New similarity search based glioma grading
NEURORADIOLOGY(2011)
摘要
Introduction MR-based differentiation between low- and high-grade gliomas is predominately based on contrast-enhanced T1-weighted images (CE-T1w). However, functional MR sequences as perfusion- and diffusion-weighted sequences can provide additional information on tumor grade. Here, we tested the potential of a recently developed similarity search based method that integrates information of CE-T1w and perfusion maps for non-invasive MR-based glioma grading. Methods We prospectively included 37 untreated glioma patients (23 grade I/II, 14 grade III gliomas), in whom 3T MRI with FLAIR, pre- and post-contrast T1-weighted, and perfusion sequences was performed. Cerebral blood volume, cerebral blood flow, and mean transit time maps as well as CE-T1w images were used as input for the similarity search. Data sets were preprocessed and converted to four-dimensional Gaussian Mixture Models that considered correlations between the different MR sequences. For each patient, a so-called tumor feature vector (= probability-based classifier) was defined and used for grading. Biopsy was used as gold standard, and similarity based grading was compared to grading solely based on CE-T1w. Results Accuracy, sensitivity, and specificity of pure CE-T1w based glioma grading were 64.9%, 78.6%, and 56.5%, respectively. Similarity search based tumor grading allowed differentiation between low-grade (I or II) and high-grade (III) gliomas with an accuracy, sensitivity, and specificity of 83.8%, 78.6%, and 87.0%. Conclusion Our findings indicate that integration of perfusion parameters and CE-T1w information in a semi-automatic similarity search based analysis improves the potential of MR-based glioma grading compared to CE-T1w data alone.
更多查看译文
关键词
Glioma grading,Perfusion MRI,Similarity search,Gaussian Mixture Model
AI 理解论文
溯源树
样例
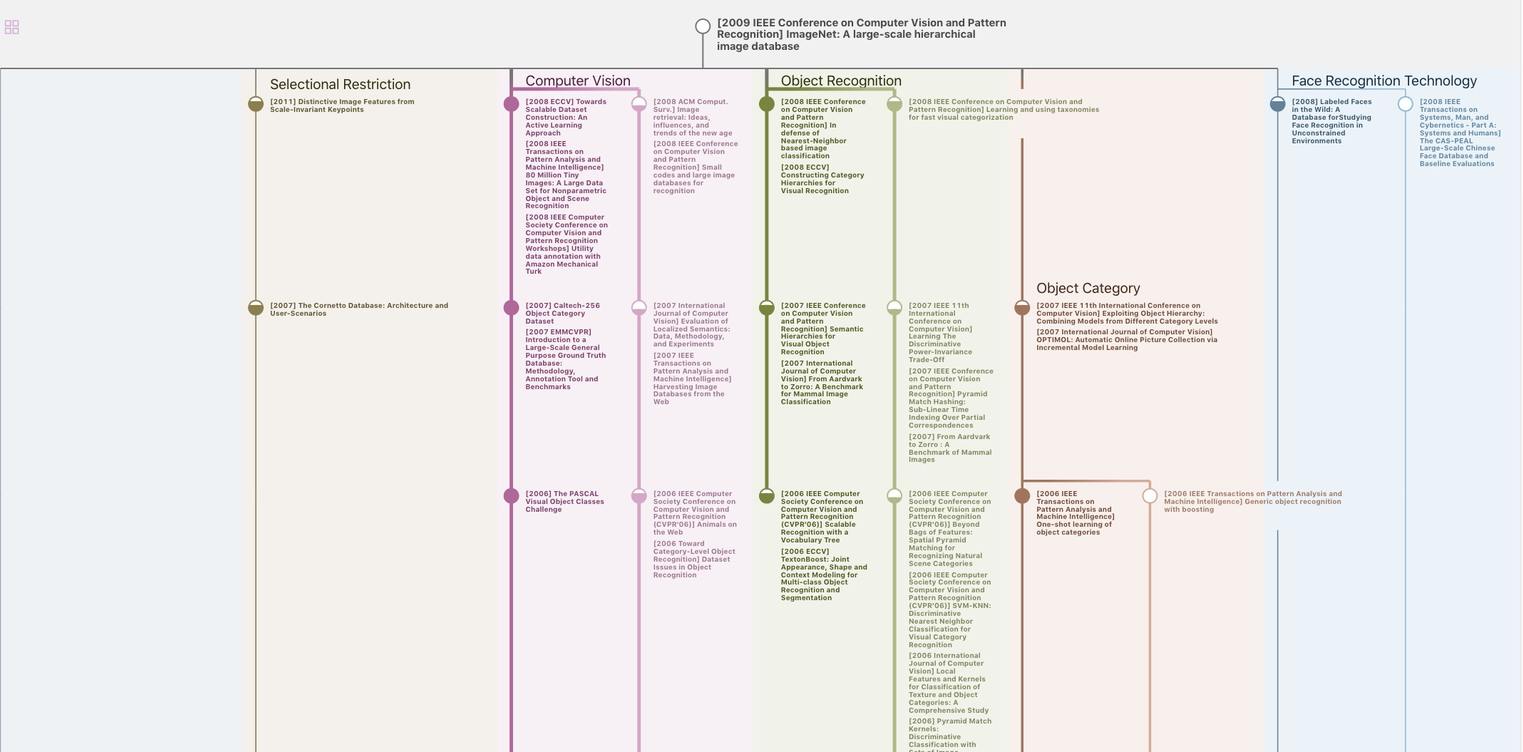
生成溯源树,研究论文发展脉络
Chat Paper
正在生成论文摘要