Learning To Detect And Retrieve Objects From Unlabeled Videos
2019 IEEE/CVF INTERNATIONAL CONFERENCE ON COMPUTER VISION WORKSHOPS (ICCVW)(2019)
摘要
Learning an object detection or retrieval system requires a large data set with manual annotations. Such data sets are expensive and time consuming to create and therefore difficult to obtain on a large scale. In this work, we propose to exploit the natural correlation in narrations and the visual presence of objects in video, to learn an object detector and retrieval without any manual labeling involved. We pose the problem as weakly supervised learning with noisy labels, and propose a novel object detection paradigm under these constraints. We handle the background rejection by using contrastive samples and confront the high level of label noise with a new clustering score. Our evaluation is based on a set of 11 manually annotated objects in over 5000 frames. We show comparison to a weakly-supervised approach as baseline and provide a strongly labeled upper bound.
更多查看译文
关键词
unlabeled videos,data set,manual annotations,natural correlation,object detector,manual labeling,weakly supervised learning,noisy labels,object detection,label noise,object retrieval,clustering score,manually annotated objects
AI 理解论文
溯源树
样例
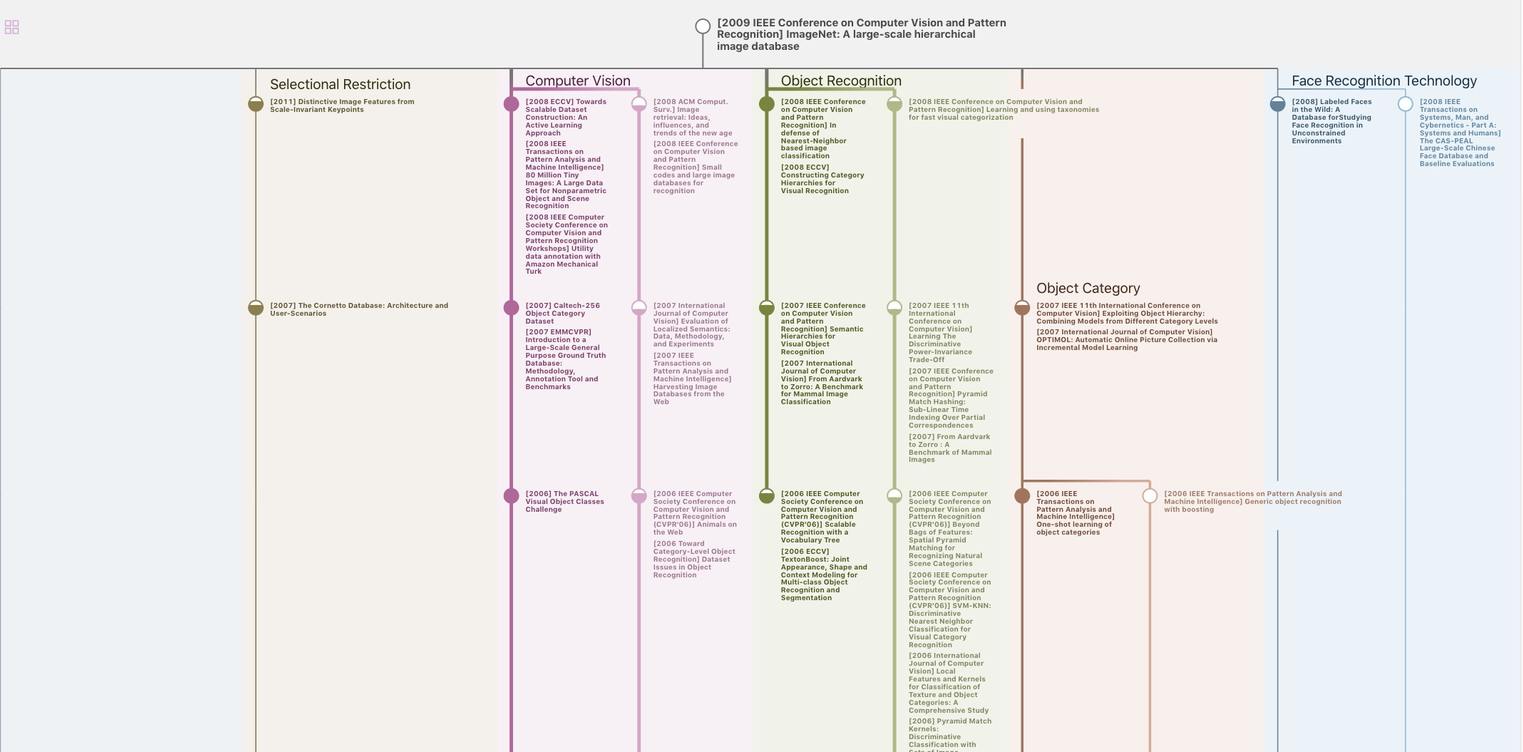
生成溯源树,研究论文发展脉络
Chat Paper
正在生成论文摘要