Video-SwinUNet: Spatio-temporal Deep Learning Framework for VFSS Instance Segmentation
2023 IEEE INTERNATIONAL CONFERENCE ON IMAGE PROCESSING, ICIP(2023)
摘要
This paper presents a deep learning framework for medical video segmentation. Convolution neural network (CNN) and transformer-based methods have achieved great milestones in medical image segmentation tasks due to their incredible semantic feature encoding and global information comprehension abilities. However, most existing approaches ignore a salient aspect of medical video data - the temporal dimension. Our proposed framework explicitly extracts features from neighbouring frames across the temporal dimension and incorporates them with a temporal feature blender, which then tokenises the high-level spatio-temporal feature to form a strong global feature encoded via a Swin Transformer. The final segmentation results are produced via a UNet-like encoder-decoder architecture. Our model outperforms other approaches by a significant margin and improves the segmentation benchmarks on the VFSS2022 dataset, achieving a dice coefficient of 0.8986 and 0.8186 for the two datasets tested. Our studies also show the efficacy of the temporal feature blending scheme and cross-dataset transferability of learned capabilities. Code and models are available at https://github.com/SimonZeng7108/Video-SwinUNet.
更多查看译文
关键词
Deep Learning,Swin Transformer,Video Tracking,Dysphagia,Videofluoroscopy
AI 理解论文
溯源树
样例
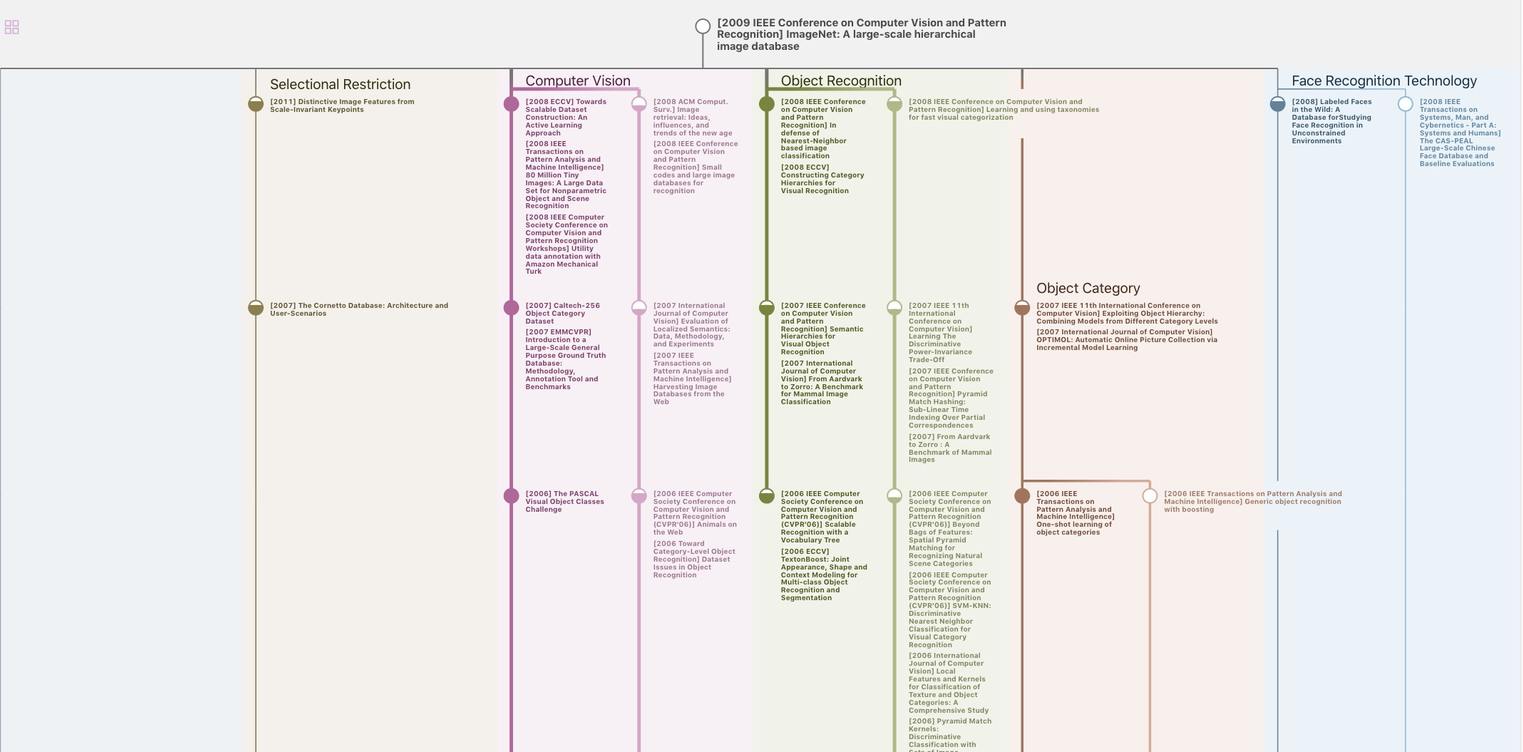
生成溯源树,研究论文发展脉络
Chat Paper
正在生成论文摘要