Feature Selection For Classification Based On Fine Motor Signs Of Parkinson'S Disease
2009 ANNUAL INTERNATIONAL CONFERENCE OF THE IEEE ENGINEERING IN MEDICINE AND BIOLOGY SOCIETY, VOLS 1-20(2009)
摘要
Effective evaluation of potential neuroprotective interventions for Parkinson's disease (PD) requires precise quantification of the motor signs associated with this disease. We have created a protocol that uses force tracking in a simultaneous task paradigm to quantify the tine motor control deficits in individuals with PD. We have used this protocol to collect data from 30 individuals with early to moderate PD and 30 age-matched controls. Based on this data, we computed 60 variables. We generated all possible combinations of three of these variables, and we then computed the classification accuracy of a support vector machine (SVM) trained on each variable combination. We were able to correctly classify 85% of subjects as with or without PD. We found that root-mean-square error variables were the most important features for classification and that utilizing a simultaneous task paradigm improves classification accuracy.
更多查看译文
关键词
image classification,feature selection,feature extraction,protocols,support vector machines,support vector machine,neurophysiology,root mean square error,motor control
AI 理解论文
溯源树
样例
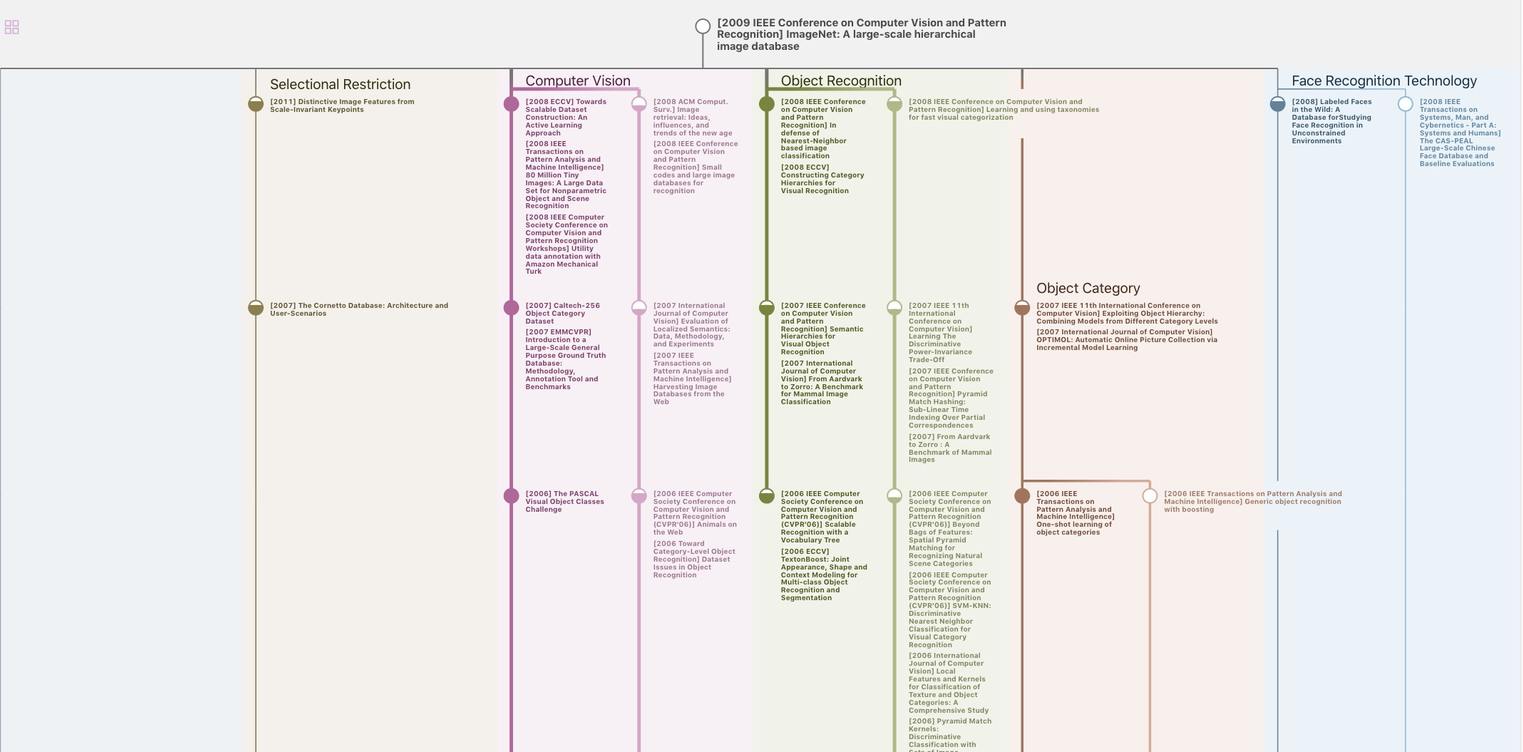
生成溯源树,研究论文发展脉络
Chat Paper
正在生成论文摘要