A Comparison of Weight Initializers in Deep Learning-Based Side-Channel Analysis.
ACNS Workshops(2020)
摘要
The usage of deep learning in profiled side-channel analysis requires a careful selection of neural network hyperparameters. In recent publications, different network architectures have been presented as efficient profiled methods against protected AES implementations. Indeed, completely different convolutional neural network models have presented similar performance against public side-channel traces databases. In this work, we analyze how weight initializers’ choice influences deep neural networks’ performance in the profiled side-channel analysis. Our results show that different weight initializers provide radically different behavior. We observe that even high-performing initializers can reach significantly different performance when conducting multiple training phases. Finally, we found that this hyperparameter is more dependent on the choice of dataset than other, commonly examined, hyperparameters. When evaluating the connections with other hyperparameters, the biggest connection is observed with activation functions.
更多查看译文
关键词
weight initializers,learning-based,side-channel
AI 理解论文
溯源树
样例
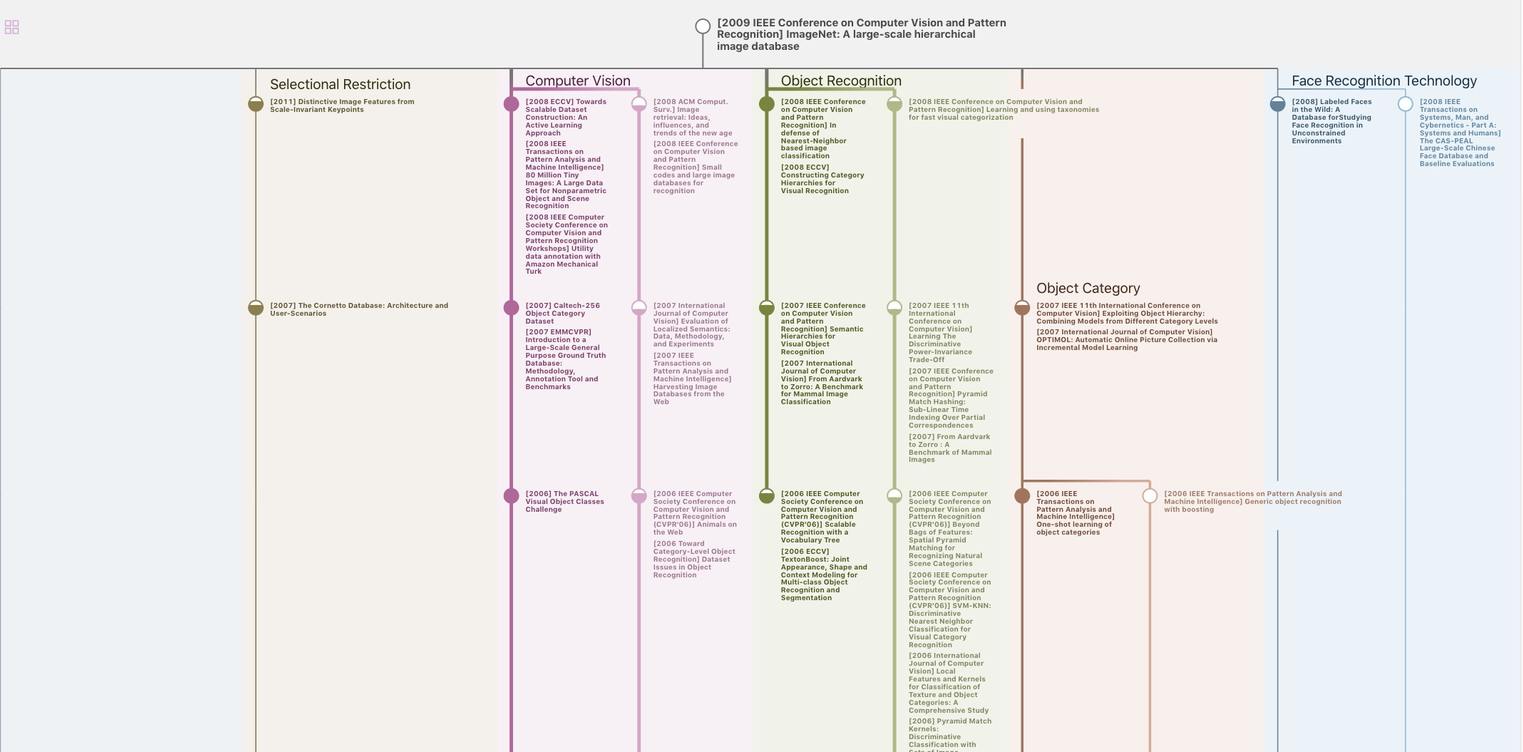
生成溯源树,研究论文发展脉络
Chat Paper
正在生成论文摘要