Automated condition-invariable neurite segmentation and synapse classification using textural analysis-based machine-learning algorithms.
Journal of Neuroscience Methods(2013)
摘要
High-resolution live-cell imaging studies of neuronal structure and function are characterized by large variability in image acquisition conditions due to background and sample variations as well as low signal-to-noise ratio. The lack of automated image analysis tools that can be generalized for varying image acquisition conditions represents one of the main challenges in the field of biomedical image analysis. Specifically, segmentation of the axonal/dendritic arborizations in brightfield or fluorescence imaging studies is extremely labor-intensive and still performed mostly manually. Here we describe a fully automated machine-learning approach based on textural analysis algorithms for segmenting neuronal arborizations in high-resolution brightfield images of live cultured neurons. We compare performance of our algorithm to manual segmentation and show that it combines 90% accuracy, with similarly high levels of specificity and sensitivity. Moreover, the algorithm maintains high performance levels under a wide range of image acquisition conditions indicating that it is largely condition-invariable. We further describe an application of this algorithm to fully automated synapse localization and classification in fluorescence imaging studies based on synaptic activity. Textural analysis-based machine-learning approach thus offers a high performance condition-invariable tool for automated neurite segmentation.
更多查看译文
关键词
Neurite,Synapse,Imaging, Segmentation,Machine learning,Textural analysis
AI 理解论文
溯源树
样例
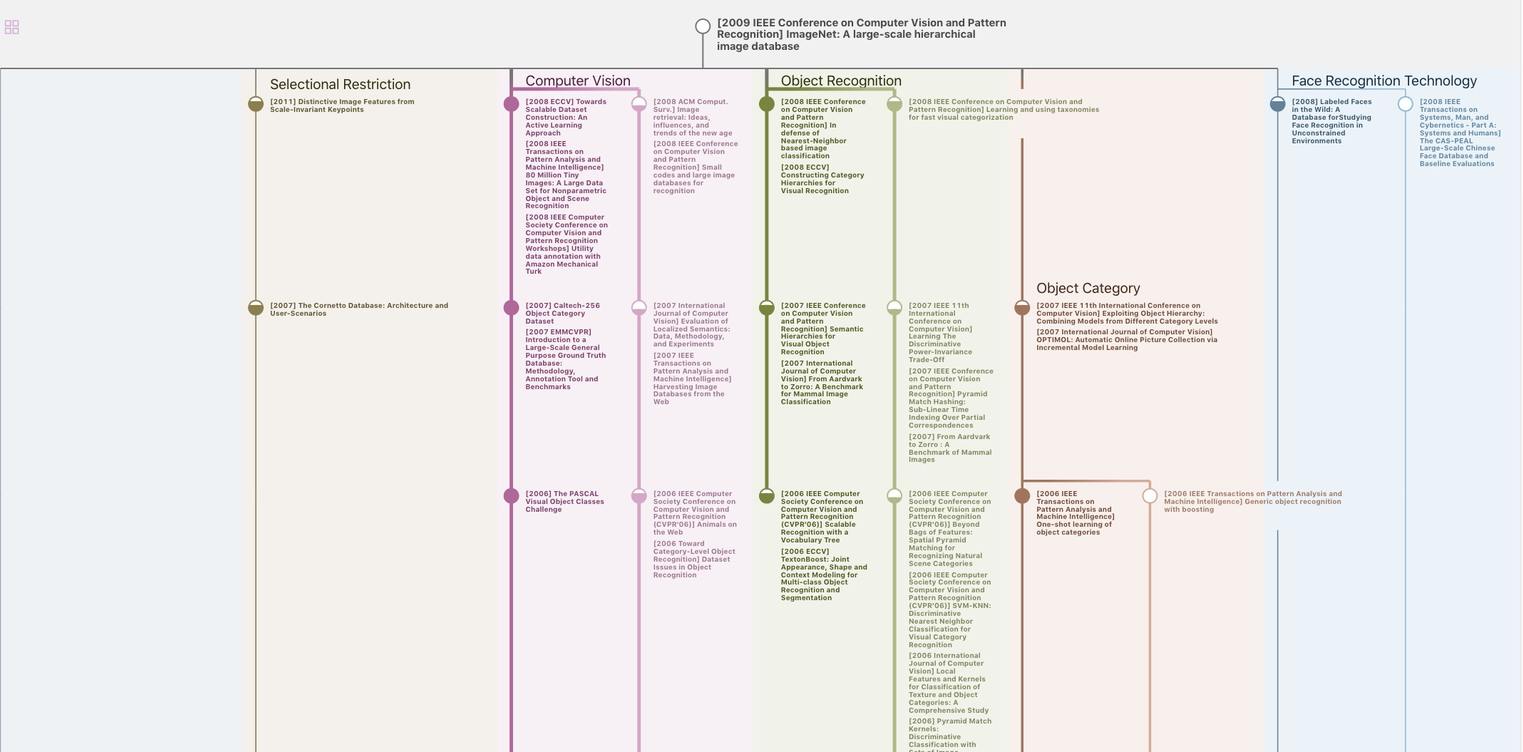
生成溯源树,研究论文发展脉络
Chat Paper
正在生成论文摘要