Predictive-property-ranked variable reduction in partial least squares modelling with final complexity adapted models: comparison of properties for ranking.
Analytica Chimica Acta(2013)
摘要
The calibration performance of partial least squares regression for one response (PLS1) can be improved by eliminating uninformative variables. Many variable-reduction methods are based on so-called predictor-variable properties or predictive properties, which are functions of various PLS-model parameters, and which may change during the steps of the variable-reduction process. Recently, a new predictive-property-ranked variable reduction method with final complexity adapted models, denoted as PPRVR-FCAM or simply FCAM, was introduced. It is a backward variable elimination method applied on the predictive-property-ranked variables. The variable number is first reduced, with constant PLS1 model complexity A, until A variables remain, followed by a further decrease in PLS complexity, allowing the final selection of small numbers of variables.
更多查看译文
关键词
Variable reduction,Partial least squares,Predictor-variable properties,Final complexity adapted models
AI 理解论文
溯源树
样例
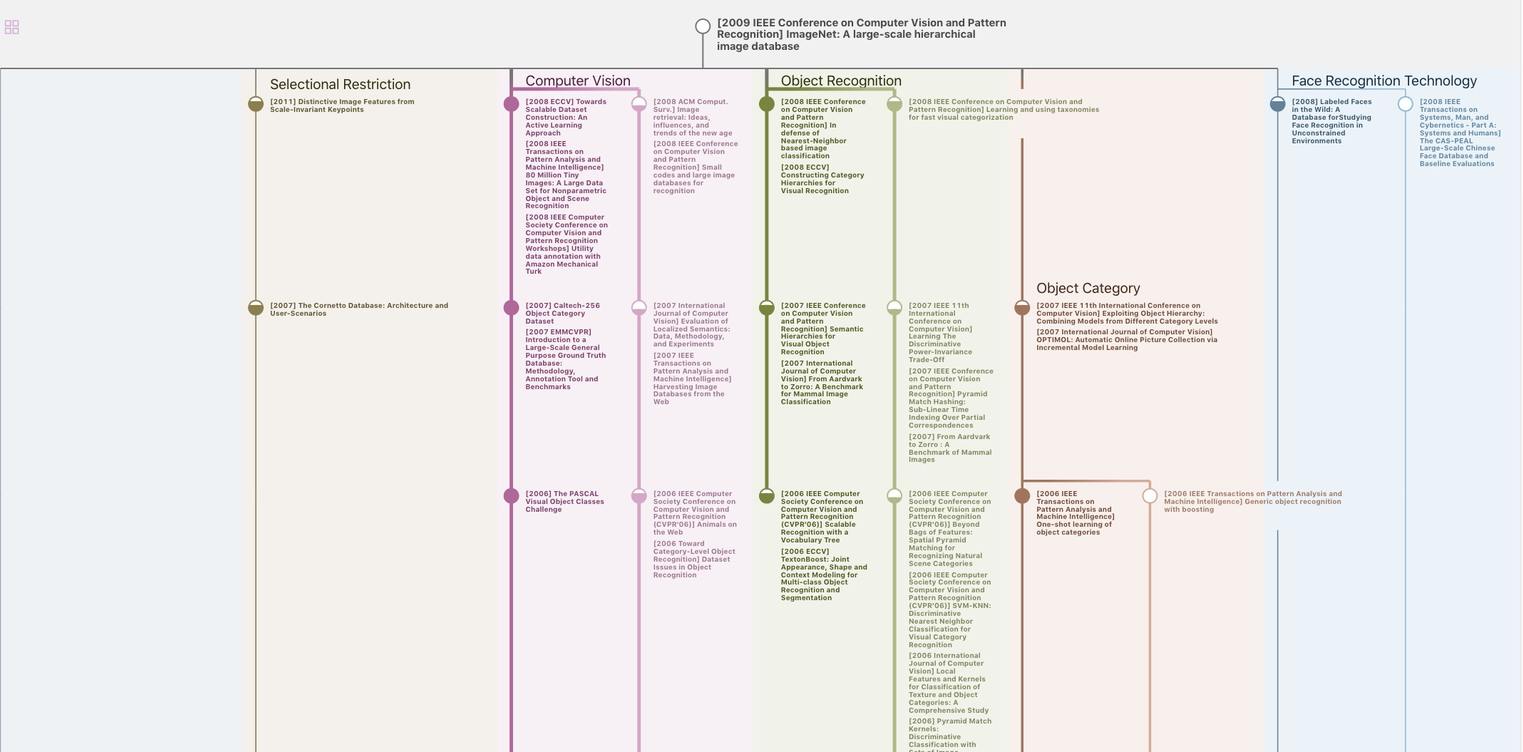
生成溯源树,研究论文发展脉络
Chat Paper
正在生成论文摘要