A Seminonparametric Approach to Joint Modeling of A Primary Binary Outcome and Longitudinal Data Measured at Discrete Informative Times.
Statistics in biosciences(2012)
摘要
In a study conducted at the New York University Fertility Center, one of the scientific objectives is to investigate the relationship between the final pregnancy outcomes of participants receiving an in vitro fertilization (IVF) treatment and their β-human chorionic gonadotrophin (β-hCG) profiles. A common joint modeling approach to this objective is to use subject-specific normal random effects in a linear mixed model for longitudinal β-hCG data as predictors in a model (e.g., logistic model) for the final pregnancy outcome. Empirical data exploration indicates that the observation times for longitudinal β-hCG data may be informative and the distribution of random effects for longitudinal β-hCG data may not be normally distributed. We propose to introduce a third model in the joint model for the informative β-hCG observation times, and relax the normality distributional assumption of random effects using the semi-nonparametric (SNP) approach of Gallant and Nychka (1987) [8]. An EM algorithm is developed for parameter estimation. Extensive simulation designed to evaluate the proposed method indicates that ignoring either informative observation times or distributional assumption of the random effects would lead to invalid and/or inefficient inference. Applying our new approach to the data reveals some interesting findings the traditional approach failed to discover.
更多查看译文
AI 理解论文
溯源树
样例
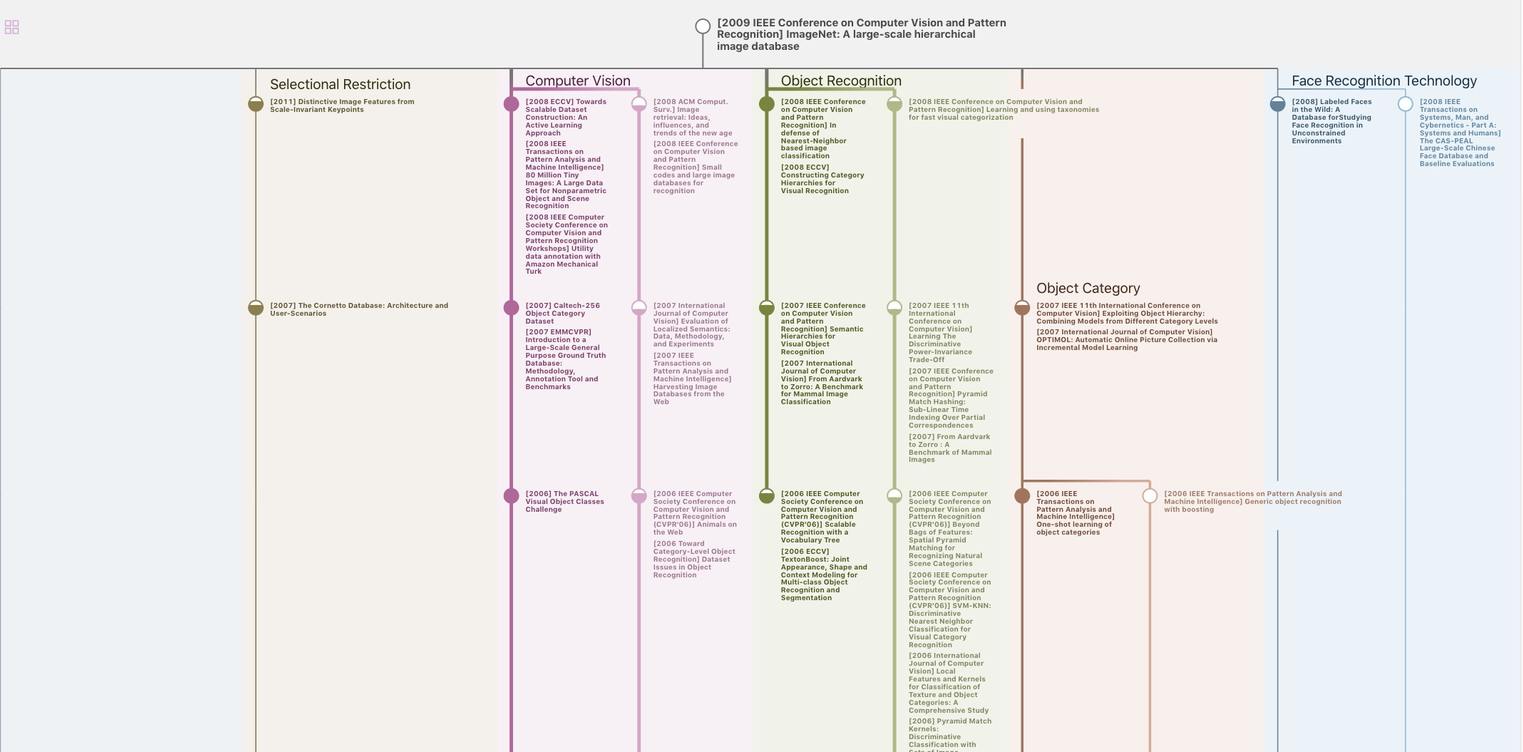
生成溯源树,研究论文发展脉络
Chat Paper
正在生成论文摘要