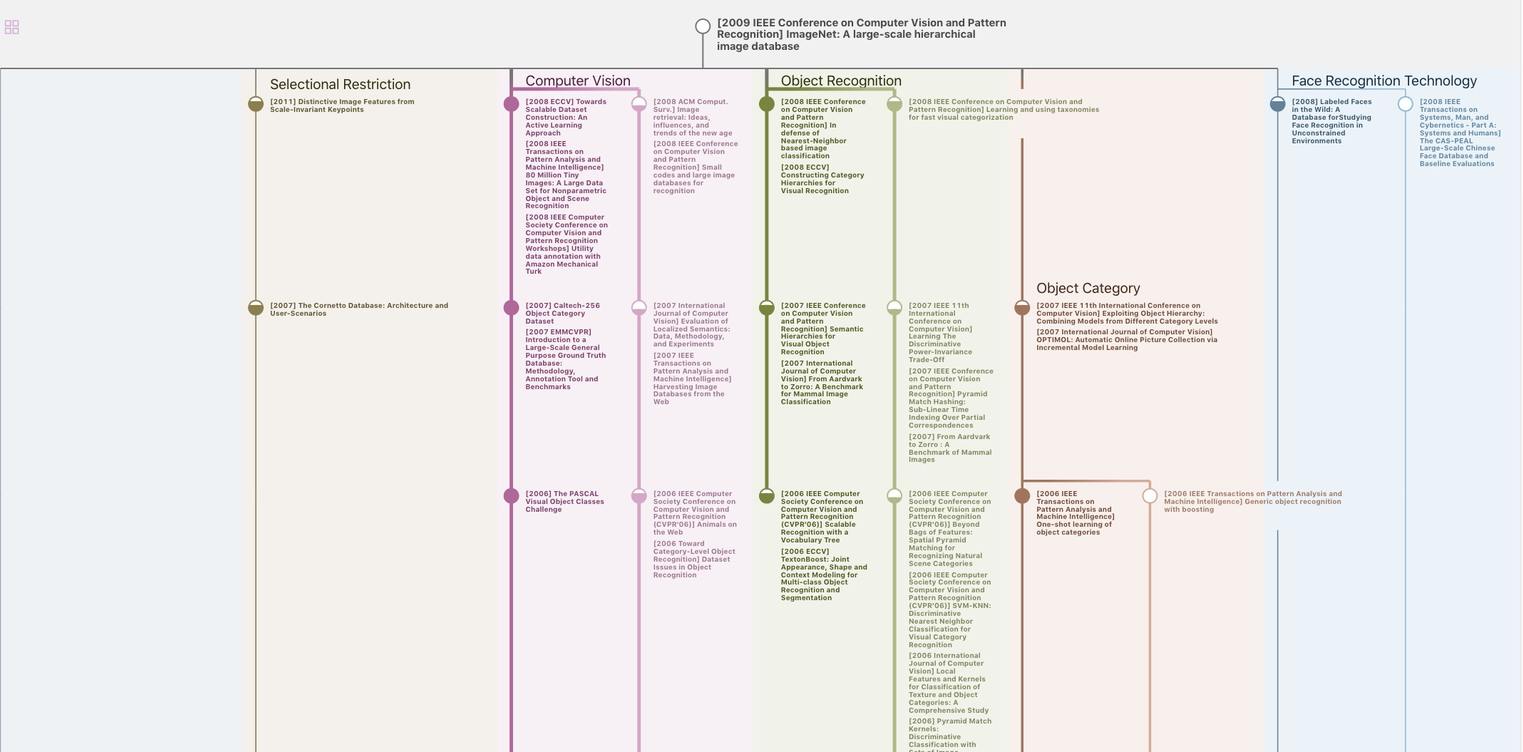
生成溯源树,研究论文发展脉络
Chat Paper
正在生成论文摘要