Explainable Automated Recognition of Emotional States from Canine Facial Expressions: The Case of Positive Anticipation and Frustration
crossref(2022)
摘要
Abstract Internal affective states are closely linked to facial expressions in both human and many non-human animals. For some animal species, objective tools for facial expression analysis such as AnimalFACS are available and are only just beginning to be increasingly used. However, their use requires special expertise, training, and certification, while there is still some remaining risk for human bias, and its application is time-consuming. Automation of facial analysis offers a promising alternative and is already being addressed by a large body of research in the human domain. In animal research, automation has so far been addressed for a few species in the context of pain, while emotional state recognition remains underexplored, especially in canine species due to the complexity of their facial morphology and expressions. The contribution of the present study is twofold. First, this is the first study to address automated recognition of emotional states in dogs using a dataset obtained in a controlled experimental setting, including videos from (n=29) Labrador Retrievers assumed to be in two experimentally induced emotional states: negative (frustration) and positive (anticipation). Two different approaches are compared in relation to our aim: (1) A DogFACS-based approach with a two-step pipeline consisting of (i) an Action Unit (AU) detector and (ii) a positive/negative state Decision Tree classifier; (2) An approach using deep learning techniques with no intermediate representation. The approaches reach accuracy of above 71% and 89%, respectively, with the deep learning approach performing better. Secondly, this study is the first to address the explainability of AI models in the context of understanding the expression of emotion in animals. The DogFACS-based approach provides decision trees, that is a mathematical representation which reflects previous findings by human experts in relation to certain DogFACS variables being correlates of specific emotional states. The deep learning approach offers a different, visual form of explainability in the form of heatmaps reflecting regions of focus of the network's attention, which in some cases show focus clearly related to the nature of particular AUs. These heatmaps may hold the key to novel insights on the sensitivity of the network to nuanced pixel patterns reflecting information invisible to the human eye.
更多查看译文
AI 理解论文
溯源树
样例
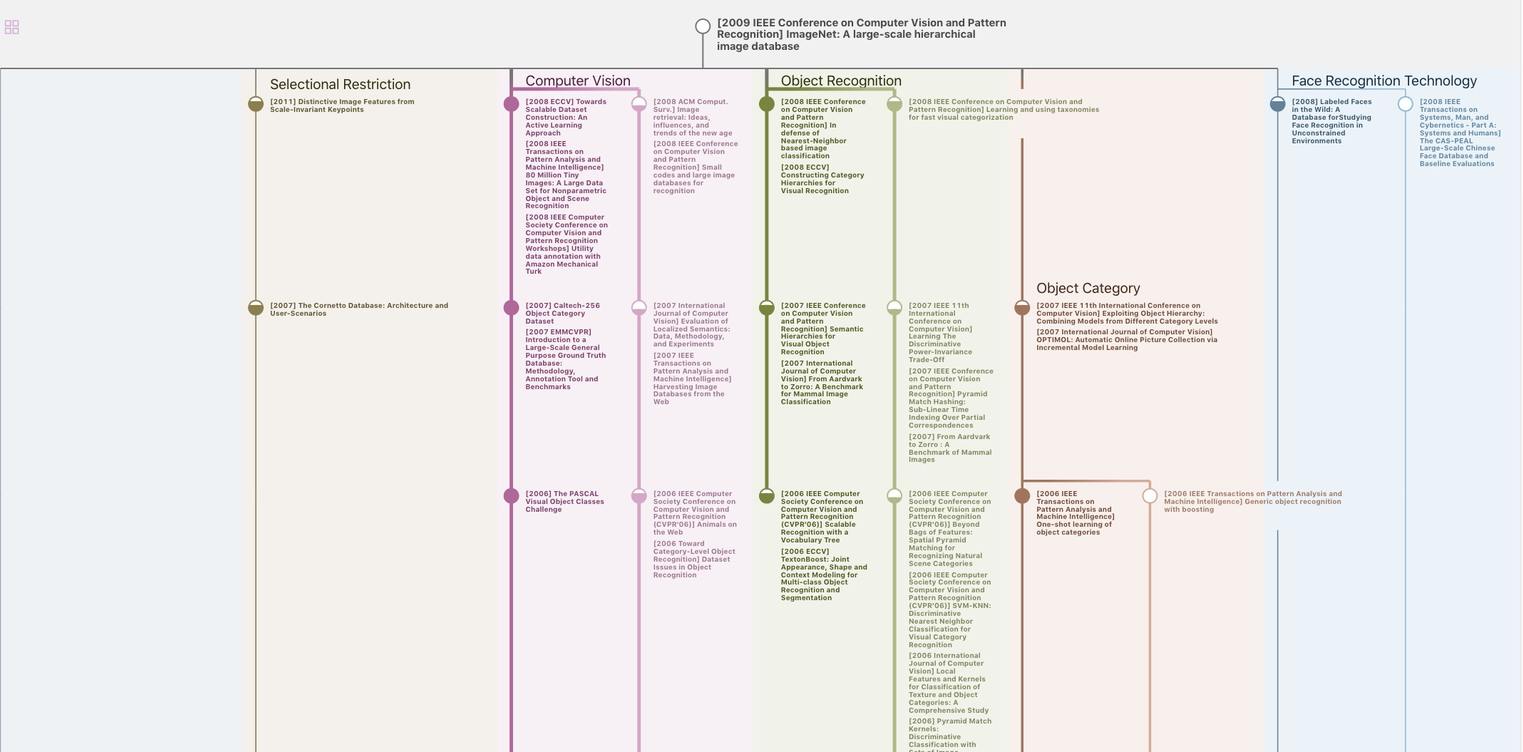
生成溯源树,研究论文发展脉络
Chat Paper
正在生成论文摘要