Application of copulas to improve covariance estimation for partial least squares.
STATISTICS IN MEDICINE(2013)
摘要
Dimension reduction techniques, such as partial least squares, are useful for computing summary measures and examining relationships in complex settings. Partial least squares requires an estimate of the covariance matrix as a first step in the analysis, making this estimate critical to the results. In addition, the covariance matrix also forms the basis for other techniques in multivariate analysis, such as principal component analysis and independent component analysis. This paper has been motivated by an example from an imaging study in Alzheimer's disease where there is complete separation between Alzheimer's and control subjects for one of the imaging modalities. This separation occurs in one block of variables and does not occur with the second block of variables resulting in inaccurate estimates of the covariance. We propose the use of a copula to obtain estimates of the covariance in this setting, where one set of variables comes from a mixture distribution. Simulation studies show that the proposed estimator is an improvement over the standard estimators of covariance. We illustrate the methods from the motivating example from a study in the area of Alzheimer's disease. Copyright (C) 2012 John Wiley & Sons, Ltd.
更多查看译文
关键词
copula,covariance,correlation,complete separation,neuroimaging,partial least squares
AI 理解论文
溯源树
样例
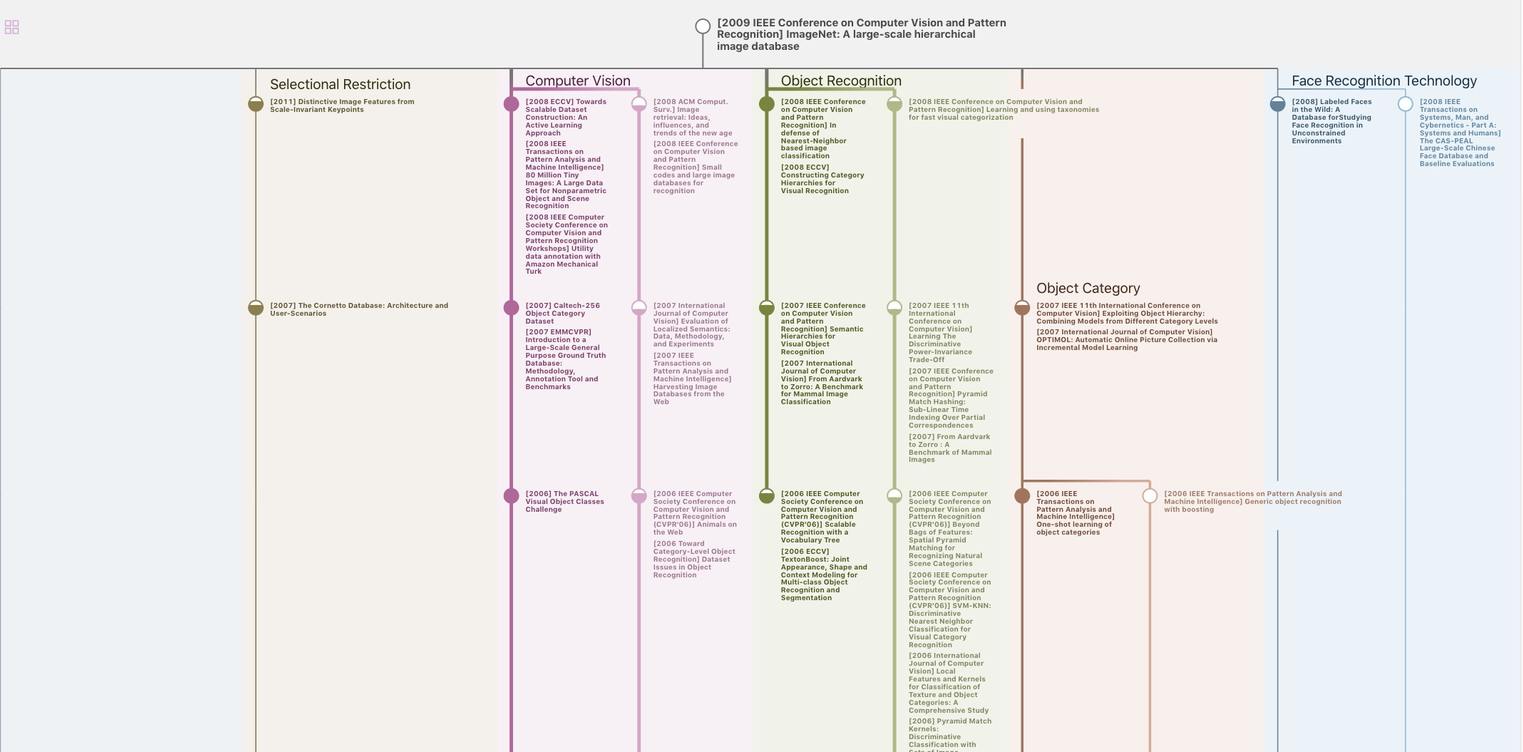
生成溯源树,研究论文发展脉络
Chat Paper
正在生成论文摘要