How humans learn and represent networks.
Proceedings of the National Academy of Sciences of the United States of America(2020)
摘要
Humans receive information from the world around them in sequences of discrete items-from words in language or notes in music to abstract concepts in books and websites on the Internet. To model their environment, from a young age people are tasked with learning the network structures formed by these items (nodes) and the connections between them (edges). But how do humans uncover the large-scale structures of networks when they experience only sequences of individual items? Moreover, what do people's internal maps and models of these networks look like? Here, we introduce graph learning, a growing and interdisciplinary field studying how humans learn and represent networks in the world around them. Specifically, we review progress toward understanding how people uncover the complex webs of relationships underlying sequences of items. We begin by describing established results showing that humans can detect fine-scale network structure, such as variations in the probabilities of transitions between items. We next present recent experiments that directly control for differences in transition probabilities, demonstrating that human behavior depends critically on the mesoscale and macroscale properties of networks. Finally, we introduce computational models of human graph learning that make testable predictions about the impact of network structure on people's behavior and cognition. Throughout, we highlight open questions in the study of graph learning that will require creative insights from cognitive scientists and network scientists alike.
更多查看译文
AI 理解论文
溯源树
样例
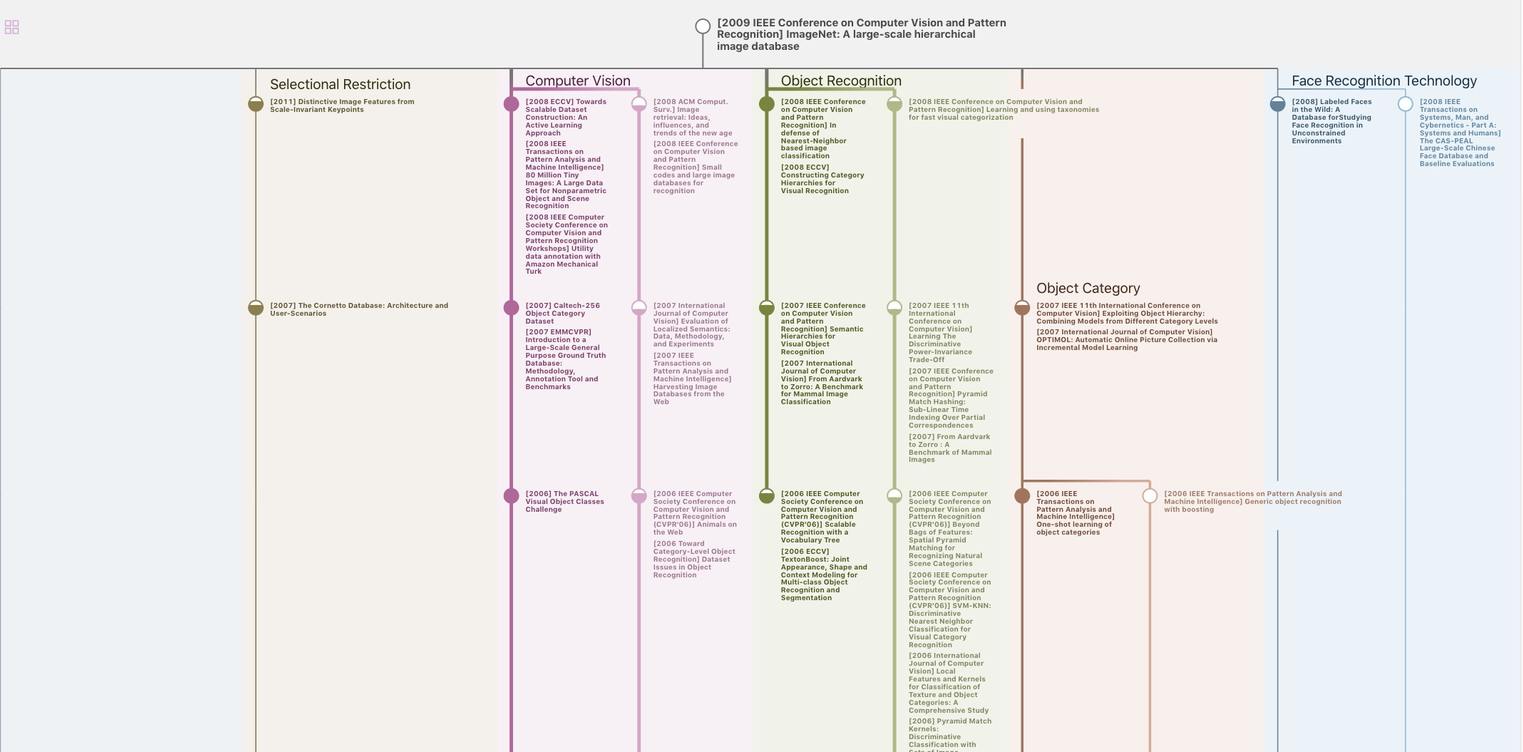
生成溯源树,研究论文发展脉络
Chat Paper
正在生成论文摘要