A Neural Network-Based Spectral Approach for the Assignment of Individual Trees to Genetically Differentiated Subpopulations
REMOTE SENSING(2022)
摘要
Studying population structure has made an essential contribution to understanding evolutionary processes and demographic history in forest ecology research. This inference process basically involves the identification of common genetic variants among individuals, then grouping the similar individuals into subpopulations. In this study, a spectral-based classification of genetically differentiated groups was carried out using a provenance-progeny trial of Eucalyptus cladocalyx. First, the genetic structure was inferred through a Bayesian analysis using single-nucleotide polymorphisms (SNPs). Then, different machine learning models were trained with foliar spectral information to assign individual trees to subpopulations. The results revealed that spectral-based classification using the multilayer perceptron method was very successful at classifying individuals into their respective subpopulations (with an average of 87% of correct individual assignments), whereas 85% and 81% of individuals were assigned to their respective classes correctly by convolutional neural network and partial least squares discriminant analysis, respectively. Notably, 93% of individual trees were assigned correctly to the class with the smallest size using the spectral data-based multi-layer perceptron classification method. In conclusion, spectral data, along with neural network models, are able to discriminate and assign individuals to a given subpopulation, which could facilitate the implementation and application of population structure studies on a large scale.
更多查看译文
关键词
convolutional neural network, multilayer perceptron, population genetic structure, remote sensing classification, sugar gum
AI 理解论文
溯源树
样例
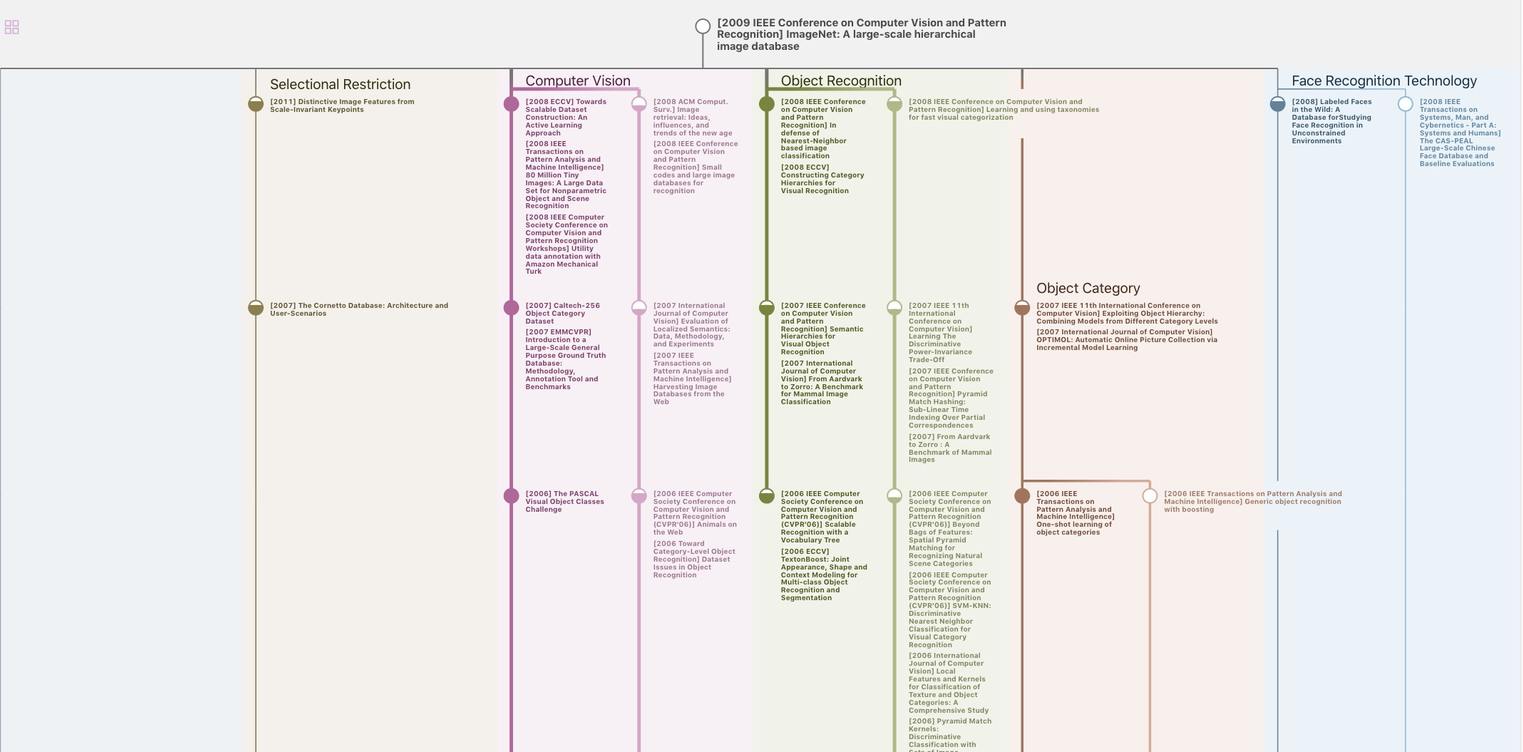
生成溯源树,研究论文发展脉络
Chat Paper
正在生成论文摘要