Sparsity-regularized image reconstruction of decomposed K-edge data in spectral CT.
PHYSICS IN MEDICINE AND BIOLOGY(2014)
摘要
The development of spectral computed tomography (CT) using binned photon-counting detectors has garnered great interest in recent years and has enabled selective imaging of K-edge materials. A practical challenge in CT image reconstruction of K-edge materials is the mitigation of image artifacts that arise from reduced-view and/or noisy decomposed sinogram data. In this note, we describe and investigate sparsity-regularized penalized weighted least squares-based image reconstruction algorithms for reconstructing K-edge images from few-view decomposed K-edge sinogram data. To exploit the inherent sparseness of typical K-edge images, we investigate use of a total variation (TV) penalty and a weighted sum of a TV penalty and an l(1)-norm with a wavelet sparsifying transform. Computer-simulation and experimental phantom studies are conducted to quantitatively demonstrate the effectiveness of the proposed reconstruction algorithms.
更多查看译文
关键词
computed tomography (CT),energy-resolved CT,K-edge imaging,material-decomposition,statistical image reconstruction,total variation regularization
AI 理解论文
溯源树
样例
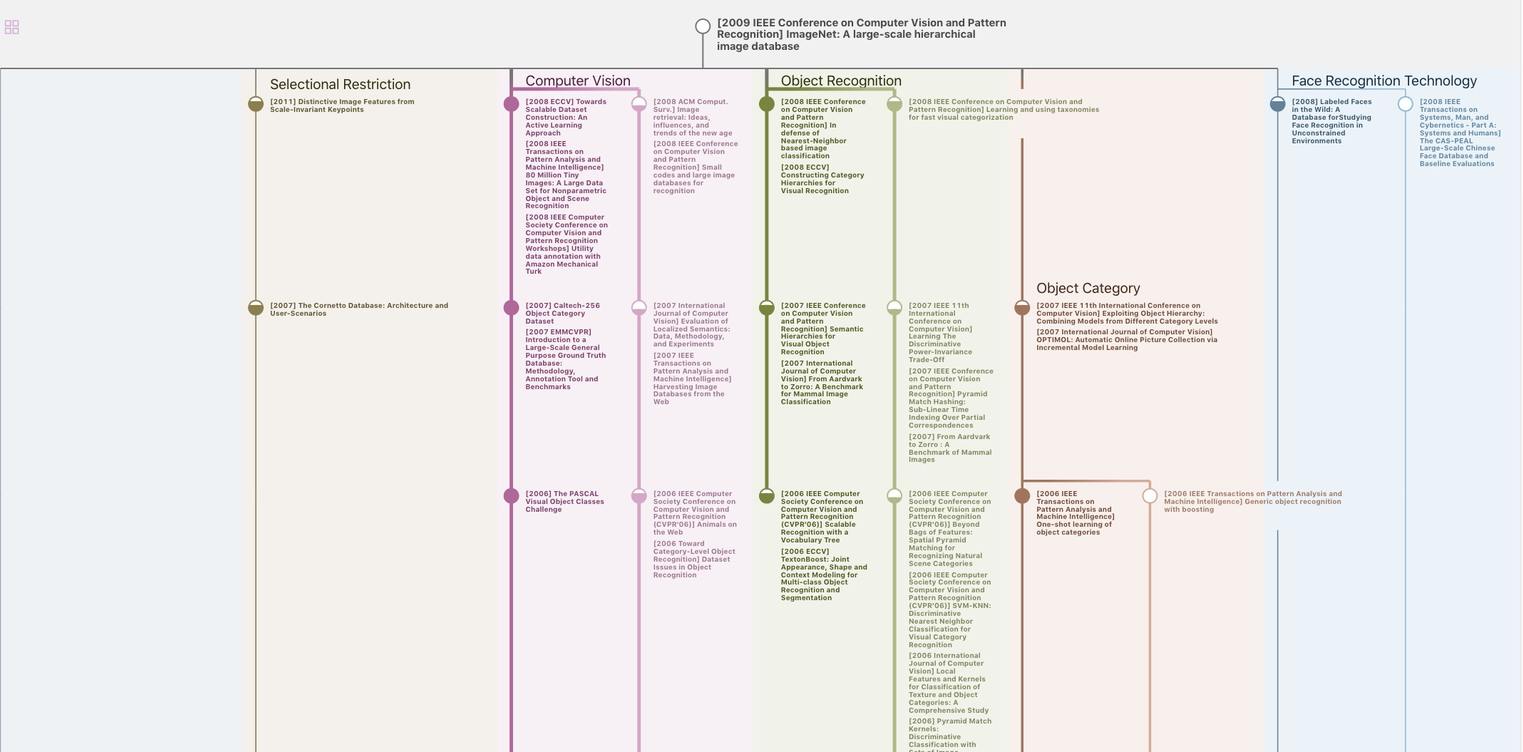
生成溯源树,研究论文发展脉络
Chat Paper
正在生成论文摘要