Recidivism And Rehabilitation Of Criminal Offenders: A Carrot And Stick Evolutionary Game
PLOS ONE(2014)
摘要
Motivated by recent efforts by the criminal justice system to treat and rehabilitate nonviolent offenders rather than focusing solely on their punishment, we introduce an evolutionary game theoretic model to study the effects of "carrot and stick" intervention programs on criminal recidivism. We use stochastic simulations to study the evolution of a population where individuals may commit crimes depending on their past history, surrounding environment and, in the case of recidivists, on any counseling, educational or training programs available to them after being punished for their previous crimes. These sociological factors are embodied by effective parameters that determine the decision making probabilities. Players may decide to permanently reform or continue engaging in criminal activity, eventually reaching a state where they are considered incorrigible. Depending on parameter choices, the outcome of the game is a society with a majority of virtuous, rehabilitated citizens or incorrigibles. Since total resources may be limited, we constrain the combined punishment and rehabilitation costs per crime to be fixed, so that increasing one effort will necessarily decrease the other. We find that the most successful strategy in reducing crime is to optimally allocate resources so that after being punished, criminals experience impactful intervention programs, especially during the first stages of their return to society. Excessively harsh or lenient punishments are less effective. We also develop a system of coupled ordinary differential equations with memory effects to give a qualitative description of our simulated societal dynamics. We discuss our findings and sociological implications.
更多查看译文
关键词
biology,algorithms,stochastic processes,monte carlo method,population dynamics,chemistry,game theory,medicine,computer simulation,engineering,physics
AI 理解论文
溯源树
样例
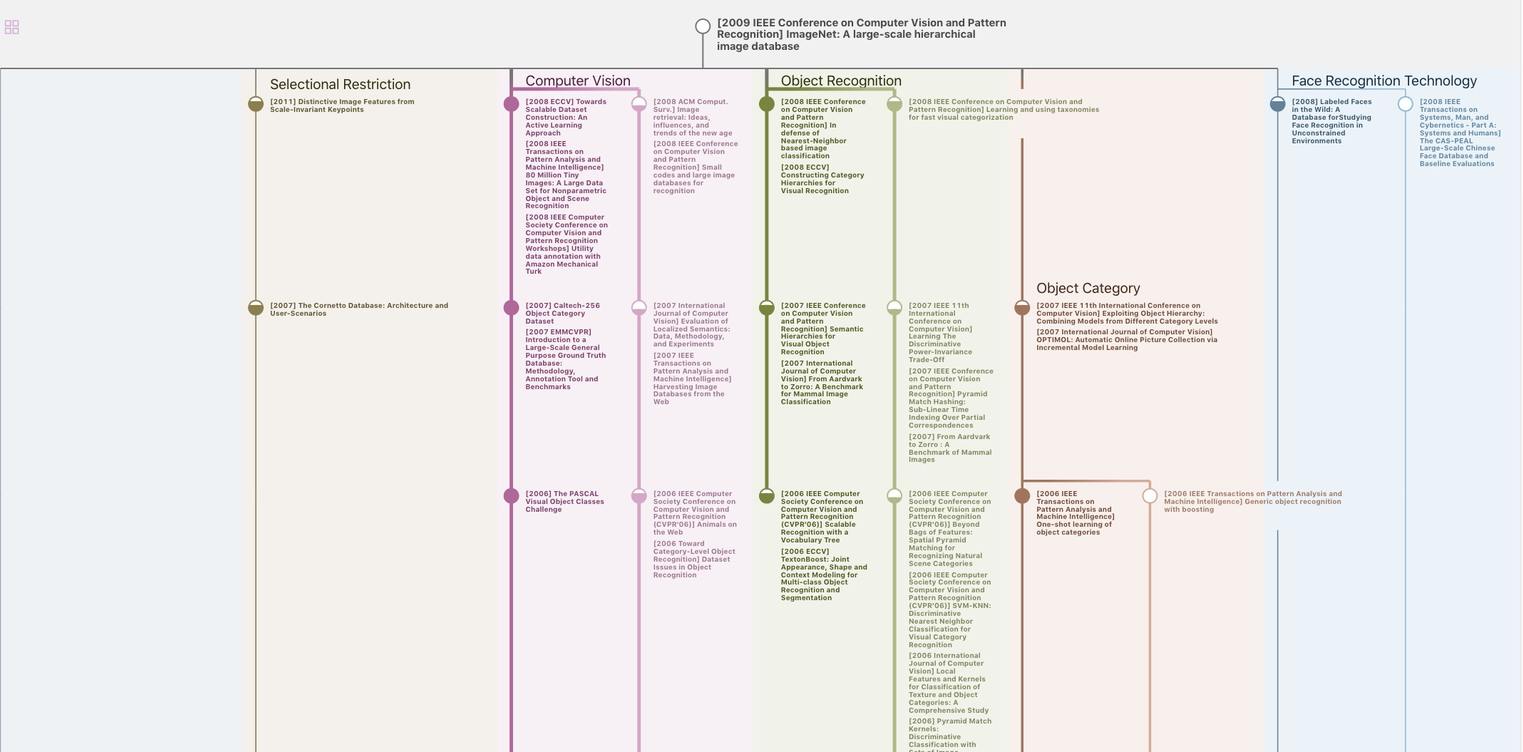
生成溯源树,研究论文发展脉络
Chat Paper
正在生成论文摘要